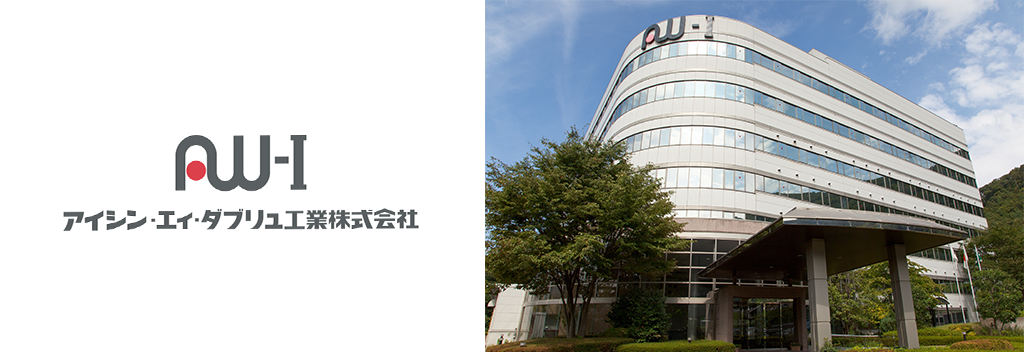
Case of Aisin AW Industries Co., Ltd.
少数精鋭チームが挑む生産ラインの品質管理AIを自社開発
アイシン・エィ・ダブリュ工業(AW-I)は、トルクコンバータ(T/C)とトランスミッション(T/M)の専門メーカーだ。
30年以上に渡り高品質なT/Cを生産できる理由は、同社が長年培ってきた品質管理(以下QC)と、世界に誇る最新技術を取り入れた高度な生産ラインにある。
2018年8月、同社はスマートな工場を達成するために、既存のQCとAI技術を組み合わせて生産ラインを管理する「DQC(デジタル・クオリティ・コントロール)」の実現を目指すプロジェクトを開始させた。
最初の取り組みはレーザ溶接工程のQCにAIを活用すること。
しかしAW-Iの真の目的は、AIの活用を単一工程の改善で終わらせることではない。社内のあらゆる課題解決へ水平展開し、コア技術全体の底上げに寄与することである。
ここでは、チームの立ち上げからAIの技術開発に至るまでの取り組みについて話を聞いた。
USER PROFILE
■企業情報:アイシン・エィ・ダブリュ工業株式会社
■事業内容:
「品質至上」
アイシン・エィ・ダブリュ工業株式会社は、1983年の創立以来「品質至上」の基本理念のもと、オートマチックトランスミッションの主要部品である、トルクコンバータとトランスミッション部品の専門メーカーとして、人と環境にやさしいクルマ社会の発展を目指し、長年培ってきた固有技術と技能に日々磨きをかけ、独自の価値創出に取り組んでいる。
summary
background
・Realization of clean and smart factories
・Establishment of advanced QC utilizing AI
・Promotion of AI technology development projects
Purpose
・Improve the efficiency of time-consuming quality control
・Using AI to estimate defect factors and reduce load
Task
・ Acquisition of knowledge and technology to quickly turn the AI development cycle
・ Acquisition of technology to prepare data handled by AI
・Acquisition of technology that facilitates horizontal deployment of AI
Attempt
・Accelerate development by acquiring development methods and knowledge
