Application for evaluation machine
Making the manufacturing industry sustainable with Brain Tech x AI Part 2 - What is the AI learning method using brain waves?
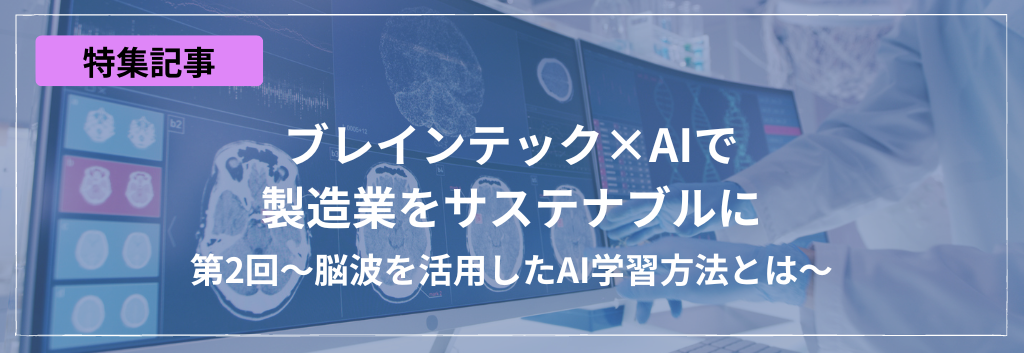
Digital Manufacturing using Braintech x AI
“Delicate human judgment” and “differences in skill level between individuals”
In the first installment of "Making the Manufacturing Industry Sustainable with Brain Tech x AI," we introduced the benefits of brainwaves x AI for Digital Manufacturing, but this second installment is based on actual cases that our company has worked on. Next, we will introduce the technical aspects of AI development that deals with brain waves.
This time we will focus on an example of AI model development aimed at automating defect inspection of screws in manufactured parts.
Initially, defects in parts were inspected at manufacturing sites through visual inspection by employees, but due to labor shortages and an aging workforce, the mission became to automate the inspection process, which had become dependent on the individual. I was there. (Click here for use cases in the manufacturing industry)
In recent years, abnormality detection at manufacturing sites has often been automated using AI image recognition models, but in defect inspections, `` ambiguous human judgment'' and `` differences in skill level between individuals'' occur. This was an issue.
Therefore, our solution adopted a method that utilizes brain wave data in the original AI image recognition model.
Advantages of label data using brain waves
hard label and soft label
Normal AI learning uses hard labels, which are judgments of 0 or 1, for training data.
Taking defect inspection as an example, set 0 for image data of a normal part,
1 is assigned as a label to image data of parts with defects.
This kind of clear black and white labeling is called a hard label, and is widely used in the original annotation.
However, in actual operations, there are cases where it is not clear whether a value is 0 or 1, or where the criteria for judgment differs depending on the person.
Therefore, we decided to use soft labels in this case.
In contrast to hard labels, labels that contain information between 0 and 1 that constantly fluctuate like brain waves are called soft labels.
For example, if the brain reacts strongly to an event that a person perceives, the brain waves will fluctuate greatly.
This allows the brain wave waveform at that time to be associated with the target event and given a label.
Furthermore, due to the characteristic that brain waves can vary in magnitude, it is possible to decipher how confident a person is about an event, so it is possible to treat ambiguous judgments between 0 and 1 as labels. It becomes.
In this case as well, we thought that using electroencephalogram data would be advantageous since it would be difficult to label the training data due to ambiguous human judgment when inspecting screw defects.
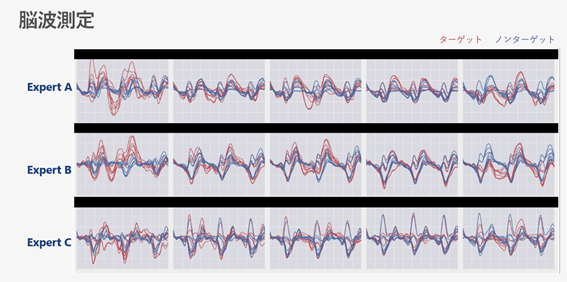
Soft label effect
In this example, we evaluated the accuracy of each AI model given three types of learning data: Ground Truth (GT), hard labels, and soft labels.
GT 's AI model is a model trained on data that was manually assigned labels as 0 or 1 by inspectors at the manufacturing site.
Next, the hard label AI model is a model that has been retrained using the labels obtained by converting the brain waves at the time of the test into 0 or 1 for the AI model trained with GT.
The soft label AI model is created by relearning the soft labels of the brain waves during the test using the AI model that was trained using GT.
When the AI model was trained under these conditions, the AI model trained using soft labels achieved an accuracy rate of 0.9 or higher, resulting in the highest accuracy.
In addition, it was found that GT 's AI model training was prone to overfitting, which is characteristic of AI models, and that soft label model training was more efficient due to the effect of label smoothing. .
Multiplication of comprehensive AI models
Panel data and ensemble learning
In this case study, we mentioned the difficulty of using an AI model to automate ambiguous human judgments, but there was an even greater difficulty in ``differences due to individual skills.''
Anomaly detection in the manufacturing industry is a task that is carried out by multiple employees rather than just one person, but it is inevitable that there will be differences in judgment depending on the level of veteran employees, such as years of experience, and individual sensory values. On the other hand, to train an AI model using supervised learning, label data is required.
