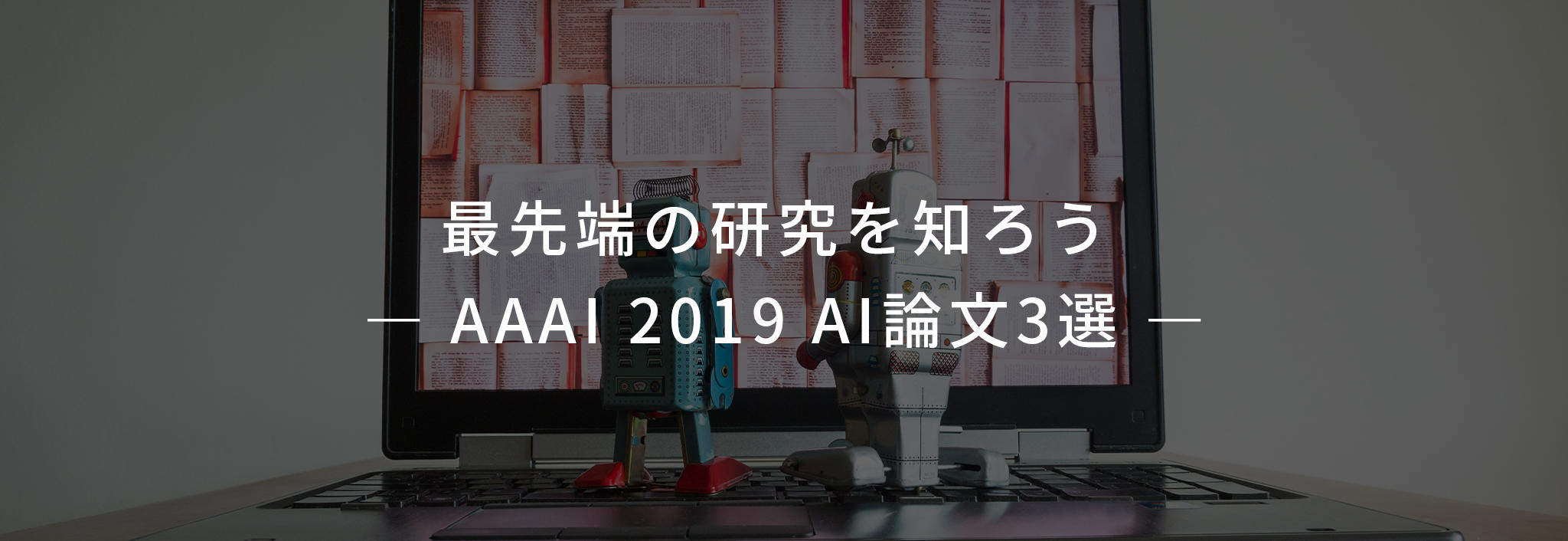
This article is recommended for those who
- Those who want to know what kind of papers are accepted for AAAI 2019
- Those who do not have time to read papers but want to know about recent AI research
- Those who want to know about AAAI 2019
Time needed to finish reading this article
10分未満
AAAI 2019 papers will be picked up and introduced
Hello, I'm Makky from Macnica AI Women's Club! Summer is getting longer every year, and it looks like there will be more hot days.
However, the season will shift to autumn and it will gradually become colder.
As the seasons are changing, please take care of your health!
Now let's get to the point. The theme of this blog is “AAAI 2019 Adopted Papers”.
In the tech blog the other day, I introduced the papers accepted for CVPR 2019, and I would like to introduce the papers accepted for AAAI 2019 following CVPR.
Recent AI trends can be seen from the AAAI 2019 paper
AAAI is a world-class international conference on artificial intelligence held from January to February every year.
Therefore, by reading papers accepted by AAAI, you can learn about the latest trends in artificial intelligence in general.
This time, we will introduce three selected papers from China, where AI research has been active in recent years, among the accepted papers of AAAI 2019 held from January 27 to February 1, 2019.
- “Joint Representation Learning for Multi-Modal Transportation Recommendation” Transportation Recommendation Method Using Multi-Modal Learning
- High Performance Face Recognition Framework “Selective Refinement Network for High Performance Face Detection”
- “Deep Interest Evolution Network for Click-Through Rate Prediction” CTR prediction architecture whose performance has already been evaluated in actual operation
1. “Joint Representation Learning for Multi-Modal Transportation Recommendation”
The first article to be introduced, "Joint Representation Learning for Multi-Modal Transportation Recommendation," is a paper that proposes a transportation recommendation system based on multi-modal learning and builds its framework.
Multimodal learning is one of the trends in deep learning and has been the subject of a lot of research recently.
Multimodal refers to the use of multiple modalities (senses), and multimodal learning in machine learning is a learning method that learns from multiple types of data and processes them in an integrated manner.
Now, regarding the transportation recommendation system that appears in the paper I introduce, conventionally, the focus is only on one modality, and various modes of transportation such as walking, cycling, automobiles, public transportation, etc., and combinations of these modes. I was recommending transportation to users.
However, if the user's preferences (cost, time, etc.) and travel characteristics (travel purpose, travel distance, etc.) are taken into account when recommending transportation, it is believed that better recommendations can be made that are more tailored to the user.
