Introduction
The use of AI has been gaining momentum in recent years, and recently the term “endpoint AI” has begun to be used.
This article describes the characteristics of endpoint AI compared to cloud AI and edge AI.
In addition, we will introduce examples of endpoint AI and the AI chips used.
■ Contents
1. What are Cloud AI and Edge AI in the first place?
2. Beyond Edge AI! ? Characteristics of endpoint AI
3. Examples of Endpoint AI
1. What are Cloud AI and Edge AI in the first place?
Many conventional systems send data to the cloud and perform AI analysis on the cloud.
Cloud AI is excellent for large-scale and accurate analysis, but there are various issues such as network connection is required, analysis takes time, privacy care is necessary, etc. Therefore, it is necessary to complete some processing on the edge side. is being sought.
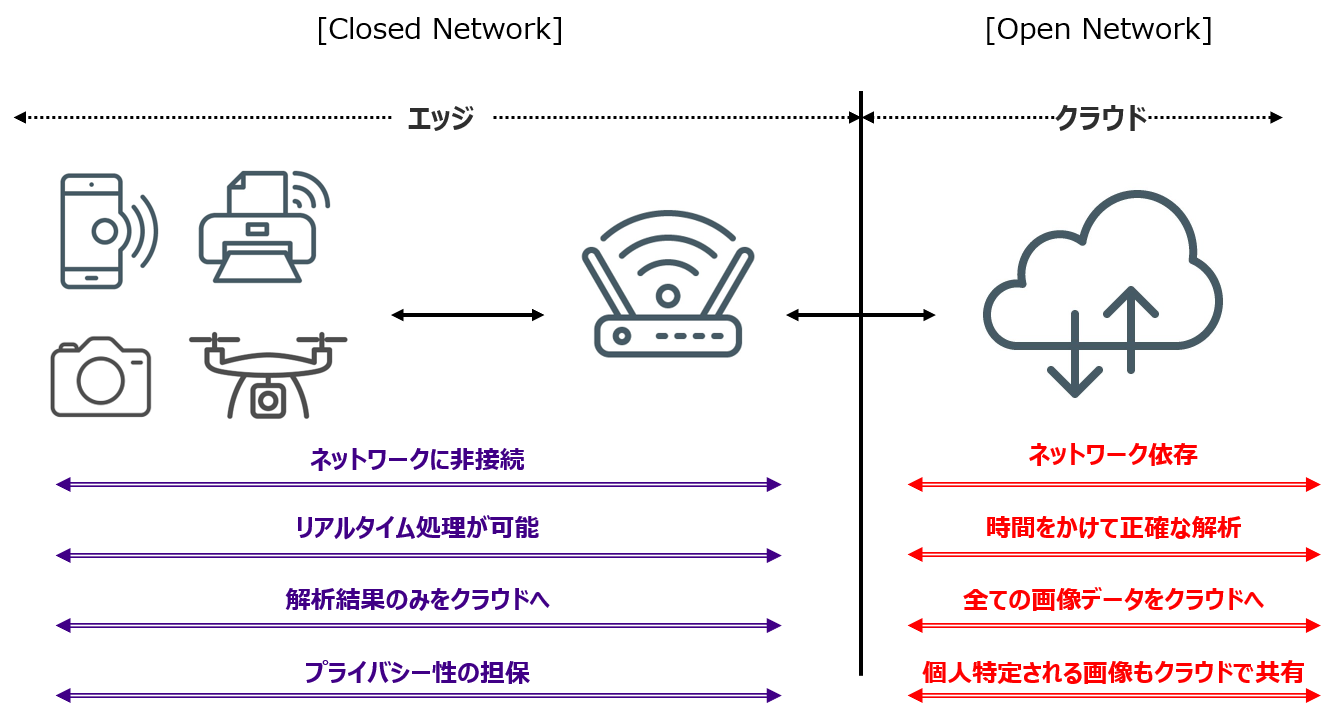
For example, in a system that analyzes what is shown in images captured by a camera, we will compare the case where cloud AI is used and the case where edge AI is used.
