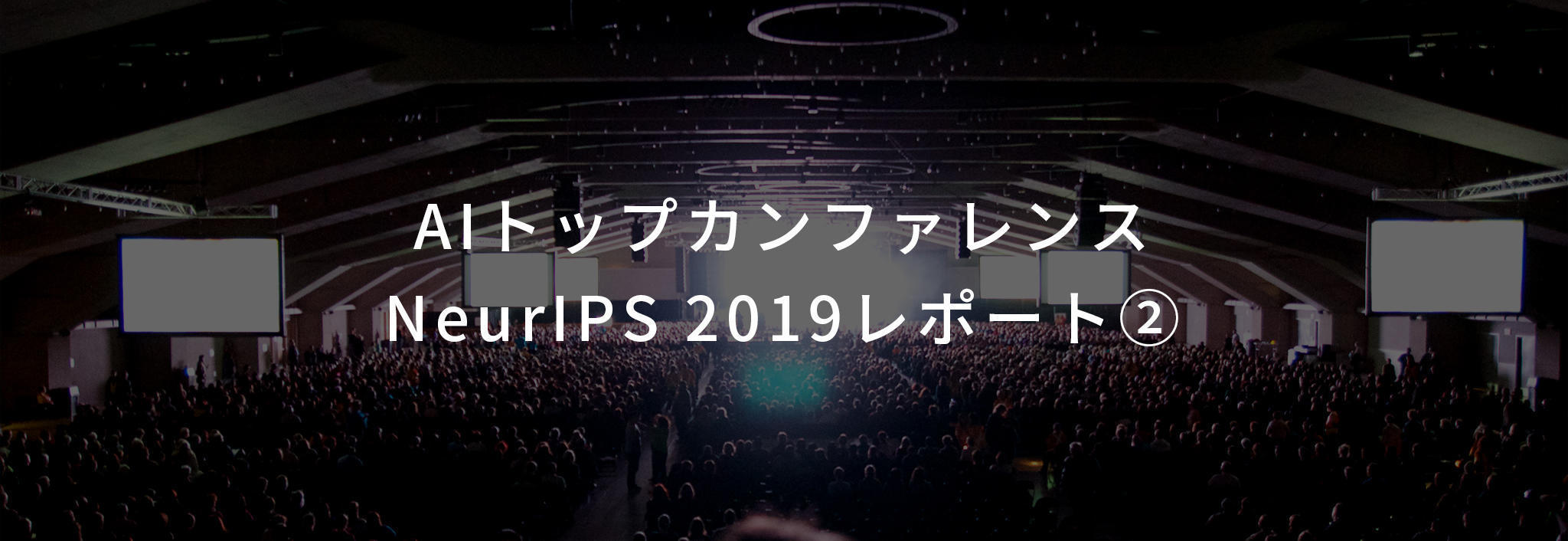
This article is recommended for those who
Data scientists, machine learning engineers, anyone studying artificial intelligence
Time needed to finish reading this article
10 minutes
Introduction
This column is a participation report of NeurIPS 2019.
Members of Macnica 's AI Research & Innovation Hub (ARIH) participated in NeurIPS 2019, which was held in Vancouver, Canada from December 8th to 14th, 2019.
In the first report,
・What is the position of NeurIPS among many AI societies?
・What are the AI technology trends seen from the submitted papers?
I told you. (If you haven't seen it yet, please check it out here!)
In the second report,
・AI technology trends seen from actual sessions
・ 5 AI Keywords that ARIH is interested in
I will tell you.
This year's AI technology trends as seen from NeurIPS 2019 sessions
NeurIPS consists of Posters, Workshops, etc., and we saw changes in each session.
First, I will introduce some of the contents of the workshop.
Workshop
We are shifting from mere theoretical research to full-scale research toward the practical application of machine learning to social issues such as public health, climate change, natural disasters, and crime prediction, as well as financial services and online marketing. I got the impression that there is a lot of research on privacy and fairness.
Healthcare
Fair machine learning in healthcare and machine learning to tackle climate change were attracting attention.
