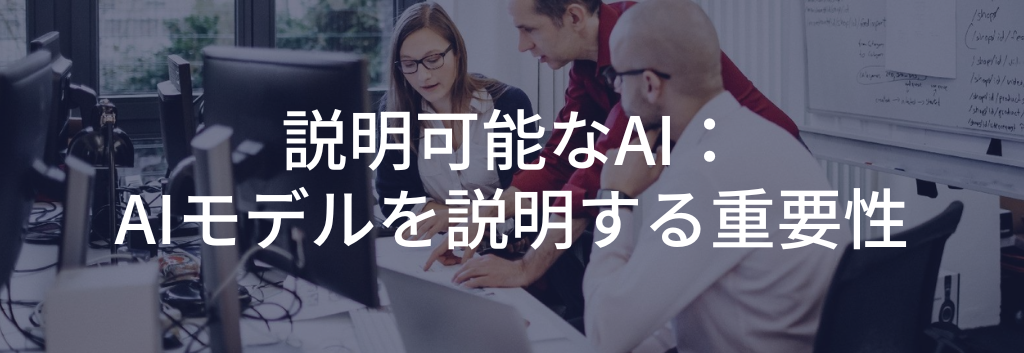
As artificial intelligence solutions proliferate, AI developers and users have introduced various words and expressions to describe them and name their features. You've probably heard new terms like "specialized AI," "deep learning," and "neural networks," used to distinguish between different types of AI, their capabilities, and elements of AI solutions. Also, as a result of the exponential growth in available AI and its widespread use, there is a need for what is called “explainable AI”. In other words, there is a need for methods and technologies that make AI easier for everyone to understand.
So why do we need “explainable AI”? The biggest reason is that the spread of AI solutions has made AI widely used even by workers with only general knowledge of data science and artificial intelligence. Previously, AI solutions were available only to those with technical knowledge. Practical AI is now available to recruiters, salespeople, visual artists, and many other jobs, and it is being used outside of work as well. Whether taken personally by a floor manager or downloaded directly to a mobile phone, many solutions are available out of the box.
AI is now influencing our lives, from the small things to the big ones. AI could save a human from time-consuming repetitive tasks, or it could make a life-saving diagnosis for you at your next appointment. These two very different examples are the most often cited rationale for making AI explainable.
- People want to know how AI works. By doing so, you can use AI with peace of mind, or you can customize it. Plus, you'll be able to choose from a number of solutions that are right for you, and you'll be able to tailor them to your needs.
- Ethically people have a right to know how AI works. We are entrusted with decision-making in medicine, law, finance, and other areas where ethical boundaries are of paramount importance to many.
Being explainable also unlocks the part of AI often called the “black Box” and helps us discuss solutions in language most people can understand. You'll also be able to answer common and important questions like, "How was this important decision made?" and "Are there other possible options?"
CrowdANALYTIX Companies like , often need to work on explainable AI. For example, recently a very practical ensemble model developed. It will define the selection parameters for the Phase 3 trial based on the results of the previous Phase 2 trial. By the way, at first random forest was developed on the basis of The resulting algorithm resulted in a Phase 3 trial with 20% fewer patients than the traditional statistically-based approach. This would have saved the pharmaceutical companies millions of dollars.
However, I ran into a problem when trying to explain how the model I ran led to this result. Random forest models are accurate, but individual trees are often entangled, with their loads adjusted by algorithms to maximize accuracy. It's a good technique, but it's too complex to be easily explained by humans. Unless it's more descriptive, pharmaceutical companies won't get the FDA approval they need to conduct tests with the Company proprietary method.
Being well explainable was key to getting the solution approved. To address this, we designed a similar working model using a decision tree approach, rather than the random forest used in our solution. This version produces similar results, but is much easier to explain and understand for people outside the data science community. Although some of the accuracy of the original model had to be compromised, the compromise was worth it because of the transparency of traditional and ethically stringent FDA standards. Efforts to make AI more acceptable in the pharmaceutical and healthcare sectors are a step in the right direction.
It's clear that "explainable AI" is considered the future of artificial intelligence by some of the world's biggest companies. Google has invested heavily in this area to provide interactive visualization tools for those who want to understand how machine learning works. In addition, investors are quick to sense the growing demand for AI that can decompose information to a level that ordinary people can understand. Ordinary people will also seek such knowledge when it comes to incorporating solutions into their work and lives and entrusting them to them.
As humans continue to innovate and improve, we can move closer to the goal of transforming explainable AI into impactful AI capable of performing optimal tasks. Understanding when and why AI successes and failures will allow us to allocate resources more strategically and achieve greater efficiencies at every level.
Now is the time for data scientists to acquire the skills of “explainable AI” and start appealing to consumers who want to utilize the solution and “understand the tool of AI”.
▼Contact us
https://go.macnica.co.jp/CAX-Inquiry-Form.html
▼ Business problem-solving AI service that utilizes the data science resources of 25,000 people worldwide
Click here for details
Source:https://www.crowdanalytix.com/explainable-ai/
CrowdANALYTIX Resource Library