商品の最適価格算出
- POSデータ・商品の購買相関を元に最適価格を算出
- 売上拡大につながるデータドリブンな価格設定により売上・粗利を向上
- リテール
- 商品価格最適化
- 売上向上
- 需要予測
- POSデータ
- CrowdANALYTIX
Business challenge
小売業界において多くのケースでは、商品の価格設定を行う際、商品担当やカテゴリオーナーが競合店や過去の経験から導き出される勘を元にして売価を決めている。
一品一品の粗利が少ない商材においては、顧客単価を増やしながら、お客様に購買頂けるリーズナブルな価格を設定することが重要だ。
季節などを加味しながら、バスケットの商品数量を出来るだけ増やし、かつお客様にご購入頂きやすい最適価格を継続的に算出する、そして店舗売上・粗利を向上していくためにはどのような取組を行えばよいだろうか。
課題
スーパーマーケットは、周辺の住民にとってなくてはならない存在だ。日々の食料品を始めとする商材をリーズナブルな価格で提供し、人々の日々の生活の一部となる店舗でなくてはならない。商品を消費者に求められる低価格で提供しなければならない一方で、店舗の売上・粗利率の向上は常に課題となっている。
一般的に、スーパーマーケットにおける全国平均客単価は、平日約1,950円、土日祝約2,220円前後と言われている。また、一回の買い物で購入される1人あたり全国平均買上点数は、平日約9.8点、土日祝約11点と言われている。売上を向上させるために商品を値上げした場合、より安い価格で商品提供する競合店等に人が流れてしまう。すなわち、店舗の売上・粗利を向上させるためには、①平均客単価を向上させる②平均買上点数を増やす の2つしかない。
