Visual inspection automation by AI
Manufacturing industry DX starting with the digitalization of visual inspection processes
「撮像」「判定」「運用」の3つのノウハウを統合したワンストップソリューション!!
Do you have any of these problems?
I want to digitize and automate the work that depends on individual skills in the inspection process.
It takes a huge amount of time to design feature extraction (parameters) for inspection equipment...
The work of NG classification requires a lot of man-hours for inspectors, and we want to promote efficiency and automation.
Those who have concerns about AI utilization and are at a standstill
外観検査は、顧客からの高い品質要求に応え、信頼を確保する上でとても重要な工程となります。
属人的な検査方法からの脱却を目指し、自動化に取り組まれている企業も増えてきました。
その背景には、人の目に代わる撮像技術の向上と、人の脳に代わるAI技術の飛躍的な進歩にあります。
特にAIにおいては、これまでの「人の目で判別できるものはAIでも判別可能」という時代から、「人の目では判別できないものでもAIなら判別可能」という時代に変わってきました。
しかしながら、これら技術の進歩によって自動化実現の可能性は高まりましたが、一方で、より専門的な知識が求められるようになり、自社の人材だけでは、プロジェクトを推し進めることが難しくなってきました。
弊社では、これから外観検査の自動化に取り組まれる方を対象に、安心してプロジェクトを進めていただけるよう、専門性が求められる「撮像」「判定」「運用」の3つのノウハウを統合し、自動化に向けた全体計画の策定から運用支援までワンストップでご提供いたします。
In addition to automating the appearance inspection process, we analyze the causes of defects using acquired image data, and provide support for quality improvement solutions that identify defects and digitization of the entire manufacturing site.
Three things necessary for Visual inspection automation
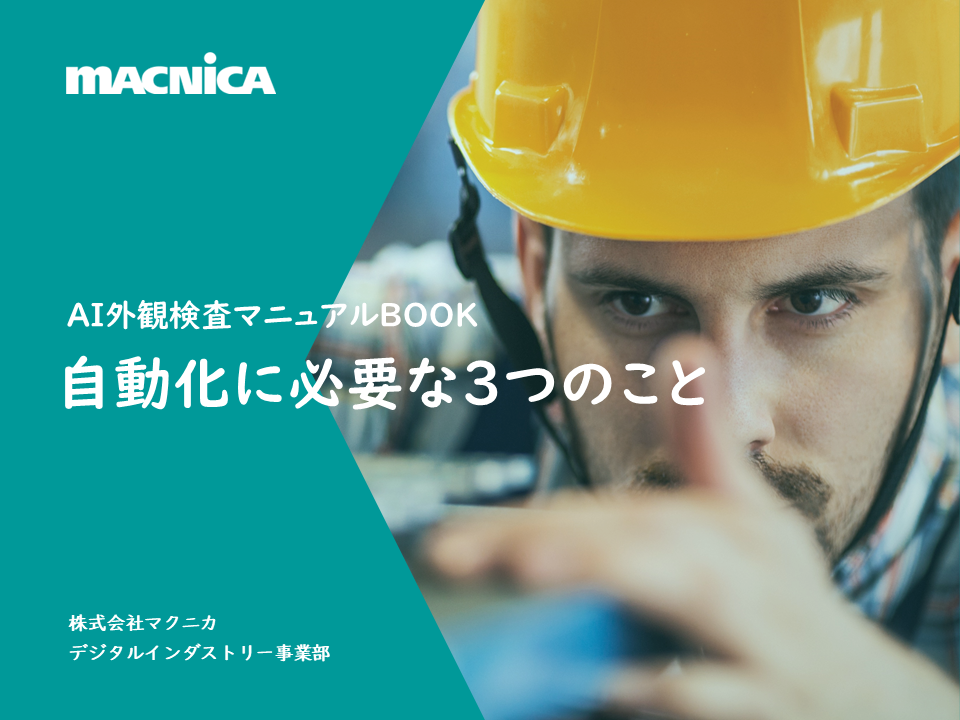
AI visual inspection manual BOOK
This is a free material that explains in detail the points to consider: "imaging", "judgment", and "operation", which are the three essential elements for Visual inspection automation using AI!
