Application for evaluation machine
What is the fusion of Braintech and AI?
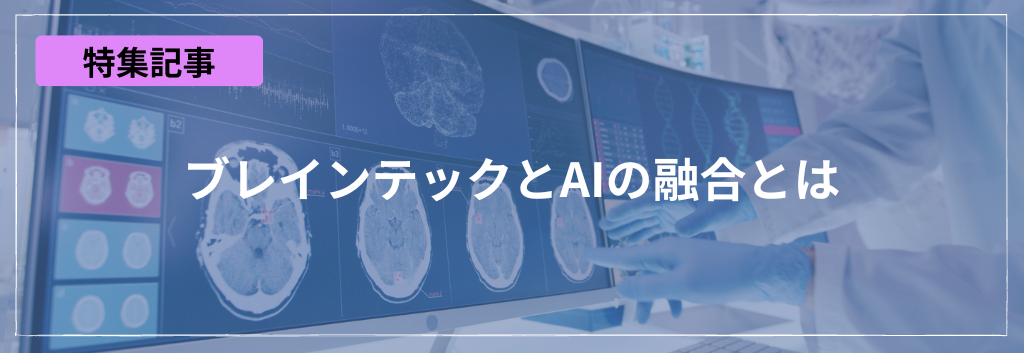
What is “Braintech x AI”?
In recent years, attention has been focused on the fusion of neuroscience and AI, linked to neurons modeled by AI.
The application of neuroscience to IT, such as deep learning, is widely known as "neurotech" in the United States and other countries. At our company, we describe the application of neuroscience to AI as “Braintech x AI,” and this is a field we have been focusing on in recent years.
Although it is still a developing field in Japan, we would like to introduce a research case study on "Braintech x AI", which is expected to expand the possibilities of AI.
EEG measurement and application of AI
About EEG measurement
Currently, non-invasive devices such as fMRI, NIRS, MEG, and EEG are mainly used as EEG measuring devices.
As introduced in our feature article, each has different characteristics such as spatial resolution and temporal resolution, device size and versatility.
There are also so-called wet-type electroencephalographs that are mainly used for research purposes in the field of neuroscience.
As mentioned in "Rapid Fabrication of Low Impedance, 3D Dry Electrodes for Physiological Sensing", many wet-type electroencephalographs apply a conductive gel to the electroencephalogram electrodes and attach them to the scalp when measuring electroencephalograms. increase.
This can reduce the electrical resistance that occurs at the contact points between the scalp and the electrodes, but the subject must wash off the gel on the scalp after EEG measurement, so it is difficult to say that the measurement can be performed easily.
Source: Rapid Fabrication of Low Impedance, 3D Dry Electrodes for Physiological Sensing
https://arxiv.org/abs/2201.03612
On the other hand, dry-type electroencephalographs included in the aforementioned non-invasive devices have been remarkably developed and popularized in recent years.
Dry types have been developed in various shapes, but most of them are shaped like a helmet or headband and have electrodes scattered around the head, and are worn on the head by bonding the electrodes to the scalp. is.
The major difference from the wet type is that it does not require the application of conductive gel, and although it may introduce some noise, advances in development have made it possible to accurately measure brain waves by preprocessing.
Application of AI
Regarding “Braintech x AI”, which I will introduce this time, “Braintech” is a word that combines “brain” and “technology”.
Braintech, as the name suggests, means using IT technology to clarify the mechanism of brain movement and applying that mechanism to IT technology.
The electroencephalogram measurement device mentioned above plays a role in analyzing the mechanism of the brain, and as development and efforts are progressing, it seems that more and more people are hearing the word “braintech.” think.
And the “Braintech x AI” that we are working on specifically utilizes brain wave analysis information as AI learning data. In the past, when it came to learning data for AI, it was necessary to perform one-hot labeling on images in object recognition models.
However, by using brain waves in AI learning, it is possible to treat human tacit knowledge as data, making soft labeling possible, which is a major advantage.
Possibilities of “Braintech x AI”
Braintech and image recognition
One of the possibilities associated with the fusion of Braintech and AI is the ability to understand other people's thoughts and the scenery they see without using words.
In "Seeing Beyond the Brain: Conditional Diffusion Model with Sparse Masked Modeling for Vision Decoding", "MinD-Vis", which was jointly developed by three world-famous universities including Stanford University, produced results with higher accuracy than existing models. I put it out.
MinD-Vis (Sparse Diffusion Model for Human Vision Decoding) makes it possible to reproduce the images that humans perceive by analyzing the activity of the primary visual cortex during image recognition by fMRI. As a result, the SOTA model benchmark accuracy exceeded existing models by 66% in semantic mapping and 41% in generation quality.
MinD-Vis was trained in two steps.
In the first step, Sparse-Coded Masked Brain Modeling (SC-MBM), the vectorized voxels of the fMRI image are divided into patches and converted to embedding using a one-dimensional convolutional layer. These patches are then masked to reduce computational cost while preserving learning power. Finally, we tokenized the masked data into large embeddings and trained an autoencoder to recover the masked patches.