For cloud AI
First, in the case of cloud AI, all image data will be uploaded to the cloud.
High-precision analysis is possible because it is not affected by the lack of computing power of the specifications of embedded devices and layer restrictions of NN models.
Another attractive point is the ease of re-learning (easiness of setting up the environment) when the NN model needs to be updated.
On the other hand, there are concerns about increased communication costs due to image transmission, privacy concerns when people are in the image, and concentration of load due to cloud analysis of all data.
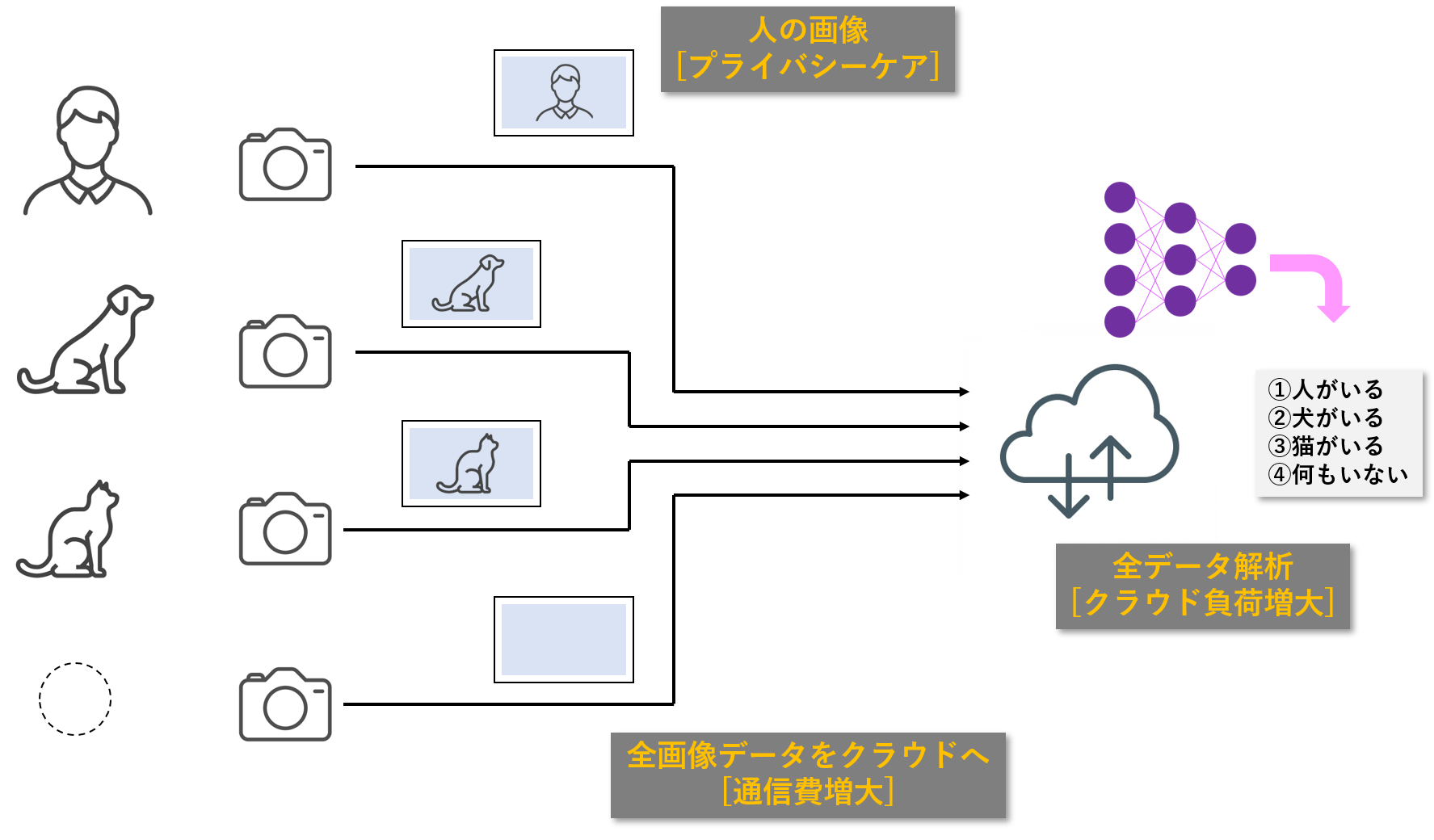
For Edge AI
Next, in the case of edge AI, it is possible to solve the issues I mentioned earlier.