・Collecting data that describes quality by making full use of IoT technology
・Development of preprocessing programs for AI analysis
・Promoting team activities that are familiar with manufacturing and AI technology
Obsession
・Use of OJT to break free from rigid thinking
・Selection of tools that can be used immediately on site
・Collaborate with factories to build AI and support manufacturing
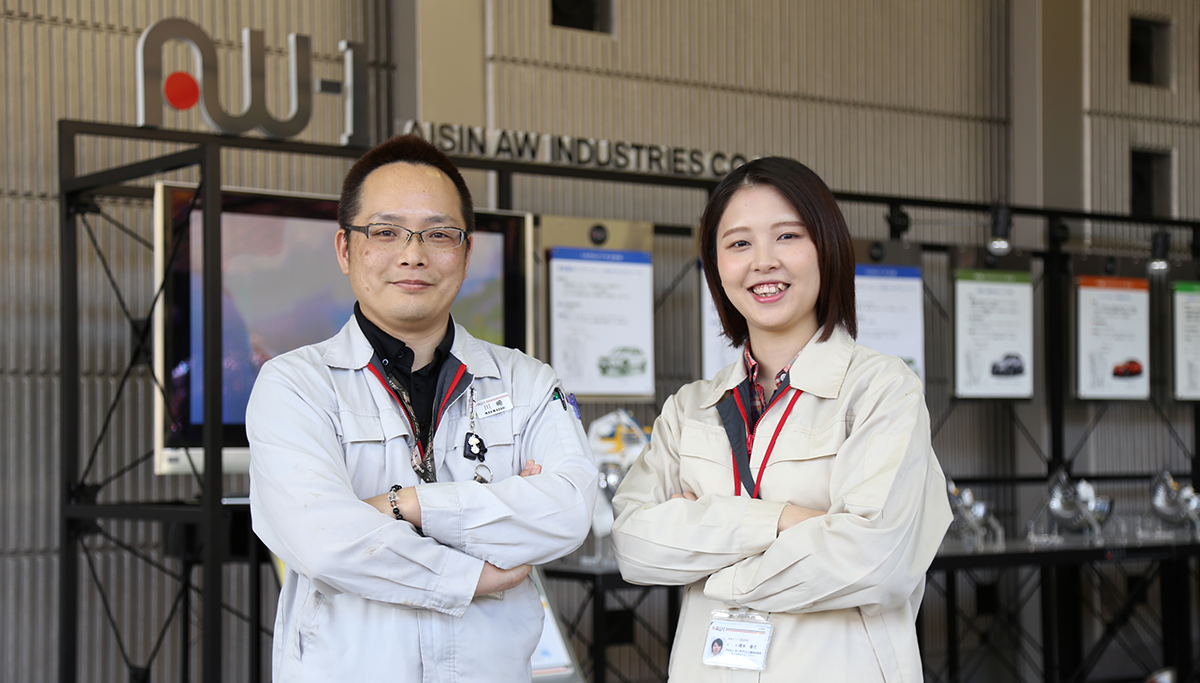
アイシン・エィ・ダブリュ工業株式会社 生技開発部 川崎 正哉 氏(左)
アイシン・エィ・ダブリュ工業株式会社 生技開発部 橋本 優花 氏(右)
株式会社マクニカ 楠 貴弘、本村 健登
background
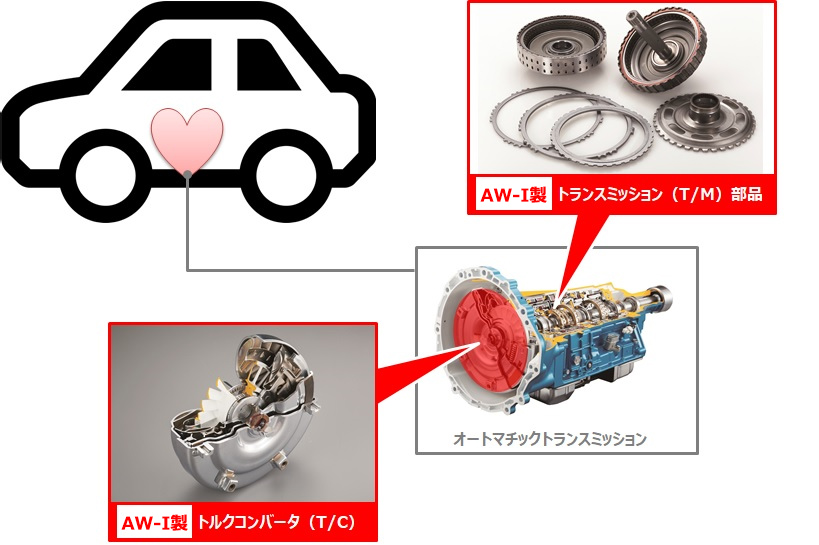
高度な生産ラインのQCにAIを活用
アイシン・エィ・ダブリュ工業(AW-I)は、トルクコンバータ(T/C)とトランスミッション(T/M)の専門メーカーだ。
創業時はプレス加工を得意とするT/Cの部品メーカーであったが、市場からの要望によりプレス以外の加工や組み立て作業にも挑戦し、1989年には同社初のT/C Assy製品(組み立て済み製品)の出荷に成功した。
その後30年以上に渡ってAT車の主要部品であるT/CとT/Mを主力製品として、経営理念である「品質至上」のもと、高品質なモノづくりに取り組んでいる。
ー Mr. Kawasaki
We have achieved the development of innovative production technology and aim to realize a clean and smart factory.
Cleanliness is a requirement for any factory.
We want to be even smarter, in other words, we want to create a factory that can produce AW-I's proud high-quality products at low cost, achieving stable quality no matter where or who produces them.
These goals cannot be achieved with traditional QC alone.
