What are the hurdles to introduce an image AI processing PC?
Image AI usually involves a large amount of data and calculations, and requires a high-spec PC with a GPU. However, the user burden in terms of size and price has been an issue for this level of spec PC. There is also the option of processing on the cloud, but due to the large amount of data, network load and cloud usage fees are also issues.
Therefore, I would like to verify the image AI processing of Axiomtek's RSC101 as one of the solutions to the above-mentioned introduction hurdles. RSC101 is equipped with Hailo-8™ as an AI accelerator, and is a product that aims to improve performance by entrusting AI processing.
About Axiomtek RSC101
The RSC101 is a small, fanless, easy-to-use BOX PC that uses an Intel® Elkhart Lake processor as the CPU. Hailo-8™ is adopted as the AI accelerator, realizing AI processing performance of 26 TOPS, and there are many options for wireless communication, so I think it is an easy-to-use BOX PC.
Below are the main specs.
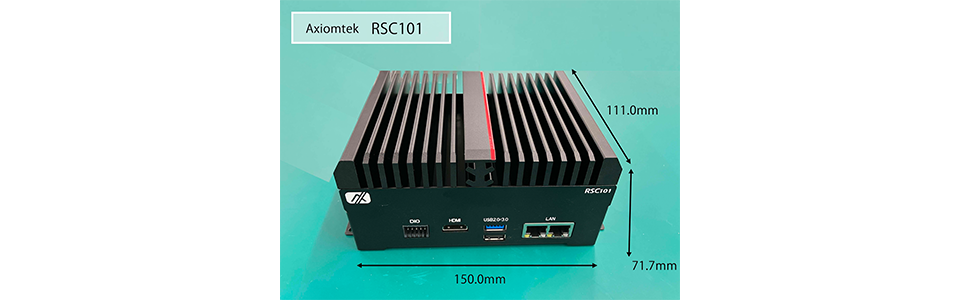
AI Accelerator | Hailo-8™ SoC, up to 26 TOPS |
CPU | Intel® Celeron® Processor J6413 |
System memory | 1 x DDR4 SO-DIMM slot, up to 32GB |
Storage | 1 x M.2 Key B 2242 SSD slot (SATA3) |
USB |
1 x USB 3.2 Gen1 1 x USB 2.0 |
Ethernet | 2 x 10/100/1000 Mbps Ethernet |
Display | 1 x HDMI 1.4 with 4K2K supported |
Digital I/O | 1 x 8-CH DI/DO |
Expansion |
1 x M.2 Key E 2230 for Wi-Fi/Bluetooth 1 x M.2 Key B 3052 for 5G 1 x SIM slot |
Regarding the size, there are two types depending on the size of the heat sink, and they are as follows.
・150.0mm x 111.0mm x 71.7mm (-10℃ to +70℃ compatible) ←Photo is here
・150.0mm x 111.0mm x 49.4mm (-10℃ to +60℃ compatible)
Image AI performance verification of RSC101
The OS is Ubuntu22.04, and the Tappas tool and PCIe driver provided by Hailo are installed. Tappas is a demo application tool that includes a series of functions from image capture to AI processing and display. First of all, I would like to introduce the status of the PCIe connection with Hailo-8™. See the results of the lspci command below.
As mentioned above, PCIe is connected with Gen3 x 4lane, which is the upper limit of Hailo-8™. I think that there are still many inexpensive embedded PCs that can only connect with Gen2, and even with 1 or 2 lanes.
The actual transfer rate of Gen2 and Gen3 is as follows, and there is a difference of about 2 times.
・Real transfer rate of PCIe Gen2 --- 4Gbits/s (5Gbits/s x 80%)
・ Actual transfer rate of PCIe Gen3 --- 7.877 Gbits / s (8 Gbits / s × 98.462%)
Furthermore, if there is a difference between 1lane and 4lane, the total difference in transfer rate will be about 8 times. Hailo-8™ loads and runs AI network models over PCIe, so PCIe bandwidth is an important factor, especially for large models. With RSC101, PCIe connection is possible with the maximum value of Hailo-8™ specifications, so high performance can be expected.
Now let's check the actual performance.
Verification ①: Object detection (Yolov5m, Yolov3)
First, let's try Yolov5m object detection with Tappas. Check the screen below for the result.