Specifically, as shown below, a Closed Network is built to build an environment where AI inference can be performed locally.
In this way, only the results can be shared to the cloud, and there is no need to share image data.
Compared to sharing “image data” of a person to the cloud, sharing “only the result” of whether or not there is a person is advantageous in terms of privacy, communication costs, and cloud load.
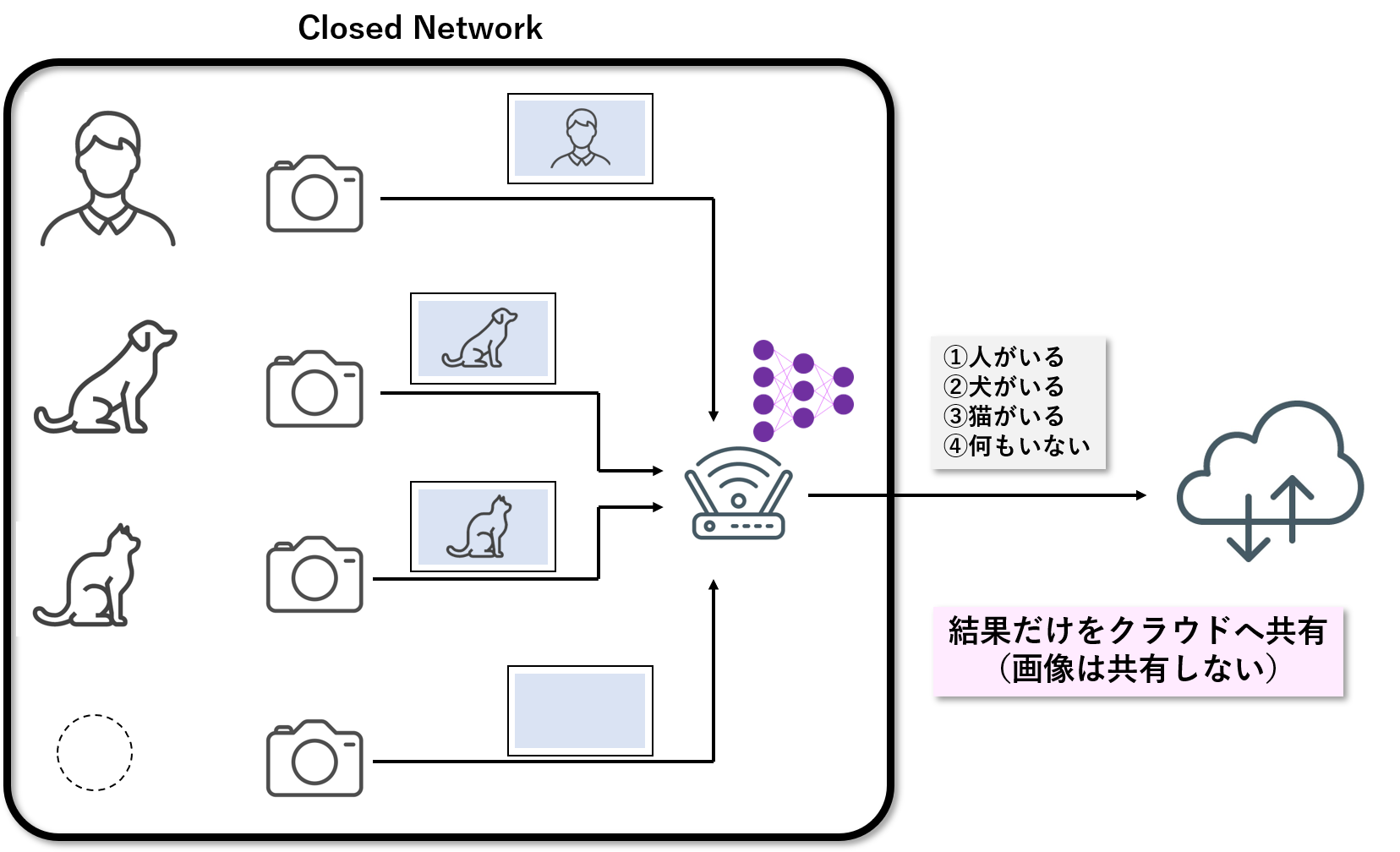
In this way, edge AI is expected to solve the problems of cloud AI, but in reality, its use cases are still limited.