Therefore, we have started a project to digitize the information we have accumulated over many years and utilize AI analysis technology to realize DQC (Digital Quality Control), which advances the QC of the production line. raised.
The team that develops AI that can analyze and utilize huge amounts of data is a small group of three people, including Mr. Kawasaki.
Mr. Hashimoto, a data scientist who specializes in AI technology development, talks like this.
ー Mr. Hashimoto
Members who are good at IoT technology collect data from the production line, and I, who is in charge of AI technology, analyzes it using various techniques.
Kawasaki, who is well-versed in production technology, supervises the team, so we can develop toward the realization of realistic DQC with a strong awareness of the site.
Also, if we need additional data for analysis by AI, we can quickly propose it to the whole team because the number of people is small, and we can promote the project efficiently.
Task
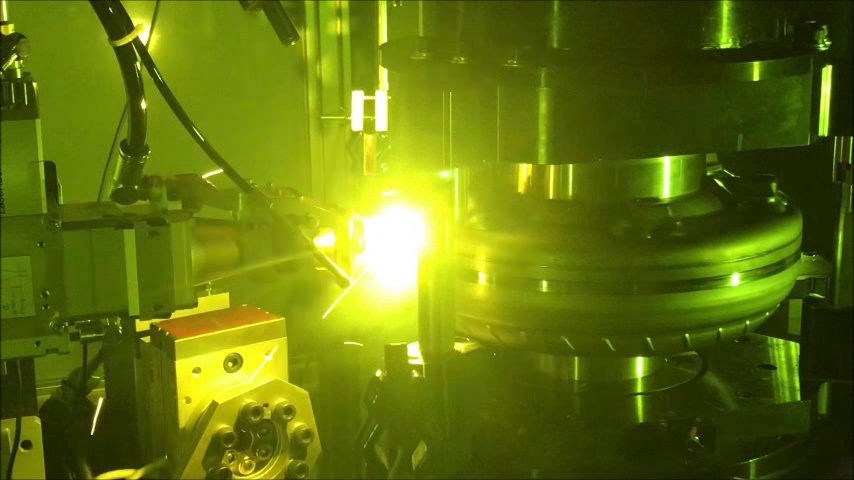
Launch an in-house AI development team from scratch
レーザ溶接工程は、従来の溶接工程よりも優れた加工ができる一方、工程の管理項目が多く不良が発生した際、要因を特定するまでに時間がかかる。
特に複数の要因が重なった不良はさらに複雑で特定は困難を極める。
そこでAW-Iは、人が気付けない要因を推定するためAI活用の開発に着手した。開発では以下の内容が課題となった。
1. データ分析・AI開発サイクルをクイックに回したい
DQCの実現には、AIの開発活用だけでなく、データ収集をはじめ、要因分析運用に向けたシステムの構築、業務シナリオの検討といった様々な要素に対応しなければならない。
また、これらの課題解決を最短経路で進めるには、開発を始めやすいパッケージ型でありながら、分析要件に即した形へカスタマイズできるAIツールが不可欠となる。
2. I want to know how to prepare the data handled by AI
In order to utilize AI, in addition to the huge amount of data collected in the past, it is essential to acquire data close to the processing point in order to improve the accuracy of the analysis, preprocess it, and convert it into input data.
In addition, it is necessary to repeatedly check whether the AI functions as expected using the converted data.
3. I want to run an AI-savvy team in-house
The company hopes to use AI not only in the laser welding process, but also to solve various internal issues in the future.
Therefore, in addition to developing AI, technical support for team management is required.
We were looking for a partner who understands this situation, organizes cutting-edge AI technology information, and proposes the optimal development method.
Attempt
Delicate support for areas where a small team of elites lacks hands
"Industry 4.0," the keyword for FA innovation, was the impetus for AW-I to incorporate AI technology into its production line.
The company had already been working on promoting Industrial-IoT (IIoT) since 2017, and in August 2018 began a project to realize DQC using AI with Macnica as a partner.
- Mr. Kawasaki
In this project, in order to apply AI to factor analysis, it was essential to create a system that could develop the technology in-house.
Macnica provided us with the utilization methods and knowledge needed to make AI our own.
There were proposals from other companies as far as providing tools, but Macnica confirmed our technical level and provided information on how to operate AI, including the effects of introducing AI and sharing technical information that would serve as a basis for learning on your own. They provided a wide range of support, including suggesting essential design techniques.
Motomura, who works in smart factory consulting at Macnica, looks back on this.
ー Motomura
When we first met, we heard that AW-I would like to promote a project to develop AI for QC on its own.