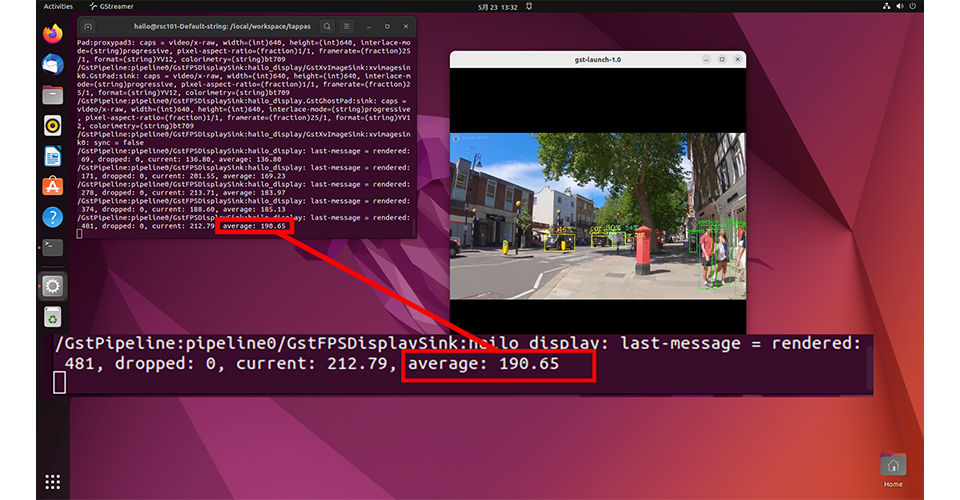
In the above, fps is displayed on the terminal, but I think you can see the operation at 180fps or more. It is a calculation that can infer six 30fps cameras with a simple calculation, so it is quite an AI performance.
Next is the execution result with Yolov3. Yolov3 has a relatively large network size, and Hailo-8™ divides the model into multiple models and executes inference processing while switching the contents on the time axis. Content switching is performed via PCIe, so PCIe bandwidth affects performance.
Looking at the results below, it can be operated at 45fps or higher. If the PCIe band is narrow, there is a concern that the fps will drop more than this.
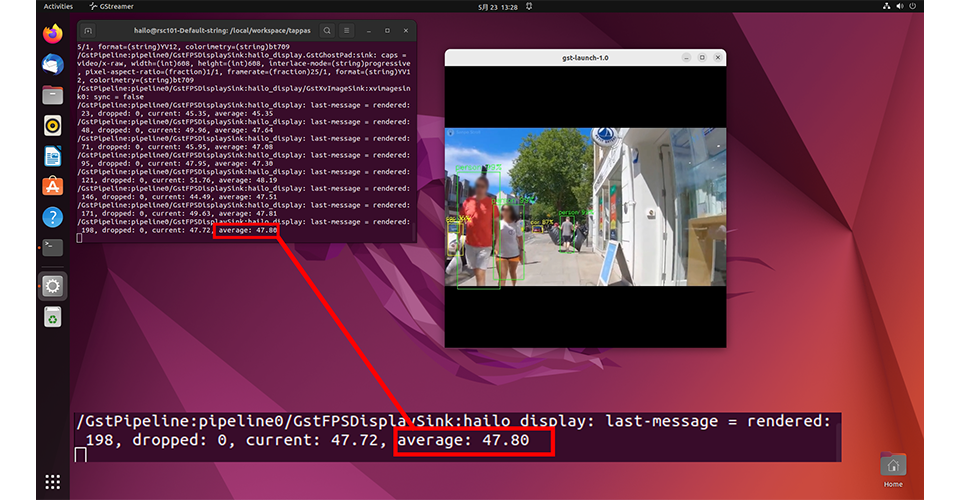
Verification (2): Segmentation with Full-HD images
At the beginning, I mentioned that the image AI process is heavy, but the higher the screen resolution, the more difficult the processing becomes. On the camera side, full-HD and 4K resolutions are becoming commonplace, but in image AI, many network models support VGA-class resolutions.
Tappas has a full-HD segmentation demo, so let's run it (the model used is fcn8_resnet_v1_18). The result is as follows, but even Full-HD can be moved at 20 fps or more, so I think it can be said that it is a practical level.
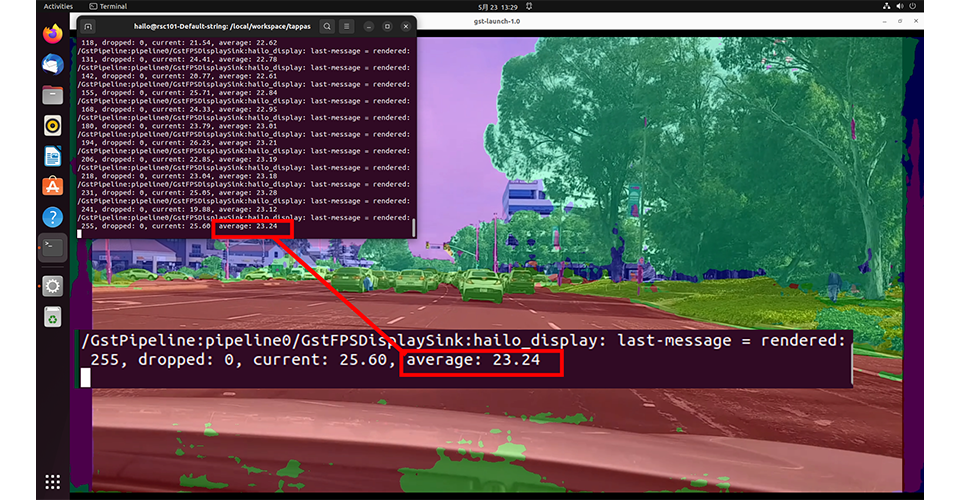
Verification (3): Switching behavior of multiple network models
When creating an actual application that incorporates image AI, there are many cases where you want to run multiple network models, such as detecting a car and then reading the license plate. The image below is a verification of running while switching multiple networks (yolov5s and fast_depth). Network switching is done via PCIe, so the bandwidth there is important.