One of the reasons for this is the local environment construction.
In order to make inference, it is necessary to install an expensive repeater (edge server, edge gateway, etc.), and it is necessary to review the entire system configuration according to the specifications of the repeater.
Therefore, it is necessary to carefully examine the merits of AI processing at the edge and the cost of changing the existing system.
2. Beyond Edge AI? Characteristics of endpoint AI
Edge AI was to complete processing within a closed network without uploading image data to the cloud.
Even within a closed network, endpoint AI means that processing is completed only on the terminal side.
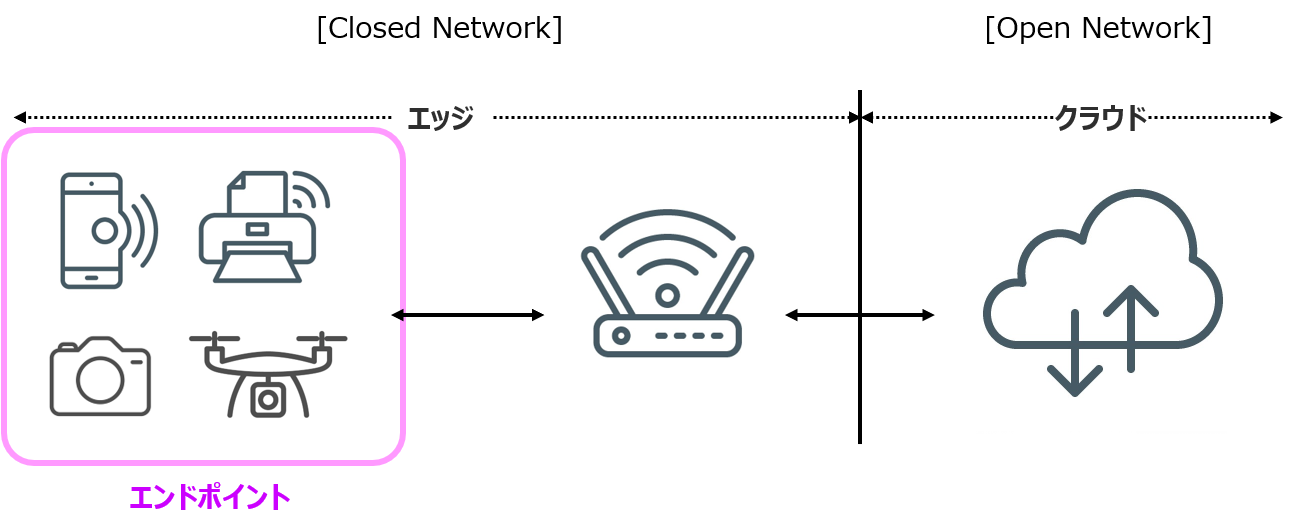
Areas of endpoint AI
Now, let's think about a system that analyzes what is reflected in the image projected by the camera, as before.
It is the same as edge AI in that only the results are shared to the cloud, but the biggest feature is that inference can be completed within the terminal instead of the repeater.
In other words, in the case of edge AI, it was necessary to exchange image data from the terminal to the repeater, but in the case of endpoint AI, only the inference results are already output from the terminal.
Since it is only inference results, the amount of data is overwhelmingly small, and it can be connected to a repeater with a general-purpose I/F such as I2C or UART.