顧客の購買に一番ダイレクトに影響する要素は「商品価格」だ。現在商品価格は、各担当者が仕入れ値や過去の売値をベースに、曜日や季節・天候・競合店販売価格等の要素を考慮しながら経験と勘で決められていることがほとんどだ。しかし、現場の担当者も「本当に設定した価格が最適なのか?」「この店舗では特定商品がよく売れる、しかし他店舗ではあまり売れない。要因がわからない。」といった悩みを抱えていることが多い。
一方で、過去それぞれの商品が「いつ」「どれくらい」「どの金額で」「どの商品と一緒に」購入されているのかは、POSデータとして日々蓄積されている。このPOSデータを分析し活用することで、現場の経験や勘に頼らずに商品ごとの最適な価格を導き出し、店舗売上・粗利を改善することは出来ないだろうか?
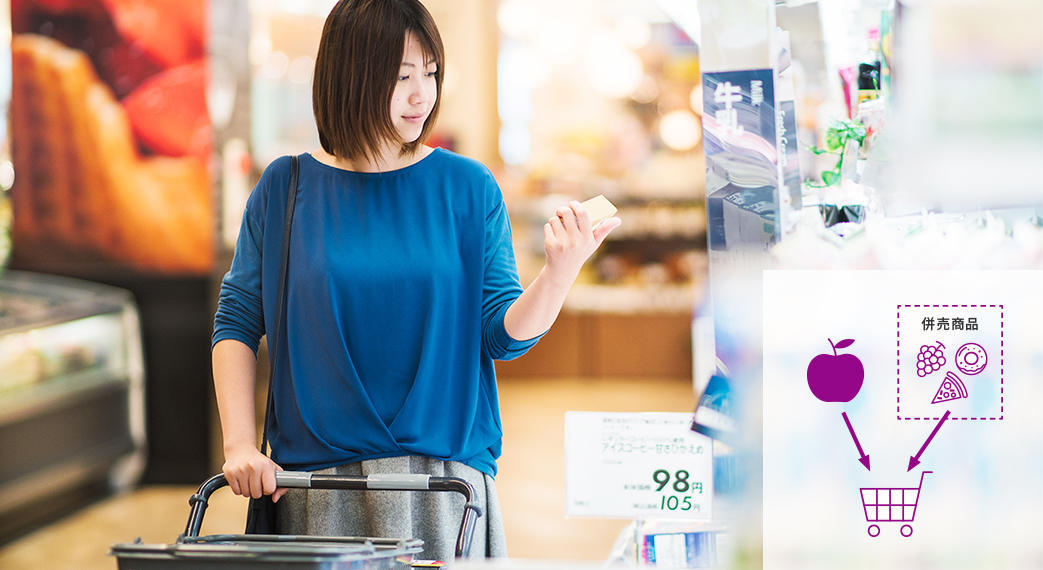
課題解決のプロセス
プロジェクトは、まず過去の売上記録であるPOSデータを徹底的に分析することからスタートした。過去3年分の店舗毎のPOSデータを分析し、それぞれの商品カテゴリから売上上位に来る商品を特定した。
また、商品がディスカウントされた価格変動率、ディスカウント時の売上・売上数量の変化、その際に関連して購入される商品種類や数量の変化まで細かくデータ分析を実施した。当然のことながら、店舗の周辺の環境に応じて、商品をディスカウントしたときの効果は異なってくる。
また、一つの主要商品をディスカウントすることによって、その商品と関連して買われる関連購買商品がよく売れていることが見えてきた(例:カレールーと人参・じゃがいも等)。膨大なデータ分析から、それぞれの店舗の売上の軸となる主力商品に対して最適なディスカウント価格を設定することで、関連購買を誘発し、①平均客単価②平均買上点数それぞれを向上させられるのではないか?という仮説が導き出された。