In the second step, Double Conditioned Latent Diffusion Models (DC-LDM), transfer learning was performed by giving fMRI latent images through two pathways to the learned LDM.
The first pathway is directly connected to the cross-attention head of the LDM. The second route is to provide the latent image of fMRI plus time information to the intermediate layer of LDM. Giving time information is a key to overcome individual differences in brain activity information in learning data in the task of reproducing images from fMRI brain activity information.
Due to this architecture, MinD-Vis outperformed the existing SOTA model in the class of evaluation data that was completely different from the training data.
![]()
Source: Seeing Beyond the Brain: Conditional Diffusion Model with Sparse Masked Modeling for Vision Decoding
Caption: Figure 3. MinD-Vis. Stage A (left). Stage B (right).
https://arxiv.org/pdf/2211.06956.pdf
![]()
Source: Seeing Beyond the Brain: Conditional Diffusion Model with Sparse Masked Modeling for Vision Decoding
Caption: Figure 2. Individual Differences in Regions Responding to Visual Stimuli.
https://arxiv.org/pdf/2211.06956.pdf
![]()
Source: Seeing Beyond the Brain: Conditional Diffusion Model with Sparse Masked Modeling for Vision Decoding
Caption: Figure 5. Decoding Performance Comparisons on GOD Test Set.
https://arxiv.org/pdf/2211.06956.pdf
There are individual differences in human brain activity, and acquiring data to train an AI model is a challenge. We have found that it is possible to reproduce images with a high degree of accuracy.
As the social implementation of this technology progresses, it will become possible to communicate beyond various barriers, such as being able to read the objects and scenery recognized by the other party without verbal communication. .
Braintech and natural language processing
Research has already progressed on decoding characters and words using invasive electroencephalography devices, but natural language analysis using non-invasive EEG presents a high hurdle.
Therefore, Meta's AI team presented "Decoding speech from non-invasive brain recordings", which proposed a self-supervised learning model using large-scale data.
![]()
Source: Decoding speech from non-invasive brain recordings
Caption: Figure 1: Method
https://arxiv.org/pdf/2208.12266.pdf
The proposed architecture extracts the contextual representation of a 3-second speech signal from a self-supervised model (wav2vec 2.0) and trains the representation obtained by a convolutional network from matching brain activity.
About 20,000 segments of MEG and EEG brain activity data were used for learning, with each segment being 3 seconds. Since model evaluation uses sentences that were not used in training data, it is equivalent to zero-shot learning and is a more practical approach. By learning the model, the MEG learning data has an accuracy of 67.2 and 72.5, which is better than the comparison model, and the EEG learning data has a low score of 19.1 and 31.4, but it is four times higher than the other models. have become.
MEG, which produced high results in this paper, is large in size and has low versatility, but as hardware development progresses, it can be combined with AI to diagnose, prognose, and restore language processing in patients with poor communication. Etc., the use will be greatly expanded.
![]()
Source: Decoding speech from non-invasive brain recordings
Caption: Table 2: Results.
https://arxiv.org/pdf/2208.12266.pdf
Braintech Psychiatric Diagnosis
Finally, the approach to neurological diseases is essential in the field of Braintech. In particular, the diagnosis of complex neurological diseases by fusion with AI is a hot topic in recent years.
In "Novel EEG based Schizophrenia Detection with IoMT Framework for Smart Healthcare" published by the author who is a member of IEEE, brain activity information obtained from EEG is analyzed by a combined model of convolutional network (CNN) and long short term memory (LSTM). , the diagnostic accuracy of schizophrenia has improved dramatically, and it has been verified that it can be implemented in IoT (IoMT) in the medical field.
![]()
Source: Novel EEG based Schizophrenia Detection with IoMT Framework for Smart Healthcare
Caption: Fig. 1: IoMT-based smart healthcare framework for Sz detection.
https://arxiv.org/pdf/2111.11298.pdf
For model learning, EEG signals of schizophrenia patients and control groups were used as learning data. In this paper, in diagnosing schizophrenia, we used CNN for automatic feature extraction and LSTM for classifying schizophrenia.
As a result of training, the proposed model has a diagnostic accuracy of 99.9% for schizophrenia when utilizing 32-channel EEG and 96.1% when using 5-channel. This is a good result compared to other existing models, and we found that highly accurate diagnosis is possible even with a small number of channels, that is, EEG, which is simple and highly versatile.
In the future, it is said that it is possible to improve the model to a more robust model by increasing the learning data, and it is also suggested that real-time diagnosis at the medical site will be used when evaluating the model.
Source: Novel EEG based Schizophrenia Detection with IoMT Framework for Smart Healthcare
Caption: TABLE XI: Comparison of accuracy among proposed, implemented conventional deep learning/machine learning approaches and the previous works (Best results are highlighted).
https://arxiv.org/pdf/2111.11298.pdf
This time, we introduced various examples of "Braintech x AI" ranging from analysis of brain waves to image recognition, language recognition, and diagnosis of diseases. The cases introduced this time are only a part of "Braintech x AI", but I hope you have understood the various practical possibilities such as the medical field.