外観検査自動化では、「撮像」「判定」「運用」の3つがそれぞれ密接に関わっています。
どれか1つだけでは自動化は実現できません。
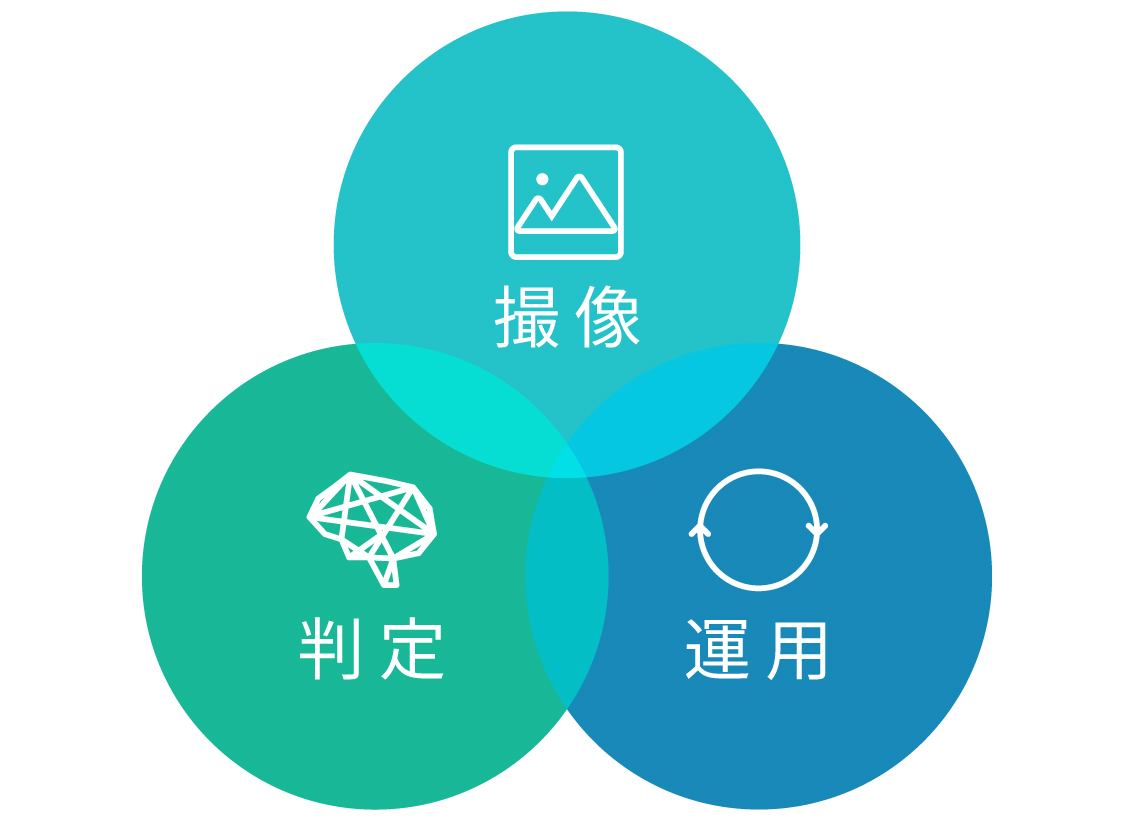
Point of Imaging: Building an Environment That Can Detect Defects
Judgment point: Eliminate oversight
Operational points: data management, maintenance
しかし、上記ポイントを押さえただけではうまくいきません。
なぜなら…
●撮像フェーズの注意点 = 対象をしっかり撮ることだけにフォーカスしてしまいがち
【失敗事例】全体撮像枚数が膨大になり結果的にタクトタイムが規定オーバーに
【解決事例】撮像視野を拡げた状態でAI学習を行い、見逃しのないモデル開発に成功
●判定フェーズの注意点 = NGを検出するモデルを学習量だけでカバーしようと躍起になってしまいがち
【失敗事例】特定のNG検出の精度向上が頭打ちになってしまう
【解決事例】撮像側で照明を増やし、見逃しがなくならない特定のNGに特化した撮像を実施し高精度なAI判定に成功
●運用フェーズの注意点 = 装置開発&AIモデル開発に成功はしたが…
【失敗事例】AIモデルの管理・運用ができる人財を確保できず、アップグレードができない…
【解決事例】運用後の業務フローを事前に策定し対策立案・人財確保を行い運用レベルを保つことに成功
▼
それぞれのフェーズで発生しうる課題も、相互に補完することで解決できる可能性もあります。
つまり、それぞれのポイントを押さえたうえで、「撮像」「判定」「運用」のノウハウを総合的に検討して進める必要があります。
01 撮像について
It is a very advanced technology that makes full use of optics, software technology and image processing technology.