Therefore, we created a proposal centered on collaboration with the Company AI Research & Innovation Hub, which has a wealth of domain knowledge in manufacturing processes and knowledge of the latest AI technology.
Macnica 's support content
1. Proposal of development methods and tools to accelerate AI technology development
First, Macnica proposed the optimal AI development method to solve AW-I's problems.
- Kusunoki (AI Research & Innovation Hub Principal)
We are investigating cutting-edge AI technologies around the world every day.
In this project, we aimed to solve problems with a sense of speed, proposed AI development methods and tools, and also supported the development of algorithms that lacked tools.
In this project, we proposed three types of AI tools to AW-I, and AW-I finally selected the product.
- Mr. Kawasaki
Based on our previous knowledge, we know that there is a certain degree of connection between sensor data and processing data when measuring defective products, and our AI tool analyzes sensor data and outputs a ranking of factors. I was looking forward to this feature.
In fact, while exchanging opinions with Macnica, we narrowed down the necessary requirements and were able to select a product that met our initial objectives.
2. Support for converting measurement data to AI input data
Next, we supported the development of a data conversion program that converts the vast amount of information AW-I has collected to date into input data for AI.
First, Macnica implemented a data conversion program in Python and confirmed that the converted data functioned correctly as input for AI.
After that, he handed over the program and development knowledge to Mr. Hashimoto, who was in charge of AI technology development.
- Kusu
It takes a lot of time to prepare input data for AI.
Therefore, based on the knowledge of AI that we have accumulated so far, we support work such as how to handle the time axis of data, how to shape waveforms, and labeling for learning. I made the groundwork for the data conversion program while repeatedly confirming the compatibility.
ー Mr. Hashimoto
You shared not only the development of the data conversion program, but also the design and implementation of the program.
In addition, he taught us the basics of the Python language in a hands-on way so that we could develop data transformations on our own in the future.
We have now learned more about Python and development tools on our own and are now able to develop our own data analysis programs.
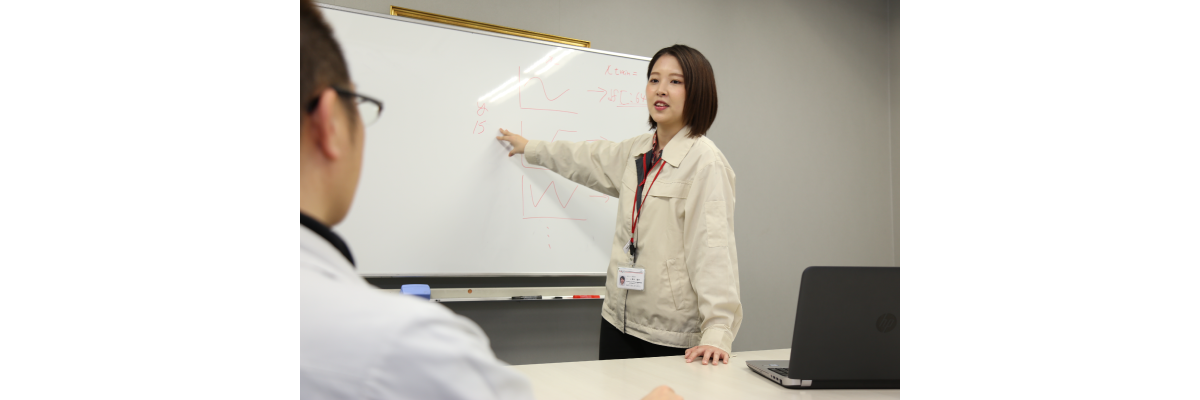
3. Knowledge transfer of AI-related technologies
For this project, AW-I was able to start operating an in-house team that is well-versed in AI technology.
In addition, for team management, Macnica implemented a "knowledge transfer" to support the management of the AI technology development team.
ー Mr. Kawasaki
We were able to obtain information on management, such as how to manage progress when developing and utilizing AI, which we had no know-how in-house, how to set up the development process, and how to manage the accuracy of AI.
In addition, we received a lot of technical knowledge, such as technical points, technical information collected from the latest papers, and how to use development tools.
Right after the start of the project, hands-on training gave us the opportunity to acquire various knowledge and ask questions about technology, so we were able to solidify our foothold for using AI.
Knowledge transfer also has benefits for Macnica.
ー Motomura
Many of the projects that do not carry out knowledge transfer and have a system where they want you to talk to them if something happens will fail at the PoC stage.
In order to lead the project to success, it is important to have an AI engineer among the customers, and we are conducting knowledge transfer to develop AI engineers.