Analyzing human brain activity and applying it to technology seems to be a future story, but social implementation is progressing steadily. It will be a field that will further develop in the future, such as the development of devices and the evolution of AI models.
■ Sources of content and papers introduced on this page / References https://arxiv.org/abs/2201.03612 https://arxiv.org/pdf/2211.06956.pdf https://arxiv.org/pdf/2208.12266.pdf |
Related information
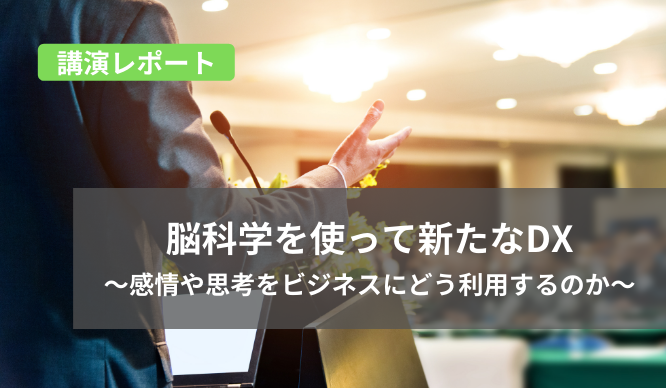
New DX using brain science ~ How to use emotions and thoughts in business ~
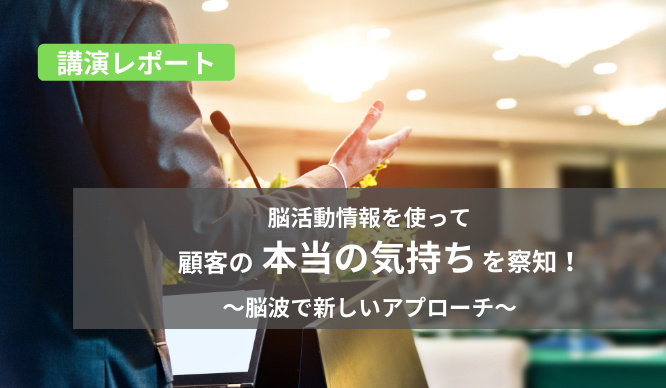
Detect customers' true feelings using brain activity information! ~ A new approach with EEG ~
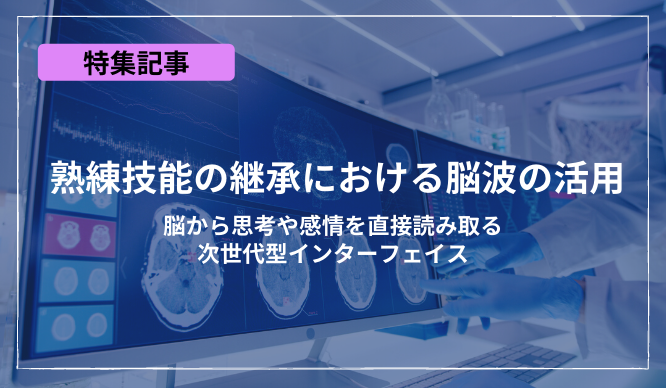
Utilization of Brain Waves in Succession of Skilled Skills -Next-Generation Interface to Read Thoughts and Emotions Directly from the Brain-
free demonstration
Guidance of the demonstration
Would you like to actually measure brain waves?
What is the "skill transfer of experts" based on brain waves? What is AI that learns human consciousness and judgment?
I think there are a lot of unknown things, so why not try measuring your brain waves first and experience what it's like?
After the actual measurement, we will provide support from proposals to implementation and operation so that we can use AI using EEG at the customer's site while including examples of AI utilization in our activities so far. increase.
Learnmore
Document download
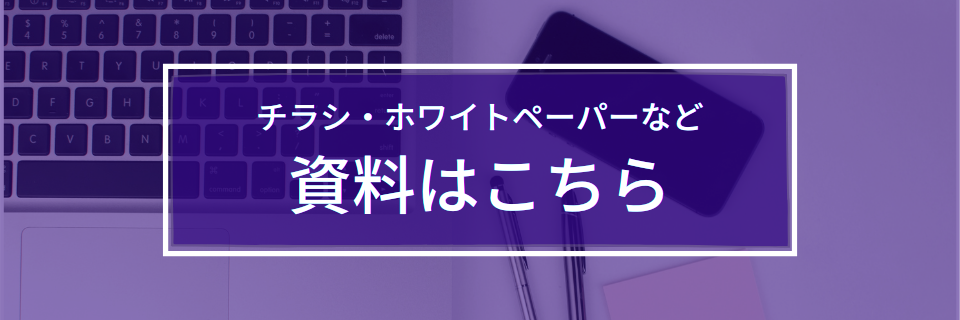
If you want to know the details, please download the materials from here.
Inquiry
If you have any questions regarding this article, please contact us below.