As an example, there are the following methods.
- A method to improve inspection performance by changing the lighting angle and lighting direction
- A technique for reducing reflected light with a modified filter
- Detection method by synthesizing images with different lighting directions
- A method that separates colors for each lighting angle, such as coaxial lighting, ring lighting, and colored lighting, and detects them in batch imaging.
Imaging example 1 Engraved character inspection
手法:照明方向の異なる画像を合成することで検出する
通常撮像画像では判別しにくい、掘り込み文字もくっきりと判別できることがわかります。
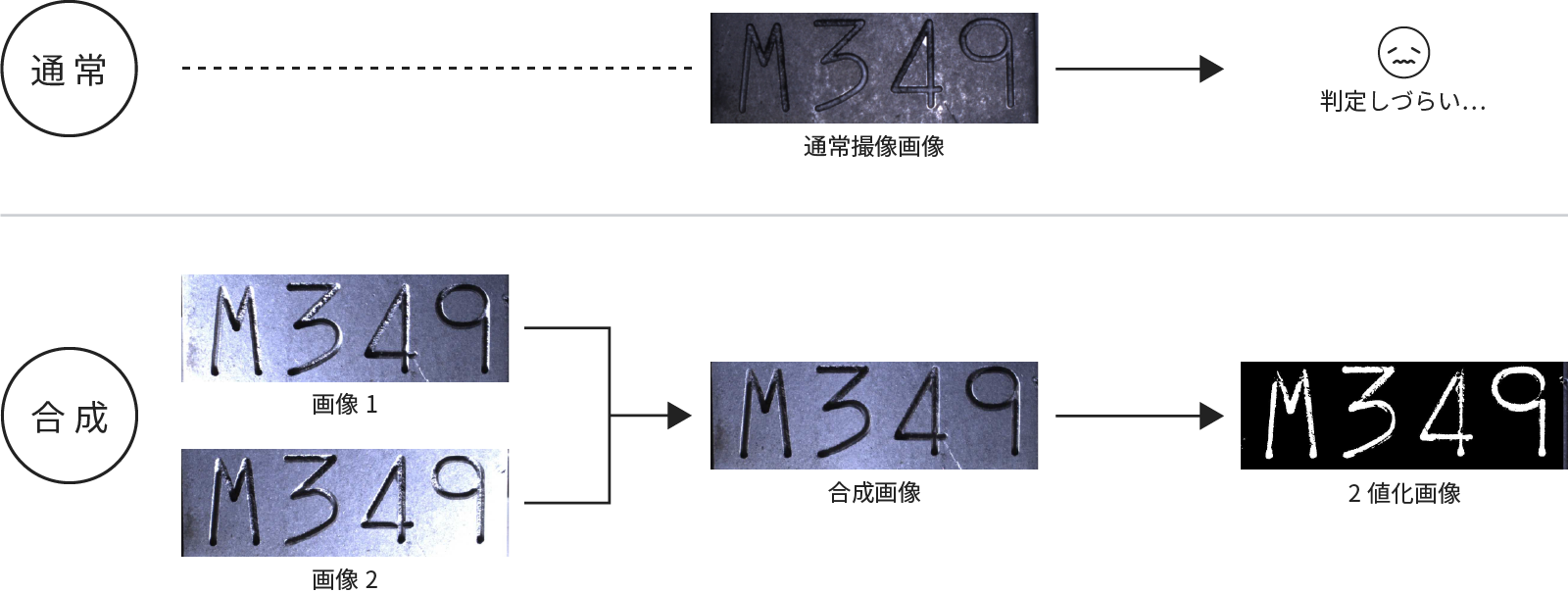
Imaging example 2 Solder inspection
手法:同軸照明、リング照明、色付き照明など、照明の角度ごとに色を分けて一括撮像で検出する
一括撮像のため、撮像時間を短縮できます。
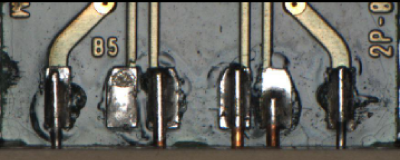
Coaxial illumination image
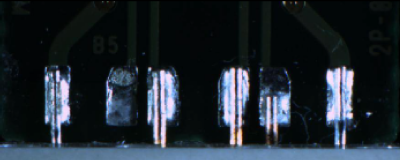
ring lighting picture
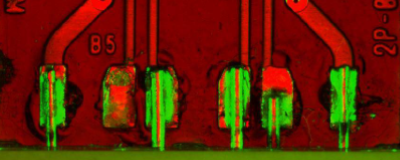
Colored lighting image
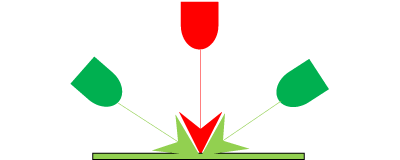
Image of colored lighting
≪標準的な撮像装置導入の流れ≫
*The order may change depending on the situation.
Inspection standard |
・不良項目や判定基準など、第三者が判断できる不良の基準をご提示いただきます |
▼ | |
Mass production inspection process |
・検査タクトを確認させていただきます |
▼ | |
Imaging |
・不良が画像に写るかどうかを確認します |
▼ | |
imaging test |
・画像から不良を検出できるかどうかを確認します |
▼ | |
Equipment introduction |
Using the imaging mechanism created by image inspection, we will manufacture a visual inspection device that meets your needs. |
≪撮像について、よくあるご質問≫
02 判定について
Here, we will introduce the points of introducing a judgment model by AI.
≪検査方法と特性について≫
We will introduce the general characteristics of AI image inspection, visual inspection, and conventional image inspection.
It is important to recognize and understand the characteristics of each in order to select the method that fits the problem to be solved.
目視検査 |
AI画像検査 |
従来型画像検査 |
|
---|---|---|---|
Ease of design |
― | △ | × |
バラつきの許容 |
〇 | 〇 | × |
distinguishing features |
〇 | 〇 | △ |
