Efficiency of housing “drawing reading work”
- AI calculates various coordinate values from PDF housing drawings
- Reduced dependency on drawing reading work and improved work efficiency
- Construction/real estate
- drawing reading
- Improve operational efficiency
- Work time reduction
- pick up
- CrowdANALYTIX
Business challenge
In the construction industry, when conducting survey work, it is necessary to correctly interpret different drawings due to various construction methods, identify the length and height of each side of each room, and quantify the standards for openings.
This drawing reading work is the first step in all cost estimation work, but it required the expertise of a skilled person.
From the perspective of future increase in the number of buildings and business continuity, it was necessary to consider more efficient business operations using AI that does not rely on human resources such as BPO.
Task
In the building materials wholesale industry, it is necessary to correctly interpret the information on the drawings sent from building contractors, and to estimate materials such as wallpaper and tiles. As the business expanded, the number of buildings to be estimated increased, and it was necessary to improve the efficiency of the work of reading drawings, which is the first step in cost estimation.
A housing drawing contains a large amount of information necessary for construction, but the drawing method differs depending on the construction method. There are various scales, such as 909mm, 910mm, and 1000mm, that express the scale of the drawings. In addition, depending on the creator and the building contractor, the way of notation of walls, pillars, internal and external openings is different, and in order to interpret and estimate it, it is necessary for experienced workers to read the drawings. In order for an inexperienced person to acquire the skill to read and understand drawings to some extent, it is necessary to refine the work accuracy while accumulating experience after receiving at least one month of training.
Moreover, even an educated and experienced worker cannot easily read a drawing, and it is a time-consuming task. Even for a skilled worker, it takes about an hour to read a single house drawing.
Since manual work by the person in charge is essential for the work, the upper limit of the number of drawings that the person in charge can process becomes the upper limit of the number of buildings that can be accumulated. On the other hand, it is not easy to increase the number of people in charge, and it takes time to train them. Until now, we have increased the number of workers while also using overseas BPO, but it is clear that this part will become a bottleneck in the future in expanding the parts wholesale business. In addition, drawing data will be an important non-financial asset in future DX. There was an urgent need to use technology to streamline and automate the drawing reading work, which had become dependent on individual skills.
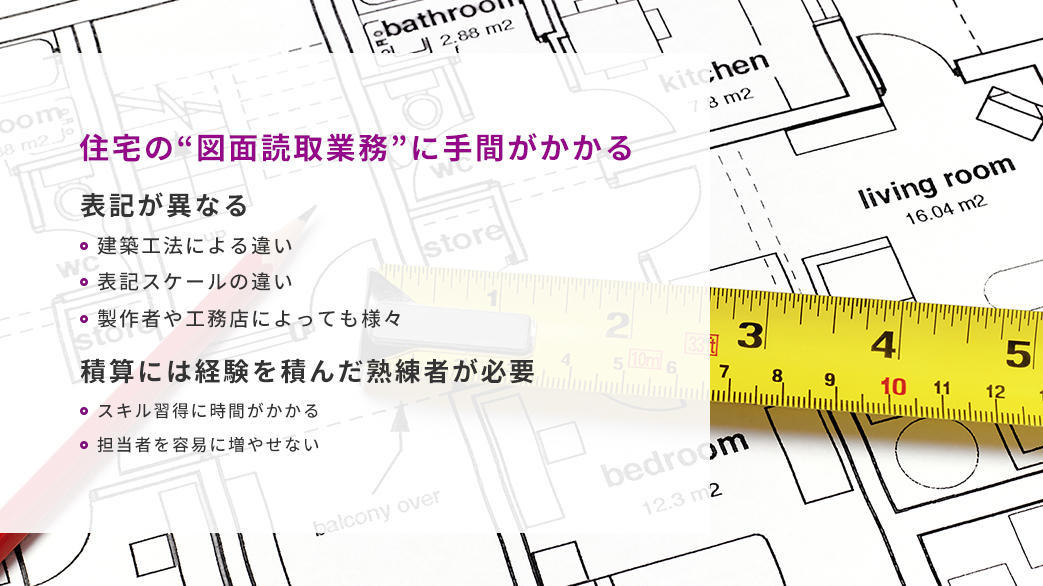
Problem-solving process
The project began by breaking down the drawing reading work into each process, and identifying which work took the longest time and which business impact could be expected when improvements were made. We interviewed not only the DX department but also the actual workers in the business department, and found that scale setting, room name input, room allocation, and opening allocation work accounted for 90% of the total work. It has been found that if this series of steps can be streamlined, a relatively large effect can be expected.