If the system originally does not use a repeater, it is not necessary to install a new repeater itself.
The biggest feature of endpoint AI is that it can be expected to introduce AI while minimizing changes to existing systems.
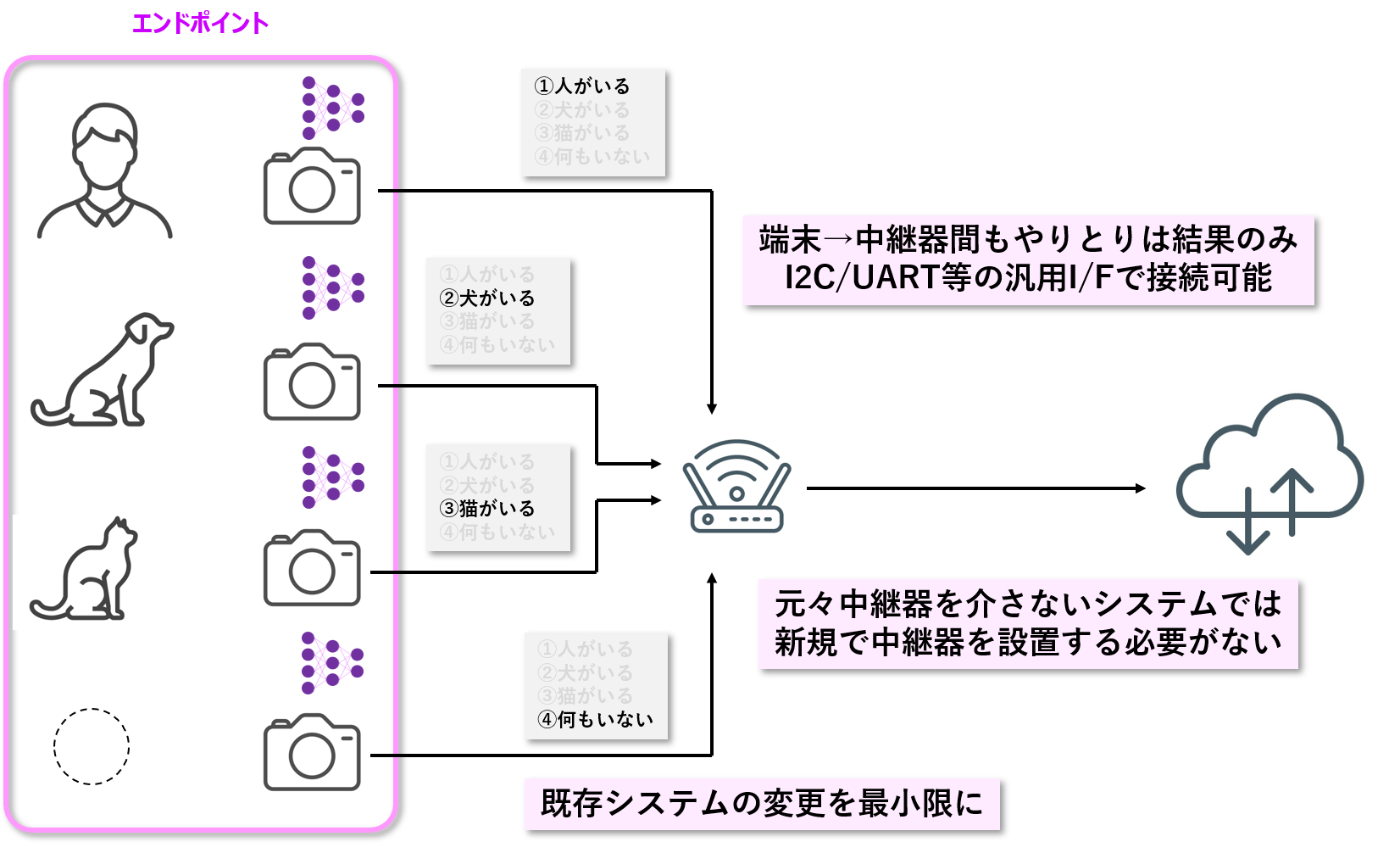
However, in order to realize endpoint AI, it is necessary to implement a chip capable of AI processing in the product terminal.