consistency of judgment |
△ | 〇 | 〇 |
Reliability of operation |
△ | 〇 | 〇 |
processing speed |
× | △ | 〇 |
exact measurement |
× | × | 〇 |
Inspection examples for which AI image inspection is easy to apply
Occurs with existing imaging tests
Reduction of gray zone (suppression of over-detection)
Dirt and scratches that cannot be dropped into rule-based inspections
Classification and sorting based on differences in characteristics, such as distinguishing between
Application example of AI image inspection Inspection of workpieces with uneven patterns
人の目では判別が困難な、素材系ワークにおける異物検査です。
現在のAI技術では、このように難易度の高い異物検知も可能です。
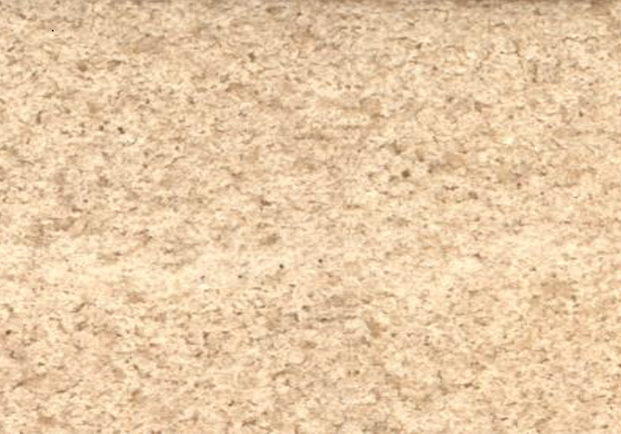
Inspection object
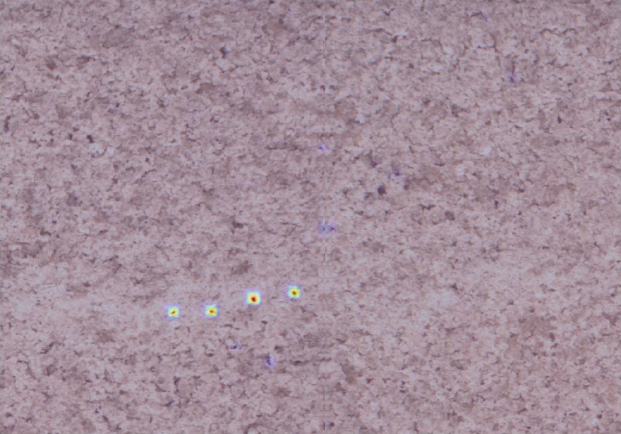
AI reaction point
≪判定モデル構築の流れ≫
sample image |
After concluding a non-disclosure agreement, we will keep sample images (OK/NG). |
▼ | |
Data preprocessing |
Consider the data structure by combining dozens of candidates for image cropping, reduction, binarization, etc. |
▼ | |
study |
Start learning by selecting tools, selecting networks, examining various parameters |
▼ | |
inspection |
We verify the accuracy of the AI model built by learning, consider it, and select the optimal model. |
▼ | |
Accuracy report |
We will report the accuracy of the developed AI model |
≪AI判定について、よくあるご質問≫
Q.
What is the difference with existing inspection equipment?
A.
AI is not omnipotent, and should be used according to its characteristics. We recommend that you consider "separation" with existing inspection equipment and "complementation by combination" according to the purpose and issues of introduction.
Q.
How do you evaluate the reliability of AI?
A.
AI has been regarded as a "black Box", but recently, technology that visualizes the basis of judgment (e.g. heat map) has been established. It is desirable to use such technology to judge the reliability of AI.
03 運用について