Having decided on the target process, the next step was to check the drawing data and formulate a technical approach for AI. About 300 sample housing drawings were checked one by one, and the technical approach that was most likely to be expected to be accurate was selected. During the assessment, we collected various opinions not only from our in-house data scientists, but also from the CrowdANALYTIX data scientist community to formulate a specific technical approach.
as a result,
(1) In order to achieve accuracy, a model trained individually for each type of drawing style is required, and a general-purpose AI model will not be able to achieve operational accuracy.
(2) Combining multiple AI models and post-processing and performing pixel-based calculations to calculate the final coordinate information seems to be the best approach.
That's what I found out.
After that, we annotated 3,000 drawings of different types. We created 3,000 sets of drawing annotation data and coordinate data as training data sets.
Then the project moved to AI model development. In order to read the drawings, this time, we developed (1) an object detection model, (2) a segmentation model, (3) a point detection model, and (4) an OCR model separately, and combined them to convert the pixel-based coordinates calculated into numerical values in post-processing. I take the. Locate and group each individual room plan location and corresponding street axis in the drawing to identify wall regions. Define the origin coordinates and correct the predicted values from other AI models based on the calculation results of the pillar positions. By combining the processing of multiple AI models, the final vertex coordinate values are derived.
As a result, after multiple iterations and model tuning, we succeeded in developing an AI that calculates the coordinates contained in the drawing with an accuracy of over 90% for the five attributes to be extracted.
Even if a highly accurate AI model is completed, the project does not end there. It is necessary for the DX department, business departments, and development vendors to work together to apply AI to actual operations, modify and add functions as appropriate to achieve the desired effects, and maintain them.
This project is currently in progress. In the future, we will continue to brush up this AI service while conducting field tests and solving problems that occur in actual operation one by one.
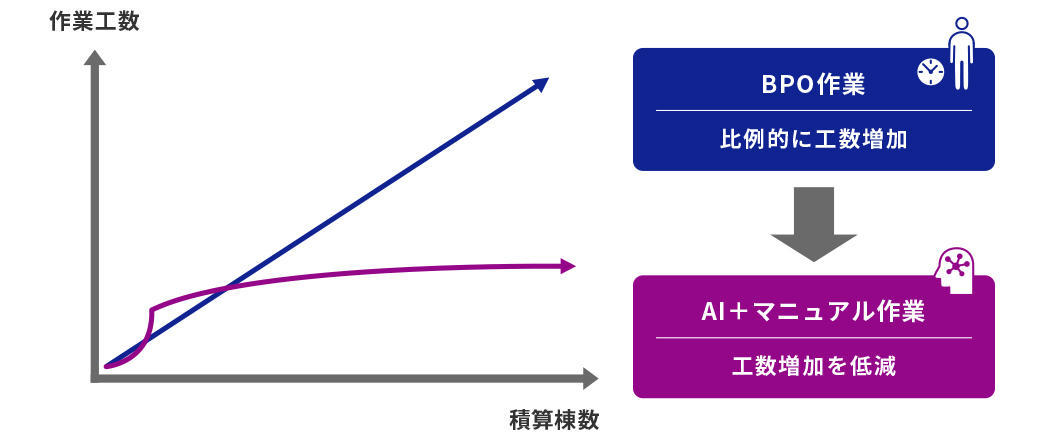
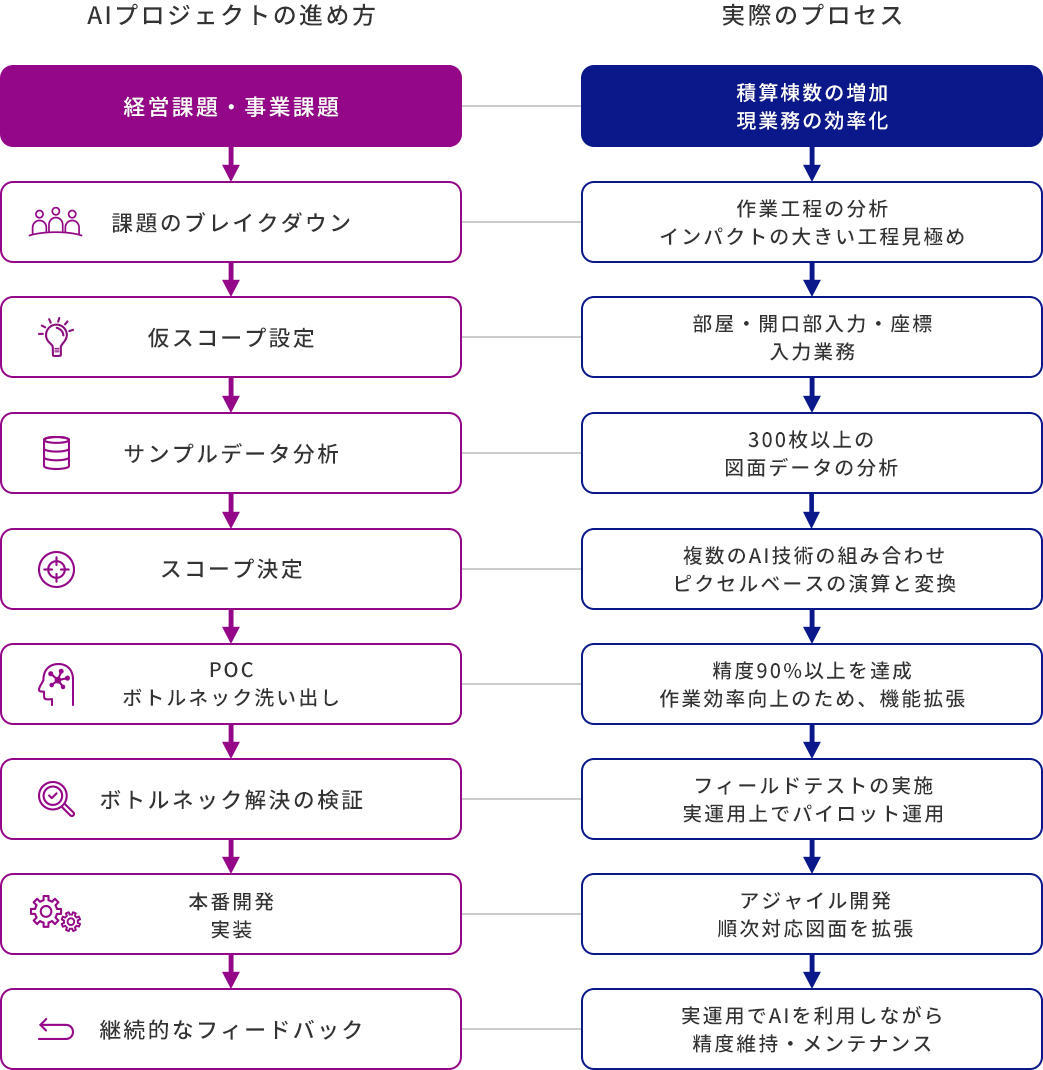
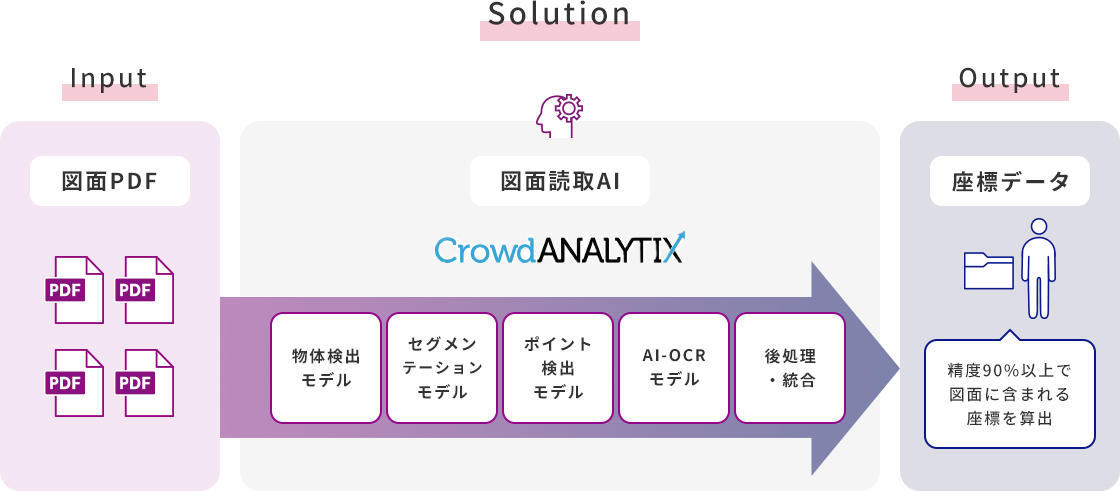