Especially in healthcare, the resources of social workers in public health are limited, and machine learning is being applied to optimize those resources to ensure fairness.
In India, machine learning using data for tuberculosis patients was used to check if they were taking their medicines properly, and if there were patients who forgot to take their medicines, an alert was thrown to save their lives.
He also touched on the biases inherent in machine learning models, and made a presentation on health risk judgments based on race.
privacy
Regarding privacy issues, it is necessary to delete a model created using personal information when an individual requests that it be deleted.
In that case, you will have to retrain all the data as this will affect the accuracy of the model.
However, considering the computational resources, it was not realistic, and a method using online learning was presented.
Human-in-the-loop (HIL)
NeurIPS has established a Diversity and Inclusion Committee to focus on diversity and the participation of people with disabilities.
Women in Machine Learning, Black in AI, Queer in AI, LatinX in AI, {Dis} Ability in AI, etc. were held.
Each group is working to expand the range of activities in machine learning.
As these activities become more active, the needs of individuals who want to be more active in society and companies who want to secure more resources will match, and it is expected that the world will continue to grow and contribute to society.
NeurIPS 2019 5 AI Keywords that Macnica was interested in
1. XAI (Explainable AI)
In particular, the content of neural network models such as deep learning is fundamentally difficult to interpret.
Therefore, the contents are often treated as a black Box.
However, as mentioned earlier, when applying it to the real world, there is a problem that a model that cannot be explained can not be used with confidence.
For example, in medical care, if machine learning determines that there is some disease, the problem arises that it is not possible to investigate the cause of "why such a judgment was made" unless AI can explain it.
This is considered a risk when applying machine learning in the real world.
For this reason, research to obtain the interpretability of black Box models has been active, and several papers have been published at this conference.
Customers in the manufacturing industry have a strong interest in the explainability of AI, so we are also paying attention to this trend.
[5 pick-up papers]
・A Benchmark for Interpretability Methods in Deep Neural Networks
・CXPlain: Causal Explanations for Model Interpretation under Uncertainty
・Deliberative Explanations: visualizing network insecurities
・Explanations can be manipulated and geometry is to blame
・Fooling Neural Network Interpretations via Adversarial Model Manipulation
2.Bayesian Deep Learning
Bayes considers the parameters themselves to be random variables that follow a certain probability distribution.
In other words, we infer important parameters for the model from the obtained data.
In deep learning, it is difficult to model uncertainty, and Bayesian Deep Learning (BDL) is a modeling method for understanding that uncertainty.
Workshops (http://bayesiandeeplearning.org/) are also being held, and I would like to keep an eye on this field as it is likely to become even more exciting in the future.
[5 pick-up papers]
・Practical Deep Learning with Bayesian Principles
・Gaussian Process Behavior in Wide Deep Neural Networks
・On estimating epistemic uncertainty
・Using Loss Surface Geometry for Scalable Bayesian Deep Learning
・Uncertainty under distributional shift
3. Few-shot or Zero-shot Learning
One of the trends is how to learn well with a small amount of data.
Besides Few-shot and Zero-shot, there were One-shot and so on.
Essentially, it is important to acquire and accumulate high-quality data, and to maintain the machine learning model by retraining it periodically, but I think there are realistic cases where data cannot be collected. Such research is also interesting.
[5 pick-up papers]
・Zero-shot Learning via Simultaneous Generating and Learning
・Zero-Shot Semantic Segmentation
・Cross Attention Network for Few-shot Classification
・Adaptive Cross-Modal Few-shot Learning
・Few-shot Video-to-Video Synthesis
4.Federated Learning
It is a mechanism to update a common model using distributed data.
Learning is performed on the edge device side and differences are reflected in the common model on the cloud, so even if you do not want to upload data to the cloud, you can handle it.
In addition, it is possible to create a model suitable for the edge environment and tune a common model.
It also helps protect privacy.
It seems that they are simultaneously developing technology to compress and encrypt the update part so that it fits the realistic environment where the upstream line is slow.