Therefore, in this paper, we propose a learning framework, Trans2Vec, that enables traffic recommendations by multimodal learning. The figure below is a schematic diagram of the Trans2Vec framework.
具体的には、地図データベース、ユーザーの人口統計属性、および出発点-到着点情報を含むデータに基づいて、下図のようなイメージで、複数のモダリティに焦点を当てたマルチモーダル交通グラフを作成します。
この交通グラフを用いて交通手段の学習をすることで、ユーザーと目的地の履歴から適宜最適な交通手段を取得できるようになっています。
論文中では複数の推薦システムの評価指標を用いて、既存のアルゴリズムとTrans2Vecの性能比較もしています。その結果が以下の表になります。
北京と上海の交通機関推奨をした結果、PREC(Precision=適合率)以外の3つの指標で、Trans2Vecは良い値を出しています。
PRECにおいても値としてはさほど悪くない、むしろ1番に良い値とあまり変わらない値となっています。
Trans2Vec has already been introduced into a production system and is likely to become a framework that will continue to play an active role in the future.
2. “Selective Refinement Network for High Performance Face Detection”
Next, I will introduce a paper on the theme of "face recognition".
Recently, major Japanese companies are actively developing systems for facial recognition cashless payments, and we often see updates on system development in the news.
Of course, face recognition can be used for purposes other than cashless payments, so research into face recognition is being enthusiastically conducted not only in Japan but in many countries.
So the second is a research paper "Selective Refinement Network for High Performance Face Detection" from China, where face recognition research is very active.
The task of face recognition has been actively researched for a long time, but face recognition for images with many small faces, such as photographs of crowds, has been a difficult task because it cannot be said to have high performance. .
There are two issues with conventional face recognition tasks, the first of which is the recall rate.
Even SOTA's ReitiaNet, which was proposed in 2017, had a precision (precision) of 90% and a recall of 50%, which is good precision but not very good recall.
The second issue is that of position recognition accuracy. The smaller the face, the more difficult it is to locate the face.
今回ご紹介するこの論文では、新しい顔検出用フレームワークである Selective Refinement Network(SRN) を提唱しています。
SRNは選択的2段階分類(STC)と選択的2段階回帰(STR)という2つの主要なモジュールで構成され、この2つのモジュールによって偽陽性を減らすと同時に位置精度を改善しました。
Below is a comparison of the accuracy of face recognition using face recognition datasets such as AFW, PASCAL face, and FDDB.
You can see that Ours (that is, SRN) indicated by the red line is highly accurate in any graph.
そして、下図はWIDER FACEと言う顔認識用データセットを使用した顔認識の精度比較です。
こちらも同様に、どのデータセットケースにおいてもSRNが高精度であることがわかります。
顔認証は普段の生活でも身近な分野であるからこそ、今後さらに発展し、現在多くの企業で開発・研究中の顔認証キャッシュレス決済もクオリティの高いものになっていくかもしれません。
3. “Deep Interest Evolution Network for Click-Through Rate Prediction”
Finally, I would like to introduce the technology that is already active in the marketing field, which I have recently been interested in.
Marketing terms include LTV (the total amount of profit that one customer brings to a company in a lifetime: Life Time Value) and CPA (a numerical value that expresses how much advertising costs are spent for one result: Cost Per Action). Yes, but the relationship between these numbers and advertising is very close.
スマートデバイスの普及により、今や広告は身近すぎる存在ではありますが、ここ2年ほど市場規模ではWeb広告の方がテレビ広告を上回っています。
Therefore, in the third paper, I would like to introduce research on predicting the CTR (Click Through Rate) of the web advertising industry.
DIN (Deep Interest Network) has been devised as a method for CTR prediction.
This DIN directly considered relative interest in target items to be related to user behavior.
However, users' latent interests may not be fully reflected in their explicit behavior.
Also, since user interests are constantly changing and evolving, it is very important to capture trends in interest.
In order to model the process of this interest evolution, a new network architectureDIEN is proposed in this paper.
Below is DIEN's network structure diagram.
DIENのネットワーク構造においてDINと異なる特徴が2点あります。
1つめは、ふるまいを直接興味として扱うのではなく、ユーザーの興味/関心を抽出するレイヤー(Interest Extractor Layer)を追加している点です。
2つめは、ユーザーの関心が進化するプロセスをモデル化した、興味を発展させるレイヤー(Interest Evolving Layer)が追加された点です。
With the addition of these two layers, we are now able to use the underlying interests of our users as well for CTR prediction.
DIEN has already advanced to the actual operation phase, and was introduced to Taobao's display advertising system in China, and reportedly achieved a 20.7% improvement in CTR.
Summary
今回はAAAI 2019採択論文をご紹介いたしました。
マルチモーダル学習や顔認証などは近年とても人気の分野です。
今後も実運用フェーズで活躍できそうな研究成果が多く発表されることでしょう。
We will continue to pick up and introduce summaries of conference papers and interesting AI papers on the women's division tech blog, so please look forward to the next time!
■ Sources ofAI papers featured in this article / Reference Lists |