Terminals are limited by the size of their housings, and battery-powered products must reduce power consumption.
Also, cost will be a big factor.
Furthermore, when selecting small, low-power consumption devices for endpoints, AI processing accuracy is somewhat limited.
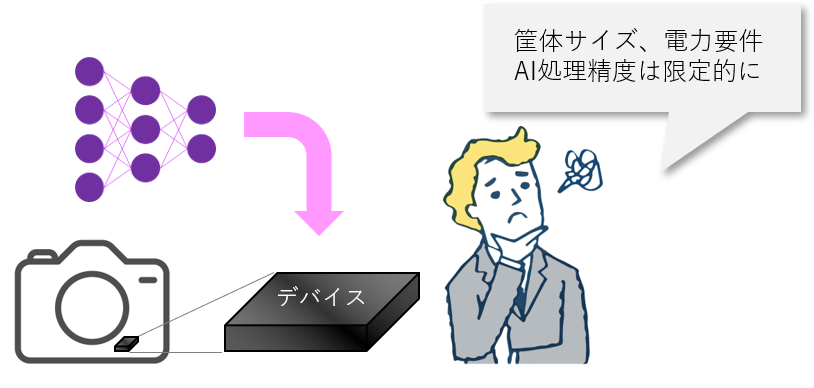
Considering these factors, where is it appropriate to have AI processing in the current system? should be considered.
Based on the above, the features of endpoint AI, edge AI, and cloud AI can be compared as follows.
Endpoint AI |
Edge AI |
Cloud AI |
|
System required for inference processing |
terminal only | terminal + repeater | Terminal + repeater + server |
network dependent |
no dependency | no dependency | Dependent |
real time processing |
Extremely low latency | low latency | high latency |
communication cost |
cheap | cheap | high |
privacy |
easy to secure | easy to secure | difficult to secure |
AI processing accuracy |
Limited | excellent | very good |
Implemented device features |
Small/low power consumption AI chip | High-spec GPU, etc. | No device implementation required |
3. Examples of Endpoint AI
Processing that requires high precision and high performance must be processed accurately at the edge (repeater) or in the cloud.
However, the resources that can be used to build the system are limited, so it seems more advantageous to leave the processing that is not necessarily required to the endpoint.
So what can endpoint AI do, and what is that AI chip like?
