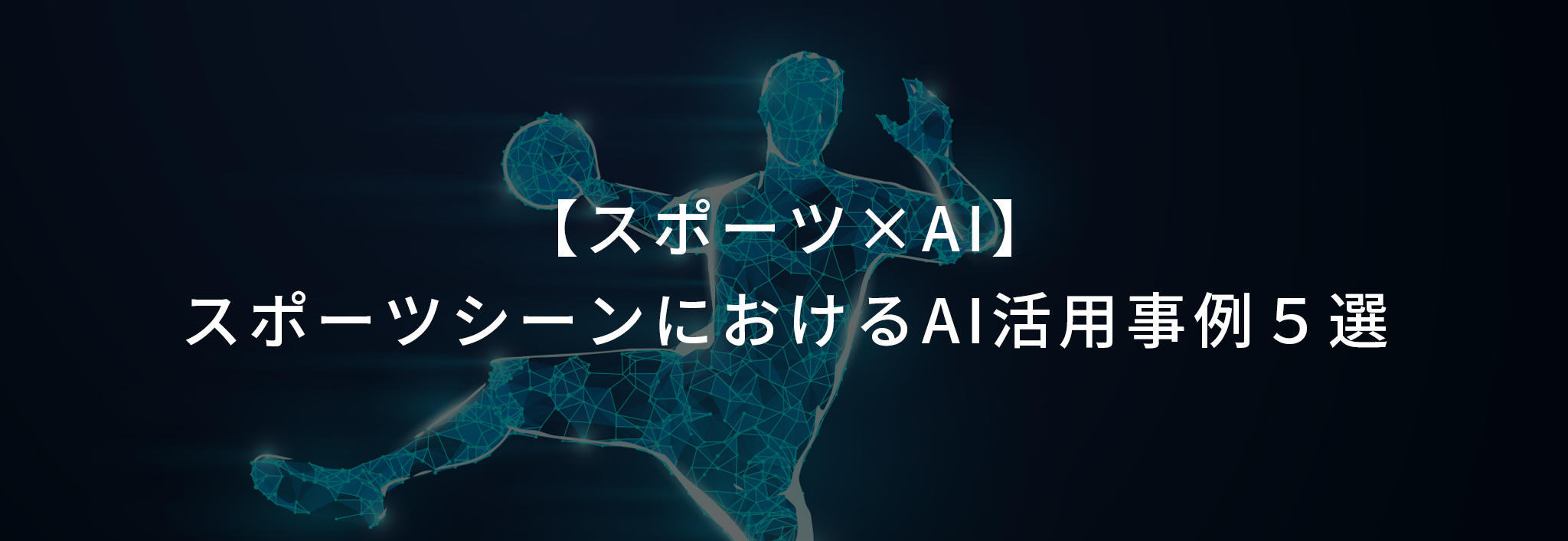
・Those who want to know about global sports x AI utilization cases
・ Those who are looking for trend information on sports analysis methods
・ Those who want to know examples of AI utilization in sports news
Time needed to finish reading this article
5 minutes
What is Sports Tech
Sportstech is a coined word that combines sports and technology, and as the word suggests, it is a field that utilizes technology such as AI in sports. In recent years, I feel that it has been introduced more and more in the news in Japan.
In this blog, I would like to introduce examples of using AI in sports from various angles.
AI Case Study Utilizing Past and Present Data Analysis
Game Analysis in Team Sports-(1)
First, we will introduce an example of using AI in the team sports scene.
The first is the use of AI in ball games.
"DeepSportLab: a Unified Framework for Ball Detection, Player Instance Segmentation and Pose Estimation in Team Sports Scenes" adopted by BMVC2021 (BRITISH MACHINE VISION CONFERENCE 2021), a conference on machine learning in the United Kingdom, We proposed a framework "DeepSportLab" that simultaneously performs three tasks: player segmentation, ball position estimation, and player posture estimation.
In the conventional method, instead of predicting these three at the same time, we used a method that utilizes a model that seeks each task individually. However, when working individually, models corresponding to each task must be run in parallel, and the large amount of calculation and excessive memory usage make it difficult to apply in real time. Furthermore, since the model operates individually, it may ignore the correlation between tasks, which may hinder better performance.
On the other hand, DeepSportLab extracts image features from a single image using CNN (convolutional network), which is commonly used in image processing, and then obtains joint positions and sizes from a network called "Part Intensity Field (PIF)". Furthermore, segmentation is performed to predict which player each pixel belongs to using "Spatial Embedding", a feature learning method used in spatial analysis.
