Why UEBA is a critical component of incident response
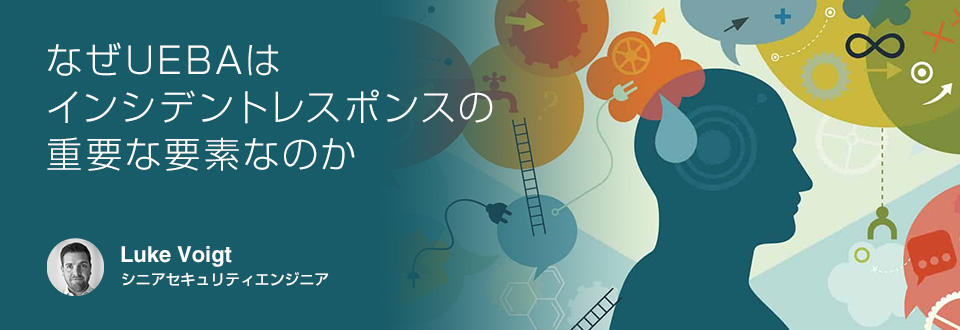
Gartner 2018 report, Market Guide for User and Entity Behavior Analytics. According to (Market Guide to User and Entity Behavior Analysis), in the world of cybersecurity, security teams tend to move away from a preventative-only approach. Security teams to prevent cyber threats and new detections and Incident response As part of our efforts to improve the effectiveness of our security systems, as we move toward compatibility with (IR) approaches, UEBA (User and Entity Behavior Analytics) and other technologies are being incorporated into traditional SIEMs and other traditional prevention systems.
Evolution of UEBA
As a basic definition, UEBA is the creation of a baseline of user and entity behavioral activity that is combined with peer group analysis to search for and analyze anomalous activity to identify potential or actual It detects intrusions and malicious behavior that has occurred. Evolving from fact-based security and simple correlation rules, UEBA leverages behavior-based analysis of both users and entities to model threats based on individual user behavior.
Gartner renamed “User Behavior” Analytics (UBA) a few years ago to create the acronym UEBA. The "E" is added to emphasize the importance of "entity behavior" such as cloud applications and unattended endpoints, not just user behavior. According to Gartner, the E is "due to the recognition that entities other than users are often profiled to more accurately identify threats, in part due to the behavior of entities other than users. by correlating with
Why UEBA is better than UBA and traditional SIEM
Compared to UBA and traditional SIEM systems, UEBA is significantly better for several reasons. First, UEBA overcomes the limitations of SIEM correlation rules and addresses the fact that in many cases the entire correlation rules model is broken. If you rely on SIEM correlation rules, you have the following problems.
- Inability to discover attacks due to lack of context in rules, or missing unknown incidents, causing false negatives.
- ルールにメンテナンス工数がかかりすぎる。
- Improperly filtered rules can delay incident response execution, as administrators must filter the rules they apply to determine which data is relevant and which is not relevant to the context of the event. be.
UEBA also reduces false positives, which helps combat alert fatigue. Additionally, teams can prioritize alerts so security professionals can focus on the most credible, high-risk alerts.
Why UEBA should be part of any organization's security framework
As we all know, cyberattacks are becoming more complex and harder to detect. Writing correlation rules for thousands of possible scenarios is no longer realistic. This is especially true in the case of insider fraud. If you have established a rule to "send an alert whenever a user sends an attachment larger than 4MB", you should consider every user individually and set exceptions. For example, a graphic designer in the marketing department may send out large PDF files on a regular basis. Thus, rather than relying on security experts to manually create a whitelist for every case, UEBA replaces traditional true-false alerts with probabilistic models and risk factors based on advanced analytics. increase.
