Specializing in appearance inspection, enabling rapid and secure manufacturing site DX using edge AI -Learning with only a small amount of normal data, all AI operations completed on edge devices-
Specializing in visual inspections for the manufacturing industry, it contributes to rapid labor saving and security risk reduction at manufacturing sites where labor shortages are being called out.
Points of the "anomaly detection model"
•Learning completed with normal data only
- Since annotation work, which is often outsourced during learning, is no longer necessary, there is no need to conclude a non-disclosure agreement with customers.
In addition, there is no need to prepare a large amount of defective product data, and learning is completed in a few seconds, making it possible to quickly introduce AI to the site.
•Both learning and inference are completed on FPGA-equipped edge devices
- Since there is no need to send image data outside the company, the risk of information leakage is reduced, and complicated non-disclosure agreements are not required, leading to labor savings.
- Can be used in places without a network environment.
• Re-learning is possible on-site without an AI engineer
- Easy-to-understand visualization of anomalies with a heat map. AI learning results can be saved and reloaded,
It can be easily redone on site, and can be used by companies that have difficulty securing IT personnel.
IT人材の不足や設備にかかるコストを低減
Due to chronic labor shortages, the need for automation is increasing at manufacturing sites. The use of AI is attracting attention for appearance inspections such as product inspections, but there are issues such as the lack of IT personnel and the high cost of equipment. In addition to the difficulty of collecting the defective product data necessary for AI learning, the diversification of consumer needs has led to demands for high-mix low-volume production. It is also assumed that learning will require the support of an AI engineer. The effort required to deal with these issues is also an introduction hurdle.
LeapMind has been developing AI inference accelerator IP "Efficiera®" that can incorporate deep learning functions into various edge devices in order to popularize devices using deep learning and make people's lives more convenient. I was. The recently developed "Efficiera anomaly detection model" utilizes our unique technology "minimum quantization technology" to realize highly secure and easy-to-operate AI visual inspection that is suitable for practical use in the field. In addition to completing both learning and inference on a small edge device equipped with FPGA, the two features of completing learning only with normal data have given various advantages. Compared to using cloud AI, the images to be inspected do not need to be taken out of the device, reducing the risk of information leaks when sending images outside the company, as well as reducing broadband network and cloud service usage fees. contribute. In addition, compared to using an on-premise GPU server, it also solves concerns about scalability due to equipment costs and installation space.
Furthermore, learning can be completed in seconds using only dozens of normal data, eliminating the need for large amounts of defective data. Since it can learn only with normal data, it eliminates the need for annotation*¹ work, reduces the risk of only being able to detect defects that have been learned, and makes it possible to detect unexpected abnormalities. The re-learning operation is also simple, and the heat map visualizes the areas judged to be abnormal by the AI, so adjustments can be easily made on-site without the support of an AI engineer, even on a high-mix low-volume production line. Training results can be saved in a few megabytes of data and used for inference later, so it is easy to redo training mistakes. Even companies that find it difficult to secure IT human resources and companies that have manufacturing bases in rural areas where broadband networks cannot be used will achieve smooth AI practical application and support DX in the manufacturing industry.
*¹ Appending information tags to data. In many cases, the work is outsourced, which can pose a risk of information leakage.
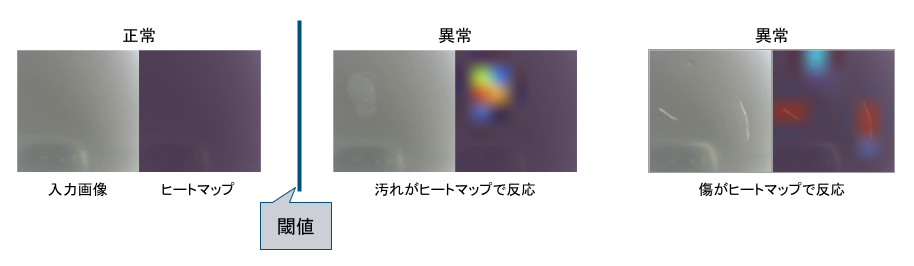
Inquiry
If you have any questions regarding this article, please contact us below.