In the operation phase, we will perform AI retraining to improve the accuracy of the judgment model.
Since AI relearning requires specialized knowledge, Macnica supports customers by providing AI operation services.
operational data |
Full-scale collection of image data, which was scarce at the PoC stage |
- AI Operation Service -
|
▼ | ||
AI model |
AI learning using operational data |
|
▼ | ||
AI model |
Implement the developed AI model |
|
▼ | ||
Accuracy verification |
Link the results of regular human inspections and AI judgments |
|
▼ | ||
Relearn |
Relearn images that AI misses/overdetects |
How to proceed with the Visual inspection automation project
When starting a project, Macnica first formulates an overall plan to address the client's unique challenges.
We also provide a variety of services at each phase to enable our clients to proceed with their projects with peace of mind.
How to proceed with the project |
service |
|
-Phase 1- |
Grasping the current situation / Creating a plan / Examining cost effectiveness / Creating a work flow / Scrutinizing the estimate |
Consulting Services |
▼ | ||
-Phase 2- |
Examination of imaging environment (camera, lighting, image size, tact time) / simple equipment estimate / rough estimate of operation equipment |
・Imaging verification service |
▼ | ||
-Phase 3- |
Required specifications / business flow / functional requirements / system configuration / data storage system / production linkage system / quotation |
Operation phase requirements definition service |
▼ | ||
-Phase 4- |
Imaging device development / transportation system / operation system introduction |
・AI model development service |
▼ | ||
-Phase 5- |
Operation image data collection / AI model development / AI model implementation |
・AI operation service |
Beyond automation
Manufacturing industry DX starting from Visual inspection automation
Various realizations can be obtained from the data (facts) obtained by visual inspection.