In this way, UEBA achieves better internal fraud detection than traditional SIEM correlation rules. Additionally, UEBA tracks anomalous user behavior and suspicious lateral movement. It can be associated not only within an organization or network, but also with cloud services, machines, mobile devices and IoT assets. User behavior analytics also saves teams a lot of time by not having to dig through logs in different places to piece together a story about an incident. Sophisticated UEBA systems ingest data from a variety of different log sources, including Windows AD, VPNs, databases, badges, files, proxies, and endpoints to create contextual stories around incidents that security teams can make it analyzable.
Below are just a few of the many benefits of UEBA.
- Combining various risk information to create a final score and rank risks
- Enable prioritization and effective response
- 自動インシデントレスポンス機能により、チームがセキュリティインシデントにすばやく、かつ少ない工数で対応できるようにする
Replacing True/False Alerts with Models and Calculations
The model offers even more benefits for teams using UEBA. Probability models are more effective than True/False alerts. Data science can also be used to combine multiple pieces of evidence across different datasets to define the likelihood that a user account has been compromised or is fraudulent.
Advanced UEBA allows modeling of:
- Normal process that triggers authentication (to catch abnormal processes)
- The day and time the user performed account creation and whether it is normal for this user to create an account compared to peers
- Ports and IP addresses that certain devices connect to regularly
- The user executing the PowerShell operation and the content of the operation
- Subnet for each user that communicates with the Internet
- Which users traverse which subnets from specific critical assets
Evolution from static threshold-based risk assessment
There is a growing realization that static threshold-based risk assessments are no longer effective against today's sophisticated attackers and tech-savvy insiders.
With UEBA, you can counter sophisticated threats with sophisticated detection capabilities, such as risk score adjustments based on peer group-based metrics. For example, UEBA can show the anomalous behavior of one accountant compared to the behavior of a peer group of accountants. UEBA uses models and matching to consider not just one, but many different anomalous behaviors that differ from peer groups to indicate threats. Anomalous behavior could be a specific accountant accessing a subnet that is not normally used, such as the human resources department.
Consider the case of a user coming from Barbados for the first time via VPN. Just because an entity behaves abnormally doesn't mean it's dishonest. It is also possible that the user is traveling. However, UEBA conducts user analytics based on relevant behavior. For example, the risk score rises dramatically when the same user roams across multiple subnets.
In the case of APT, traditional systems may make hackers with compromised credentials look like ordinary employees in HR. However, when that user suddenly accesses the industrial control subnet, UEBA recognizes that user's behavior as abnormal.
Figure 1: UEBA showing notable anomalous behavioral events and
Data analysis information that shows various models on which users' normal behavior is based
Finally, machine learning in UEBA systems such as Exabeam helps in infer the context of potential alerting events with greater accuracy in all the aforementioned scenarios, adjusting the risk score to reduce the false positive rate. can be suppressed.
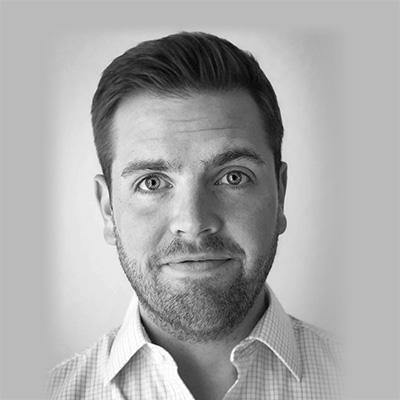
Luke Voigt
Exabeam, Inc. Senior Security Engineer
video on demand
The threat is in full view! Realizing effective log analysis with machine learning
~What is Exabeam, the next-Next-Gen SIEM Platform Exabeam?~
As targeted attacks and internal fraud continue to increase in recent years, an increasing number of companies are building mechanisms (such as SIEM) to correlate and analyze logs from multiple security products in order to implement appropriate security operations. . This is because it is difficult to visualize the impact of each incident using only the logs of security products that have already been installed, and threats may be overlooked. However, building such a system requires security-related knowledge, analytical know-how, and ideas. In this seminar, we will introduce “Exabeam” which realizes log analysis by UEBA (User Entity Behavior Analytics) technology and efficiency of conventional SIEM operation.