The framework has been evaluated using the DeepSport basketball dataset in the wild, and performs on par with the SoTA method, which handles each task independently. As a result, it is possible to actually use it for operation with satisfactory accuracy, suggesting the possibility of solving problems such as real-time performance, which had been a problem in the past.
Source: DeepSportLab: a Unified Framework for Ball Detection, Player Instance Segmentation and Pose Estimation in Team Sports Scenes
Caption: Figure 1: An overview of DeepSportLab.
Figure 3: Pose recognition and mask segmentation samples.
https://arxiv.org/pdf/2112.00627.pdf
Game Analysis in Team Sports-(2)
Next is the use of AI in the soccer scene.
A joint study by the Massachusetts Institute of Technology and FIFA called "Automatic event detection in football using tracking data" proposes a framework for automatically acquiring football event data that previously had to be partially acquired manually. . The "event data" mentioned here is a log (history) with information such as the time, position, and player of events that occurred during the game, such as passes and shots.
In this framework, positional data indicated by 2D coordinates of all players and balls that can be extracted from the video, and those coordinates are used to perform calculations and acquisitions using player and ball tracking data. In terms of acquisition accuracy, they were able to detect most events with an accuracy of about 90 %.
Using this framework makes it easier to acquire soccer event data. Furthermore, by utilizing the player and ball tracking data used to acquire event data, it will be possible to contribute to team analysis and development.
Source: Automatic event detection in football using tracking data
Caption: Figure 1: a) Proposed computational framework, along with information generated at each step. b) Schematic
detailing all possible labels for the attributes ball control, event name, dead ball event and from set piece on
the output events table.
https://arxiv.org/pdf/2202.00804.pdf
Game analysis in individual sports
Unlike team sports such as basketball and soccer, table tennis and swimming are individual sports. Even in sports that do not involve team play, data analysis using AI is used to support athletes.
In recent years, due to demand in the security and nursing care fields, spatio-temporal neural networks (Spatio-Temporal Neural Networks) have been used to detect fine-grained actions (fine-grained actions). There is an increasing amount of research on models for predicting . With this model, it is possible to make predictions by taking into consideration continuous time information, not just from a single frame of a video that is obtained instantaneously.
It is also being used for action analysis in the sports field, and there is research to detect and classify table tennis strokes using a spatio-temporal model.
In the study of table tennis stroke classification shown in the image below, a video made from RGB images, posture data of table tennis players estimated from the images, and velocity obtained from the movement of the object between each frame of the video are represented as vectors. We used three modalities of optical flow and obtained excellent performance on both detection and classification tasks.
Source: Three-Stream 3D/1D CNN for Fine-Grained Action Classification and Segmentation in Table Tennis.
Caption: Figure 1: Frames of an “Offensive Forehand Hit” stroke from TTStroke-21 with its estimated pose and optical flow.
https://arxiv.org/pdf/2109.14306.pdf
Stroke of a forehand hit when attackingestimated attitude in(Up)and optical flowー(under)
![]()
Source: Three-Stream 3D/1D CNN for Fine-Grained Action Classification and Segmentation in Table Tennis.
Caption: Figure 2: Three-Stream architecture processing RGB, optical flow and pose data in parallel with spatio-temporal convolutions.
Figure 3: TTStroke-21 dataset.
https://arxiv.org/pdf/2109.14306.pdf
The proposed spatio-temporal model pipeline (with three modalities of input) (Figure 2) and an example of detected strokes (Figure 3 (c))
Use Cases of AI to Predict Future Actions
Furthermore, in individual sports such as table tennis, tennis, badminton and other rally competitions, the relationship with the competitors influences the style of play.
Research into sports analysis in rally competitions has mainly focused on quantifying strokes in ball games, such as the examples introduced in the previous section on game analysis in individual sports, and reading information about strokes from competition videos.
Recently, however, future prediction analysis is also being conducted, such as predicting subsequent strokes, including shot type and position information, from past consecutive strokes. The predictions are useful for coaching, strategy, and in-competition predictions, as well as past and current analysis.
Stroke prediction was first considered as an application of S2S, which utilizes the vector of the input sequence (the immediately preceding stroke) to output the output sequence (subsequent strokes).
However, this model had three problems. First, it is difficult to leverage for mixed sequences in which two players rally. Second, unlike general sequences, predicting a stroke requires multiple outputs for one prediction, such as the type of shot and the position to be struck. Third, it is difficult to read complex information from a rally sequence, as strokes vary depending on the player's style of play, position, and rally situation.
