product
service
- Simple Security Consulting [Consulting]
- Splunk SOAR Automation Assessment Service [Consulting]
- Dashboard/SPL Creation Pack [Implementation/Building Support]
- Version upgrade service [implementation and construction support]
- Splunk Premium Apps construction support service [implementation and construction support]
- Splunk Security Log Analysis Start Package [Original App/Service]
- Splunk × CrowdStrike Falcon Insight, Macnica Original App [Original App/Service]
- Government uniform standard compatible App [Original App/Service]
- Smart Security Monitoring App [Original App/Service]
- Splunk × LANSCOPE Original App [Original App/Service]
- Security Monitoring App for Box [Original App/Service]
- Cloud Security Monitoring App [Original App/Service]
- SIEM Operation Monitoring Service [Original App/Service]
- List of services
- Macnica Premium Support for Splunk (utilization support, version upgrade monitoring)
- Macnica Premium Support for Splunk Skill Up Package
Specifications/Technical Information
Application for evaluation machine
- FAQ
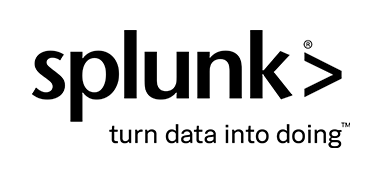
Splunk
Splunk
Behavior detection product "SplunkUBA" by machine learning
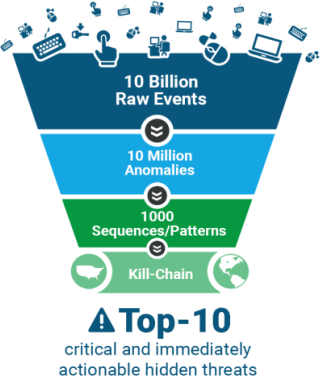
Splunk User Behavior Analytics (hereafter, Splunk UBA) is an out-of-the-Box (ready-to-use) solution that detects unknown threats and anomalous behaviors through machine learning.
Splunk UBA generates a baseline from the input data using machine learning, detects anomalies based on the baseline, performs further machine learning on the generated anomalies, and detects threats. increase.
It also provides visibility into unknown threats and multiple entities (users, devices, apps) related to threats to understand the overall security story. If it is determined to be a threat, Splunk products will conduct more detailed investigation and countermeasures.
Are you having trouble with these issues? : Internal fraud
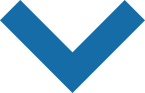
Identify individuals that are difficult to detect on a threshold basis!
Are you having trouble with these issues? : Countermeasures against targeted attacks
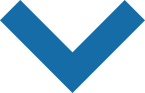
An attempt to log in to an important internal server using a hijacked account has been detected!
screen image
TOP screen
Threat details screen
feature
-
Multiple analysis with anomalies and threats
Exposing anomalies in behavior using machine learning, and extracting threats from those anomalies
In the case of anomaly detection alone, a large amount of anomalies are detected, but by multiplexing machine learning, it is possible to extract highly accurate threat information.
By detecting threats that operators really need to see and receiving advice on countermeasures, operators can avoid alert fatigue (Alert Fatigue/Alarm Fatigue).Alerts for anomalies and scoring only
Too many alerts obscure real threats, what to look for
Also, if you do not know what is detected, you do not know how to deal with itSplunk UBA scores anomalies and threats separately, so the real threat is clear.
In addition, there is an explanation of what the threat is and how to deal with it.
This reduces the burden on operators after detection. -
Multi-entity machine learning model
Detect anomalies and threats that combine not only user behavior but also device and application behavior - unsupervised machine learning
Detect anomalies/threats based on historical data without preparing correct information - User-friendly interface
Detailed descriptions of threats and anomalies are available, allowing for quick decisions on next actions - Seamless integration with Splunk products
Functional affinity such as data collection using Splunk and feedback of detection events to ES - Real-time/big data platform
Scalability through scale-out
Configuration image
Inquiry/Document request
In charge of Macnica Splunk Co., Ltd.
- TEL:045-476-2010
- E-mail:splunk-sales@macnica.co.jp
Mon-Fri 8:45-17:30