For example, if endpoint AI is implemented in a camera terminal,
"Detect hand gestures such as goo, scissors, par, OK"
"Personal authentication by recording the feature values of multiple people (about 5 to 10 people)"
"Recognize humans and objects and acquire their range coordinates"
etc. can be processed.
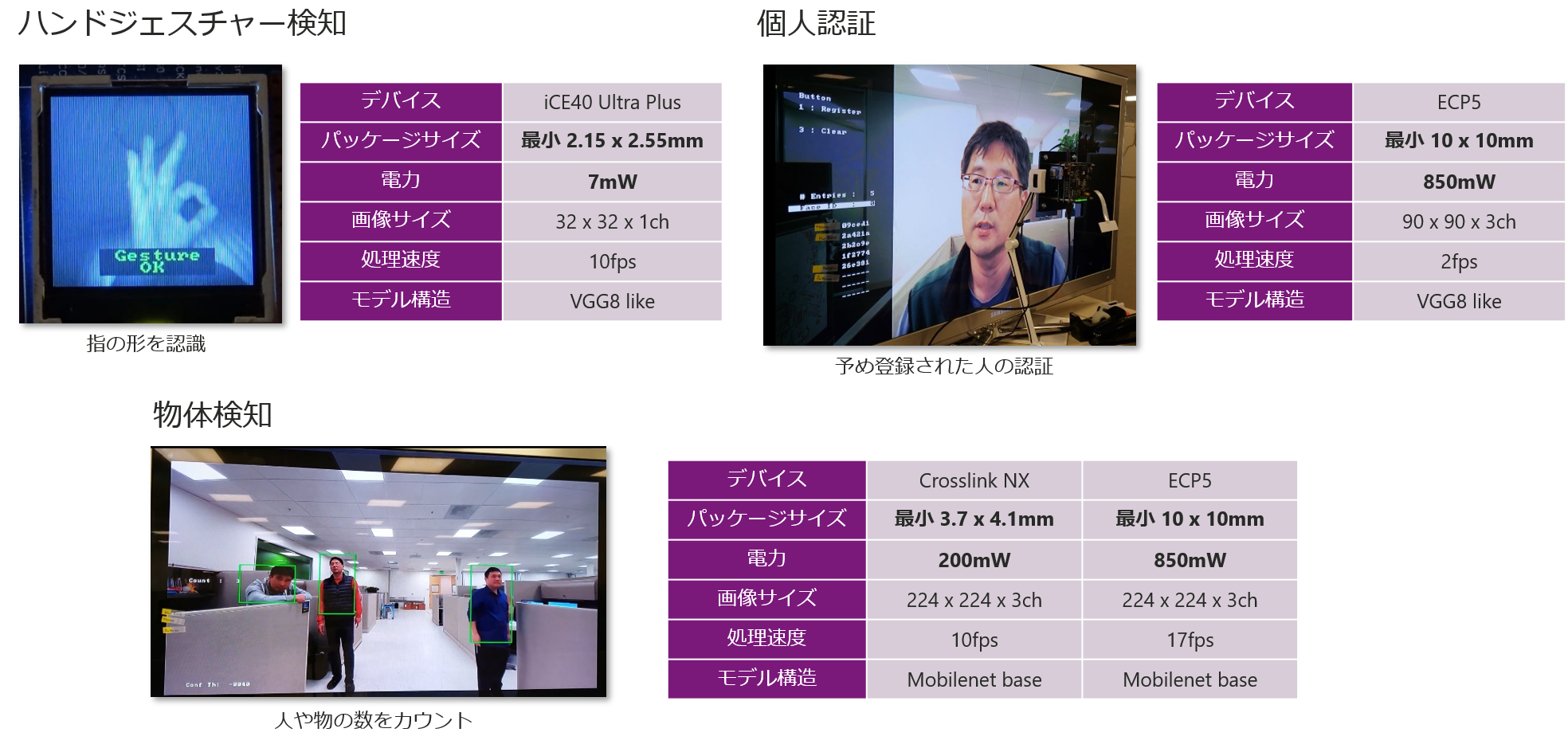
The example above uses an FPGA from Lattice Semiconductor.
Many of Lattice's FPGAs have strengths in small size and low power consumption, so they are suitable for endpoint AI.
Specifically, a trained model is mounted on a 2.15 x 2.55mm chip, and gesture detection is performed with 7mW of power.
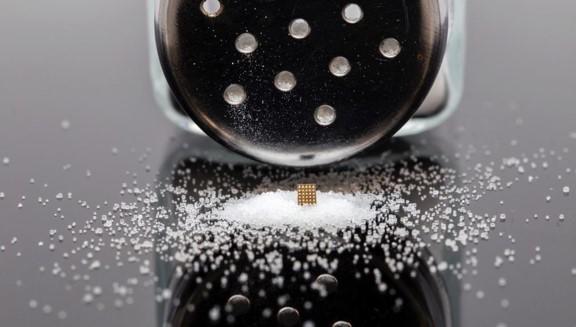
Lattice's FPGA iCE40UltraPlus is suitable for endpoint AI because it is small enough to be compared to a grain of salt
With this level of size and power, it may be possible to implement it on the product terminal side.
In addition to these AI processing, various other endpoint AI demo videos are posted on the link below.
All of them are when using Lattice's FPGA, but first, please check how much processing can be done with endpoint AI.
I want to consider introducing endpoint AI, but I can't allocate development resources...
Of course, when starting to consider introducing a system to a system, it is necessary to actually judge the performance, not just the video.
In particular, there are many new elements when considering the introduction of AI, and many people may be worried that it will not be easy to introduce it to their company.