As our customers gain a deeper understanding of AI, the number of consultations that come to mind with regards to such issues will increase, which is a great advantage for us as well.
Commitment in this project
Aiming to realize a smart factory by combining production technology with IoT x AI
Finally, we asked each member about their commitment to the success of this project.
1. Utilization of OJT to escape from rigid thinking
Human resource development was a major theme in this project.
- Kawasaki
It is impossible to design a QC system without acquiring a production engineering mindset.
However, if you first gain a deep understanding of production technology, your way of thinking will become rigid and it will be difficult to come up with new ideas.
Therefore, each member specialized in production technology, IoT technology, and AI technology, and the project was promoted in a way that effectively mixed opinions within the team.
Although I leave the AI technology to Hashimoto, I also need to understand AI to a certain extent, and I believe that Hashimoto also needs to understand production technology.
This exchange of information was part of on-the-job training, during which we learned about different technologies as we carried out development.
Macnica 's hands-on training was also helpful in deepening our mutual understanding.
2. Select a tool that anyone can use right away
For the AI being developed, we worked with Macnica to select a GUI development tool that would provide comfortable operation in actual operation, with an eye toward future horizontal expansion beyond the PoC.
- Kusu
Each AI tool has its quirks.
In addition to being able to handle production line data, we selected a GUI that can graphically display the information necessary to identify the cause of defects in an easy-to-understand manner, and that is rich in explanation of analysis results.
In addition to shortening the time to understand the analysis results of the tool, we recommended it as an environment where you can learn the essence of data analysis and AI.
3. Cultivate AI in cooperation with production sites and support with completed AI
The members in charge of IoT technology are responsible for matching the data analyzed by AI with the knowledge of the field.
ー Mr. Kawasaki
The input/output data of AI is not directly shown to the workers at the production site.
Since the factory operates based on principles, if there is a question about the data input to AI, the members in charge of IoT will visit the production site and collect information.
Through these activities, the members in charge of IoT have all the data, and Hashimoto can focus on AI development, realizing extremely efficient development.
Furthermore, regarding the use of AI in factories,
ー Mr. Kawasaki
Since AI grows based on learning, it is impossible to explain what each parameter that makes up AI means.
For this reason, we are not entrusting all the QC of the production line, which requires explanation, to AI, and are promoting behind-the-scenes use of it as a role in suppressing defects in the processing process.
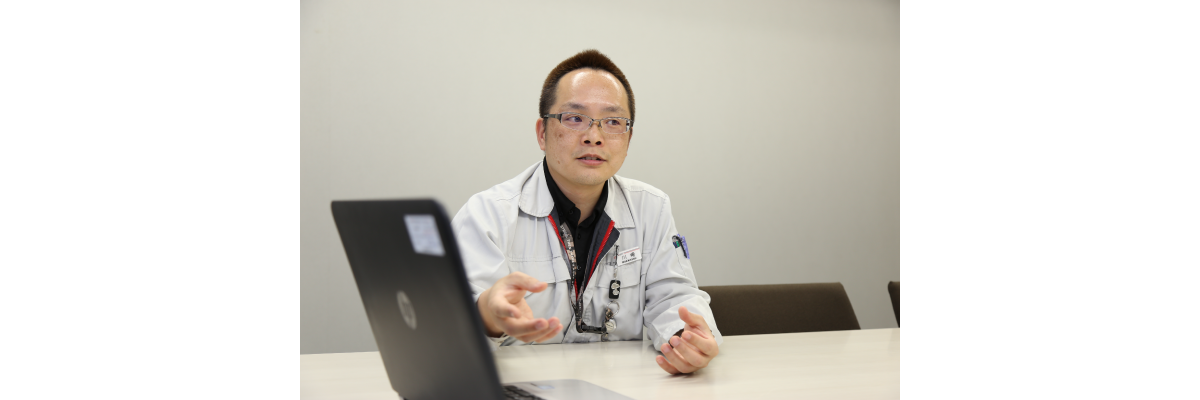
from now on
AW-I has begun to move towards further AI utilization at production sites
Efforts to realize DQC with AI can be horizontally deployed not only in the laser welding process but also in all production processes in the future.
Mr. Kawasaki talks about the future goals of AW-I as follows.
ー Mr. Kawasaki
By applying DQC, it will be possible to suppress defects in the manufacturing process instead of the inspection process.
We also expect AI technology applications such as factor analysis and defect prediction to prevent defects from occurring.
In the future, we would like to further promote the use of AI and create new technologies with our own hands that can make a strong contribution to smart factories.
*Information and company names in the text are as of July 2020.