Therefore, in this case study, we compared the model accuracy of two methods: AI model learning using panel data and ensemble learning.
These two methods are also used in regular AI model learning.
First, AI model learning using panel data is a method in which panel data, which is a weighted average of brain wave data obtained from multiple people, is trained as a single label.
Next, ensemble learning is a method that averages the scores of multiple models learned using brainwave data.
Each method is different, but the method is to average multiple labels and combine them into one.
Learning method suitable for each purpose
In this case study, we evaluated the accuracy of a total of three types: an individual model that trained labels using individual brainwave data, a model that utilized the aforementioned panel data, and a model that utilized ensemble learning.
As a result of the evaluation, the model using panel data had the smallest difference between the minimum and maximum scores, and was the most stable model.
On the other hand, the model that performed ensemble learning had the highest accuracy rate when using soft labels at 0.945, resulting in the best accuracy.
At first glance, if you only focus on accuracy, it seems best to incorporate ensemble learning, but how about thinking about it from the perspective of actual AI model operation?
In this case, ensemble learning has the highest maximum accuracy score, but panel data can also be seen as more versatile for a variety of data.
If both have relatively high accuracy, it is necessary to consider the learning method depending on which characteristic is more suitable for actual operation.
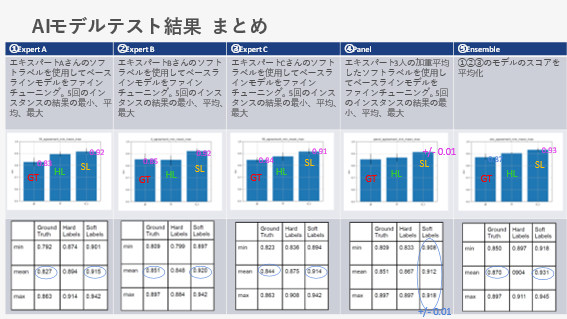
Benefits of using Braintech x AI in the manufacturing industry
Turn “subtle human judgment” into label data
As mentioned earlier, in the original AI learning, hard labels such as 0 or 1 were widely used, but in order to utilize "ambiguous human judgment" in learning, it is better to use brain wave data. It is suitable.
The reason for this is that soft labels are possible due to the constantly changing characteristics of EEG data, but also that ambiguous judgments can be faithfully converted into data by EEG measurement when adding labels during annotation. You can
Additionally, as mentioned earlier, using soft labels has the effect of regularizing the data, making it less likely that overfitting, which is a concern with normal AI models, will occur, which is another advantage of using EEG data.
Utilize “differences due to individual skills”
One of the challenges when using AI to automate decisions that were previously made by humans was that it became difficult to prepare highly accurate label data due to ``differences in individual skill.''
However, as in this case, if the learning method of the AI model can be devised, such as learning using panel data or ensemble learning, it can learn the judgments of various technicians in a balanced manner without biasing the judgments of a particular individual. can.
Summary
In this second installment of "Making the Manufacturing Industry Sustainable with Braintech x AI," we introduced a case study in which our company actually worked on a solution that automated defect inspection of screws, which are manufactured parts, using Braintech x AI. . There are various issues when using AI, but in this case we focused on the difficulty of AI model learning due to "ambiguous human judgment" and "differences due to individual skills." By devising learning methods such as using brain wave data as labels, we were able to achieve an accuracy rate of 0.9 or higher.
In recent years, the manufacturing industry has been facing issues such as the declining birthrate and aging population and the ability to pass on skills, but in addition to automation using AI, which has already been attracting attention, there is hope for solutions that utilize brain wave data.
As there are a variety of workplaces, it would be desirable for workplace work styles and the industry itself to change in a sustainable manner by advancing DX in the manufacturing industry in a way that suits the purpose.
free demonstration
Guidance of the demonstration
Would you like to actually measure brain waves?
What is the "skill transfer of experts" based on brain waves? What is AI that learns human consciousness and judgment?
I think there are a lot of unknown things, so why not try measuring your brain waves first and experience what it's like?
After the actual measurement, we will provide support from proposals to implementation and operation so that we can use AI using EEG at the customer's site while including examples of AI utilization in our activities so far. increase.
Learnmore
Document download
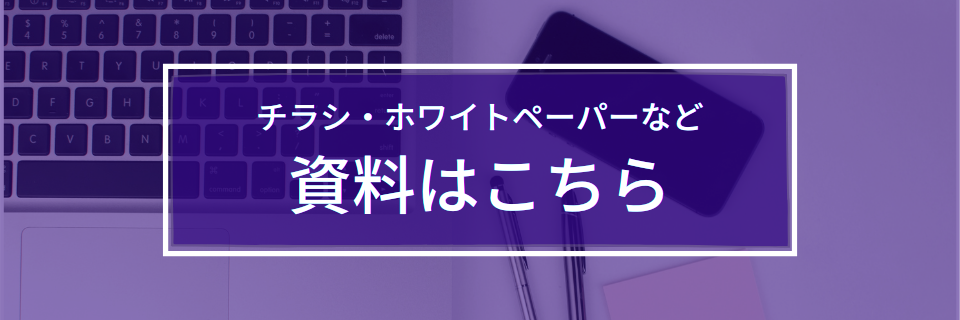
If you want to know the details, please download the materials from here.
Inquiry
If you have any questions regarding this article, please contact us below.