そこからは、実際に各データを組み合わせ店舗毎のAIモデルの作成に着手した。AIモデルは、各商品のとり得る単価のパターン・曜日・対象季節を入力データとして、それぞれの商品が誘発する関連購買商品の売上額をシミュレーションする。
そして、後処理として、実運用に即した最大ディスカウント率等のビジネスルールを適用する。それらの複数の演算を組み合わせ、AIが各商品毎の売上に対してのインパクトスコアを算出し、そこから関連購買売上額が最も大きくなる商品価格を、実際の店舗の商品価格として設定する。
つまり、AIが全体最適となる各商品価格を導き出し、店舗売上を最大化することが出来るのだ。
AIモデルの開発後、プロジェクトは実店舗でのフィールドテストに移行した。実運用を考慮し、日配・デイリーカテゴリにてAIが算出した価格を適用、店舗規模や周辺環境の類似度を元に複数店舗を選び、数週間に及ぶABテストを実施した。結果として、AIが価格設定した店舗では他店舗と比較し、約2%前後の全体売上額の向上という有意な結果が確認された。
AIプロジェクトは、検証実験で終わりではない。このAIプロジェクトは全国店舗展開することを予定している。マクニカは展開プランの策定のみならず、AIモデルの開発・運用・精度維持・これから先のさらなるAIの活用に「伴走型パートナー」として継続支援していく。
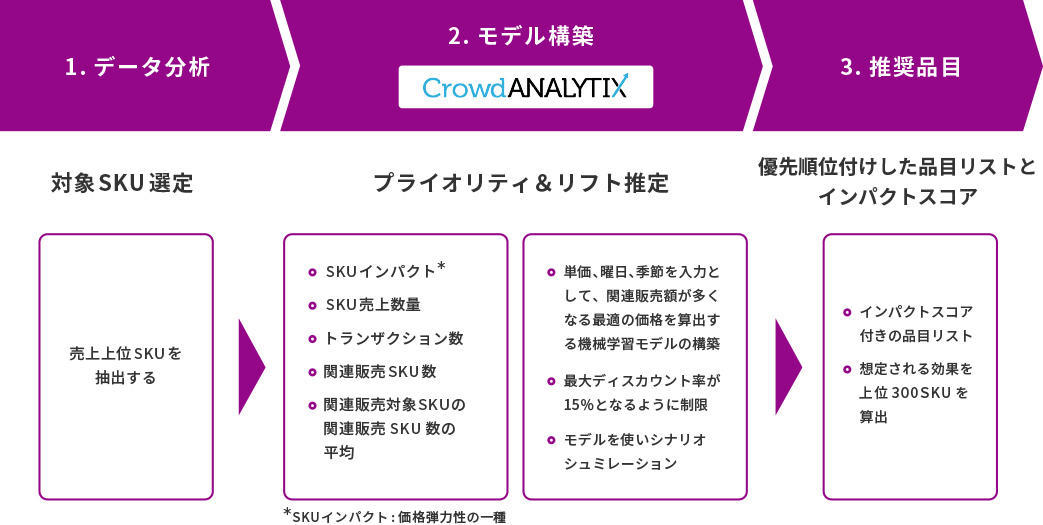
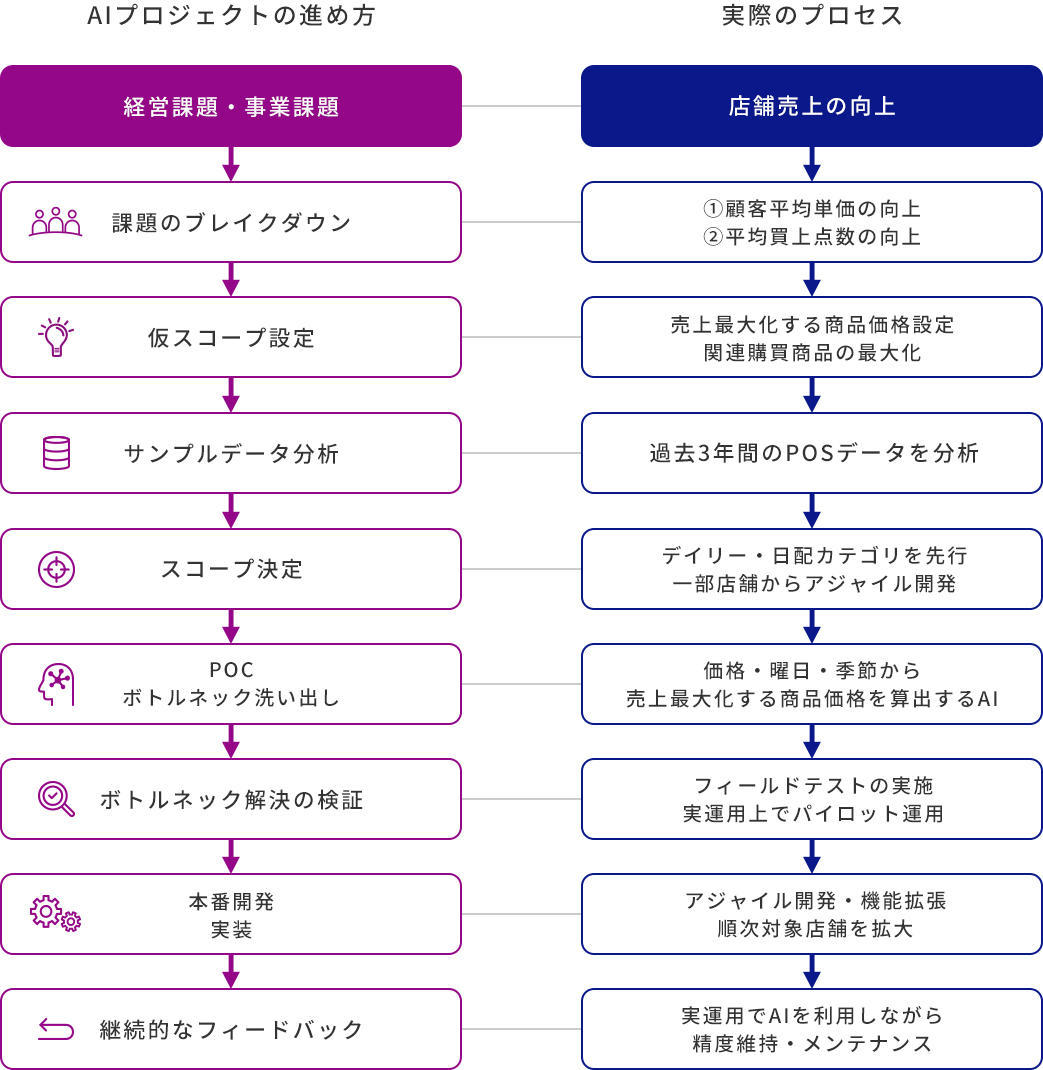