for example…
不良が多くなってきたデータがあれば
→前工程で起きている不良原因の究明と改善した方がいいのでは?という気づき
検査数量自体が減っているというデータがあれば
→生産数が変わるならば、人員シフトを構築した方がいいのでは?という気づき
→実はチョコ停ドカ停が多かったということが判明すればCBMした方が良いのでは?という気づき
このように取得したデータから得られる気づきが、DX実現に向けての足がかりとなります。
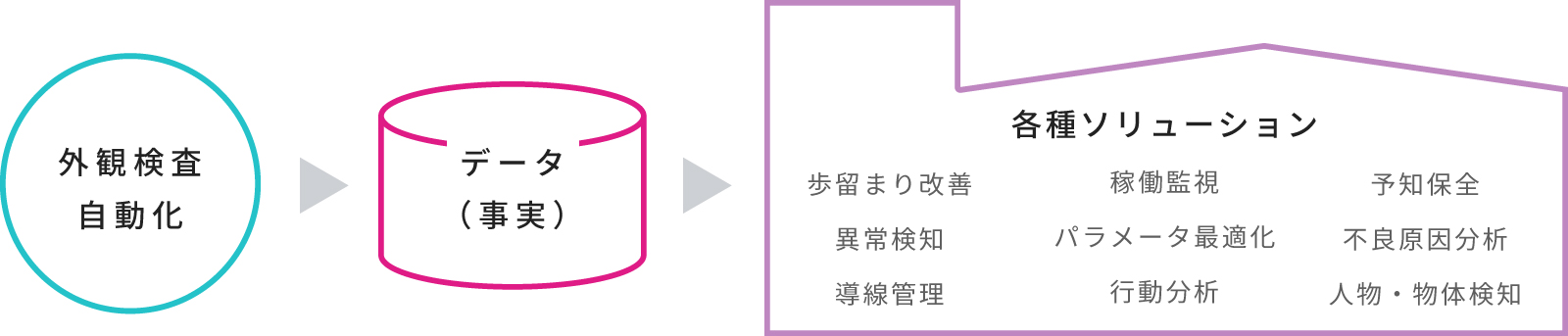
セミナー動画
外観検査自動化の進め方が分かるセミナー動画を公開中!
外観検査自動化プロジェクトについて、もっと詳しく聞きたい!という方は、ぜひご視聴ください。
撮像パートナーの新電子株式会社様、AIパートナーである株式会社シーイーシー様にご協力いただき、4部構成でお届けしています。
- [Introduction] Steps for visual inspection optimization
- [Imaging] The most important step for automating visual inspection, the secret of building an imaging environment
- [AI Utilization Edition] Appearance Inspection x The Forefront of AI ~Keep an Eye on AI's Actual Values and Possibilities~
- [Automation, Beyond] The path of factory DX starting from the optimization of visual inspection
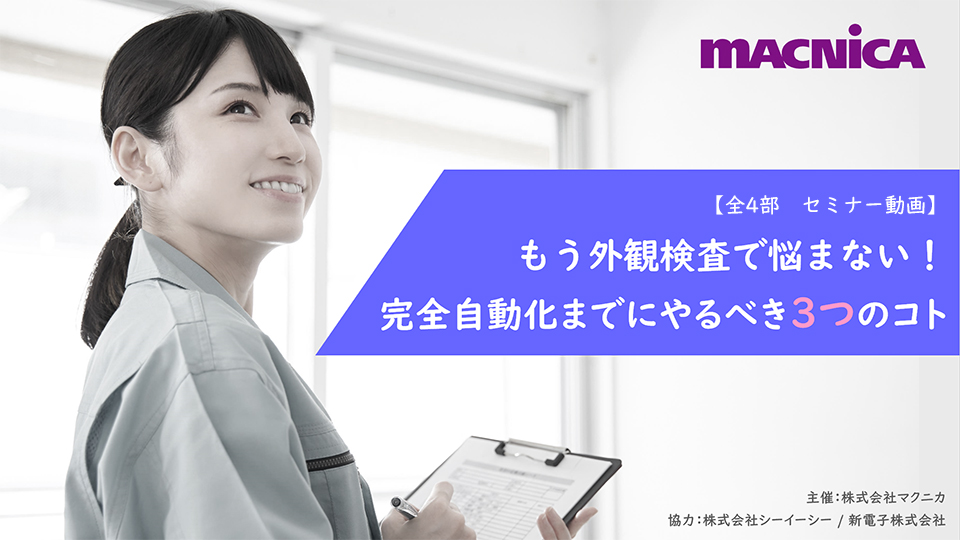