We will introduce the latest stroke prediction method that overcomes such problems and focuses on badminton rallies.
ShuttleNet: Position-aware Fusion of Rally Progress and Player Styles for Stroke Forecasting in Badminton proposed a model "ShuttleNet" that infers in which direction and how strokes are returned in rally competitions. ShuttleNet integrates two encoders / decoders (TRE: Transformer-based rally extractor; TPE: Transformer-based play extractor) that detect the information of the rally and the rally respectively, and the weight of the information and the weight of the position. It is a framework consisting of a Position-aware Gated Fusion Network (PGFN) that fuses the context of the stroke, and a Prediction layer that outputs multiple pieces of information about subsequent strokes.
In a quantitative evaluation using badminton competition footage, ShuttleNet was found to be superior to various sequence prediction models in terms of both shot type and position prediction. It suggests that utilizing TPE to detect player information contributes to the superiority of stroke prediction in two-player competition.
Source: ShuttleNet: Position-aware Fusion of Rally Progress and Player Styles for Stroke Forecasting in Badminton
Caption: Figure 2: Illustration of the ShuttleNet framework.
https://arxiv.org/pdf/2112.01044v1.pdf
Game analytics for sports coverage
Finally, we will introduce AI technology related to video analysis of team sports in the news field. Watching highlight videos of specific players or teams is a common sight among sports fans, but for some time now computer vision societies have used face detection or uniform numbers to identify athletes. was widely used. However, in order to support the generation of highlight video that cuts out a specific player, it was considered to be highly difficult because it was necessary to precisely understand the video of non-verbal expressions.
Therefore, "Distantly Supervised Semantic Text Detection and Recognition for Broadcast Sports Videos Understanding ' uses Semantic text detection and Text recognition to make it possible to understand the game by comparing the live commentary in the sports video with the text on the watch face during the competition. We also made it possible to automatically generate training data using semi-supervised learning (more precisely, a method called Distant Supervision).
In text detection, we first classify the inside of each frame of the sports video into a clock face or background, and then detect the team name, time, and quarter from the text area cropped from the clock face. Then, we use text recognition to convert the text that contains sports-specific notation so that the model can understand it, and then compare the detected text with the content of the sports commentary to understand the content of the competition. Automatic generation of training data uses Knowledge Constraints (KCs), which summarize sports-specific logical rules for team names, times, and quarters, to extract appropriate training data for the model. Previously, we used the technique of matching end-to-end sports footage with commentary, which showed the complexity of using the same technique for highlight footage. We conclude that text detection and text recognition can be refined by a framework trained by
Source: Distantly Supervised Semantic Text Detection and Recognition for Broadcast Sports Videos Understanding
Caption: Figure 1: (a) Comprehensive understanding of video segment by aligning frame with corresponding play-by-play commentary
(b) Process of end-to-end text recognition from video frames. (c) Effect of scene
Transitions in contextual objects (clocks) in contiguous time interval frames.
https://arxiv.org/pdf/2111.00629v1.pdf
Summary
This time, we introduced four AI utilization cases related to sports tech. In recent years, research on game analysis has become active in a wide range of areas, such as player and team development and sports coverage, for various types of competitions such as team play and individual competition.
As in the case above, the use of AI for the purpose of training was obvious from the point of view of the athletes themselves, but with the rapid development of networks such as the spread of 5G, the use of AI from the point of view of spectators and especially for sports reporting will be a focus in the future. It will be done. In addition, game analysis technology is already being used from the perspective of game commentary in sports commentary, and demand from core sports fans should increase further.
■ Sources of content and papers introduced on this page / References Ferran Vidal-Codina, Nicolas Evans, Bahaeddine El Fakir, John Billingham, “Automatic event detection in football using tracking data”, Figure 1: a) Proposed computational framework, along with information generated at each step. b) Schematic
Wei-Yao Wang, Hong-Han Shuai, Kai-Shiang Chang, Wen-Chih Peng, National Yang Ming Chiao Tung University, Hsinchu, Taiwan, “ShuttleNet: Position-aware Fusion of Rally Progress and Player Styles for Stroke Forecasting in Badminton” ,Figure 2: Illustration of the ShuttleNet framework., Avijit Shah, Topojoy Biswas, Sathish Ramadoss, Deven Santosh Shah,“Distantly Supervised Semantic Text Detection and Recognition for Broadcast Sports Videos Understanding",Figure 1: (a) Comprehensive understanding of video segment by aligning frame with corresponding play-by-play commentary |