The edge computing market is expected to grow in the future, so it is likely that user convenience will continue to improve as affinity with such technologies increases.
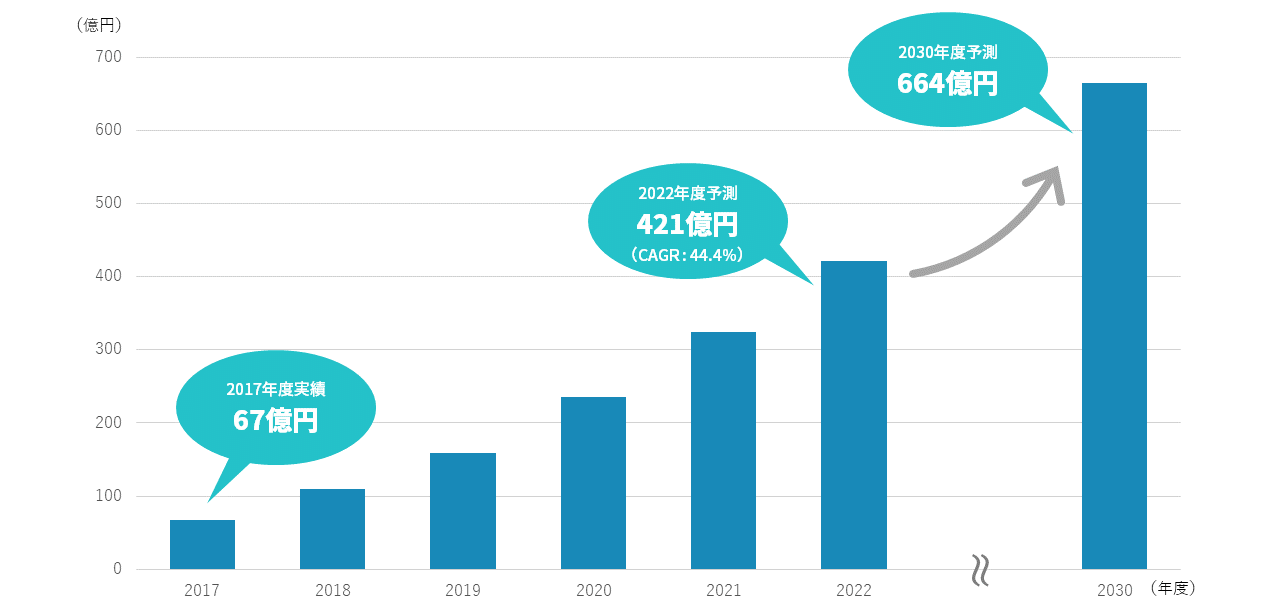
Source: Created by Macnica based on Fuji Chimera Research Institute Co., Ltd. “2019 Artificial Intelligence Business Comprehensive Survey” p.6 2. Edge computing market size
5.Privacy Protection
When machine learning enters the stage of social demonstration, the focus will be on the handling of data used for learning.
In particular, the handling of personal information is extremely sensitive, and research has been actively conducted on how to apply it to machine learning while ensuring privacy.
We want a world where users can receive the benefits of machine learning with peace of mind.
[5 pick-up papers]
・Differentially Private Algorithms for Learning Mixtures of Separated Gaussians
・Locally Private Gaussian Estimation
・Differentially Private Bayesian Linear Regression
・Differentially Private Markov Chain Monte Carlo
・Differentially private anonymized histograms
Summary
NeurIPS2019 will be streamed live on Slides Live, and even after the conference is over, anyone can watch it for free.
https://slideslive.com/t/neurips-2019
You can also check the list of accepted papers and related posters here.
https://nips.cc/Conferences/2019/Schedule?type=Poster
In 2019, I participated in AI international conferences such as NeurIPS, ICML, and IJCAI.
I was able to feel the remarkable progress of AI and the steady shift from the world of research to the world of practical use.
ARIH would like to contribute to this evolution of AI as much as possible, and will be presenting at the industrial session as a sponsor at IJCAI scheduled to be held in January 2021. Please join us.