Macnica has developed design support tools, and if you prepare an evaluation board, you can start evaluation immediately.
Specifically, it is possible to try AI processing using the demo design prepared by Lattice based on the image acquired in your own environment.
First of all, after checking the performance easily, it is possible to decide whether to start full-scale development.
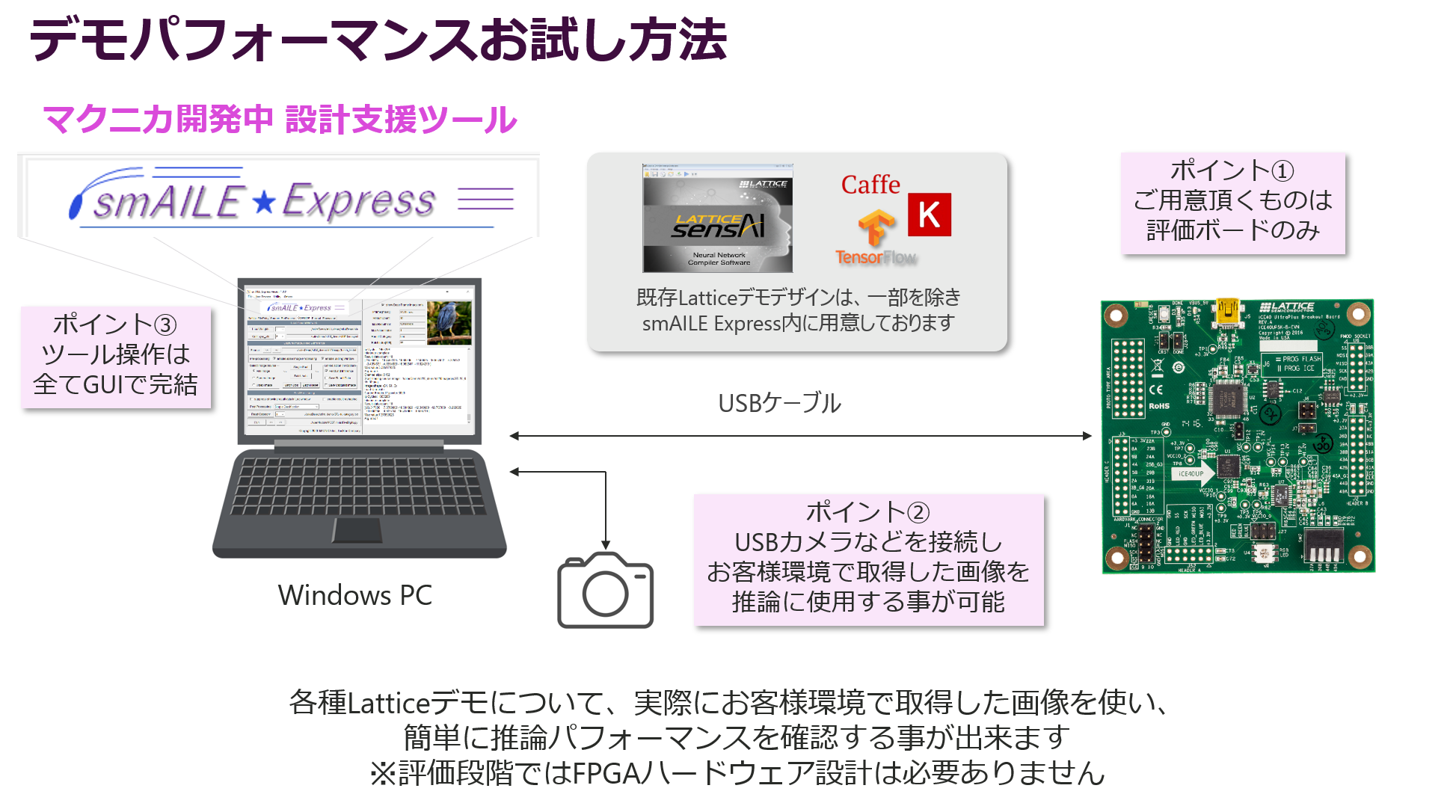