All of them can be operated at 100 fps or more, and you can see that high performance is achieved while switching networks.
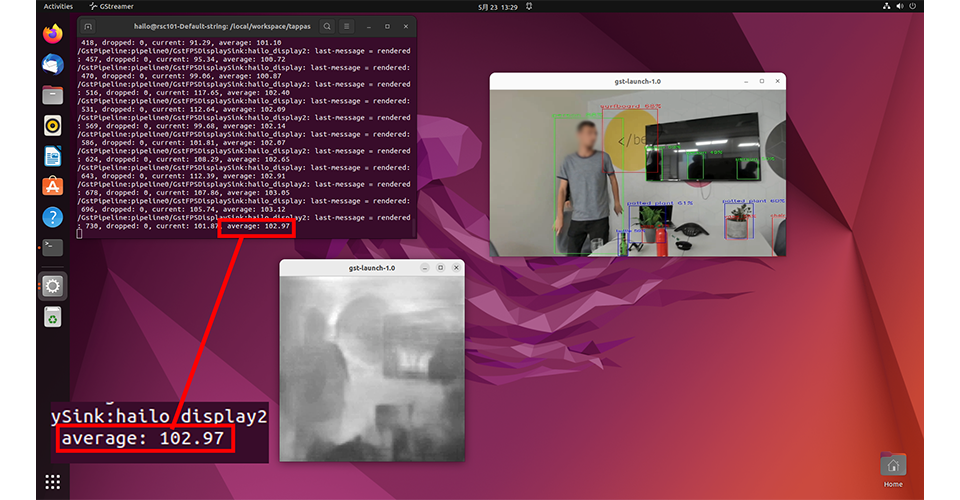
Verification ④: Human detection and tracking (ReID)
Finally, I will introduce the person detection & tracking demo (ReID). Conventionally, image AI was applied to a video as a single still image and the result was displayed.
In this demonstration, the detected person is marked and tracked. In addition, you can track across multiple cameras (the same number appears in the bottom left of Box when the same person appears in different cameras). In previous demos, the accelerator Hailo-8™ and the data transfer part (PCIe) were the major factors that determined the performance, but this time there is also some processing on the CPU side, so the performance of the entire system is shown. .
It's hard to understand on the screen, not the video, but I'll paste the image for reference. (In the video, tracking is applied numerically.)
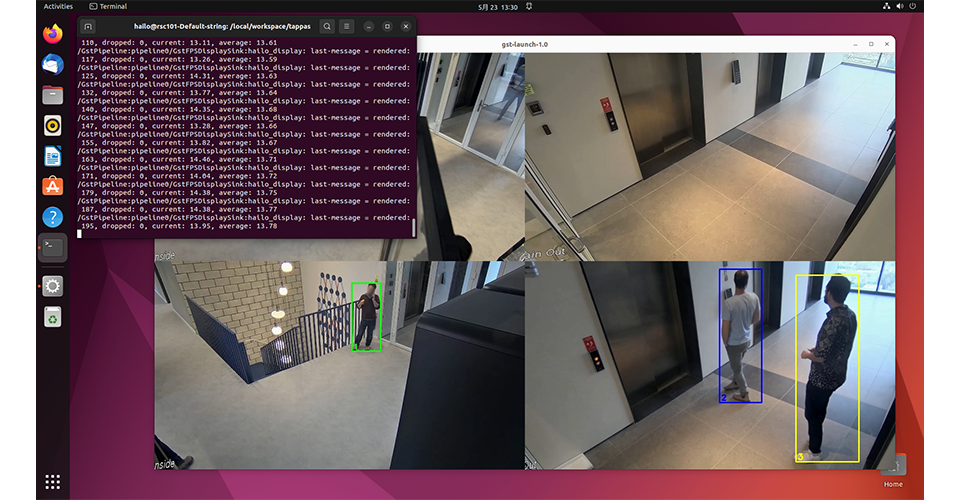
Summary
I felt that although the RSC101 equipped with Hailo-8™ is small, its image AI performance is noteworthy.
Inquiry
If you would like to know more about prices, etc., please contact us using the inquiry form below.
Hailo manufacturer information Top
If you want to return to Hailo manufacturer information top page, please click below.