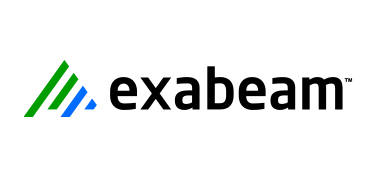
Exabeam
Exabeam
UEBA and machine learning - 5 advantages -
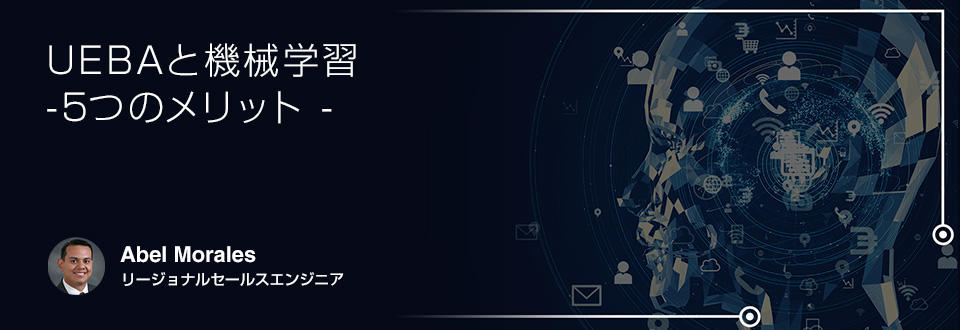
Organizations must tackle cybersecurity threats with an in-depth security approach. This approach leverages layered security that provides prevention, detection, and response capabilities. However, organizations invest a lot of time and resources in preventive controls. Sophisticated attackers are prepared to evade defenses by blending into the environment as regular users and then laterally moving across the enterprise from there. The challenge with hierarchical security is that each control point generates hundreds or even thousands of logs per second. The abundance of noise and false positives makes it difficult for Security Operations Centers to detect attackers in the network.
What is UEBA
Gartner defines UEBA (User and Entity Behavior Analytics) as a solution that analytically builds standard profiles and standard behaviors of users and entities across time and peer groups. Activity that is unusual by these standard baselines is presented as suspicious. Packaged analytics are applied to these anomalies to help uncover threats and potential incidents.
UEBA solutions build baselines of user and entity profiles to identify normal activity. The solution also leverages machine learning (ML) to create descriptive and predictive models. Descriptive models look to the past and answer the question, "What happened?" Predictive models predict the future and answer the question "what could happen?" Machine learning is a key component of UEBA that automatically builds models, learns from historical data, and identifies deviations from normal behavior. Deep learning platforms can be used to take advantage of a more advanced form of machine learning called deep learning (DL). This will allow UEBA models to run more efficiently.
As a former incident responder, I can recall several incident response cases where I had to manually analyze and draw conclusions from users' normal behavior. For example, if a user simultaneously establishes two VPN sessions from two different locations and accesses multiple servers during those sessions, we manually analyze the datasets going back three to six months. Depending on the analyst's experience, each analyst will often come to different conclusions because the experience is manual.
Leveraging machine learning, UEBA can help you understand how each user (human and service account) and entity (machine) typically behaves in your environment. The UEBA platform prioritizes users and entities in an environment in descending order of risk, maximizing analyst time. The challenge with traditional SIEMs is the large number of false positives generated by static correlation rules and the one-sided analysis direction. The difference with the introduction of UEBA tools is that the detection engine of the platform is multifaceted. That is, aggregate anomalies per user or entity when that user or entity deviates from normal behavior. When a user or entity's value exceeds a threshold set by your organization, analysts are notified for priority attention. The ability to prioritize users and entities addresses CISO and SOC managers' concerns about alert fatigue. Alert fatigue is the phenomenon in which analysts are blinded by a flood of alerts and miss important alerts.
In early deployments, legitimate user activity may be flagged as anomalous. This often happens during the early learning stages, and analysts can tag this activity as normal behavior. The UEBA system's machine learning then aggregates that data to reduce similar false positives.
Accurate user behavioral data combined with machine learning allows analysts to more accurately monitor users and entities while gaining deeper visibility into their respective activity. Here are some examples that demonstrate the power of UEBA.
- Abnormal Data Downloads: A user downloads up to 100MB of data each day. One day, this user suddenly downloaded several gigabytes of data. The system detects this anomaly and adds points to the user's profile.
- Credential theft: An attacker compromised an employee's username and password and attempted to use this credential to gain access to an executive's system. However, this attacker's behavior deviates from the normal behavior of credential holders. For example, if compromised credentials are routinely used in a particular region, at a particular time of day, and on a particular machine, the attacker's behavior has deviated throughout the attack chain. The attacker attempts lateral movement within the environment, and this activity is also detected by the UEBA platform.
- Abnormal Transactions: A financial institution's UEBA system can detect bank employee fraudulent situations in which large amounts of money transfers are initiated and approved. The system recognizes that the bank employee's (insider's) normal trading patterns differ from the norm for normal behavior and flags this activity for management investigation.
- APT (Advanced Persistent Threat): APT attacks are carried out by groups of highly skilled hackers. It targets websites and moves through the organization carefully evading detection for several months after the intrusion (lateral movement). Each of these steps may evade conventional detection techniques, but when combined they provide an anomalous picture. UEBA solutions can identify anomalous behavior of some users or entities within a group and alert analysts as coordinated malicious activity.
Benefits of UEBA and Machine Learning
Using machine learning and UEBA will allow us to learn about behavior and integrate it into our detection engine. This significantly reduces the time analysts spend writing and modifying complex correlation rules. Correlation rules are static, so you need to repeat the same rule multiple times to cover all possible scenarios. This also causes a large number of false positives. UEBA dynamically adapts to its environment and can detect subtle changes in behavior. This is difficult with static correlation rules. The dynamic nature and detection capabilities of UEBA benefit enterprise cybersecurity in a number of ways, including:
- Detect breaches of protected data: When you have protected data, it's not enough to just keep it safe. When a user accesses this data without a legitimate business reason, you need to know. The UEBA system will detect this situation and send an alert if it occurs.
- Internal Fraud Detection: Employees can be fraudulent and use their access rights to steal data and information. UEBA helps detect data breaches, sabotage, privilege abuse and policy violations by employees. For example, if an attacker compromises a system administrator's credentials, the attacker can move data within the environment. This includes offline storage (OST) files, documents, presentations, etc., including confidential and proprietary data. In years of experience in Security Operations Centers, out of 100+ alerts, only 1 or 2 are true positive DLP incidents. UEBA reduces the number of false positives and helps identify true insider threats.
- Flag for privilege modification and privileged user creation: Some attacks take advantage of privileged users. UEBA will send an alert if a privileged user is created or if there are accounts with unnecessary privileges. According to the MITER ATT&CK framework, one of the Tactics, Techniques, and Procedures (TTPs) used by attackers is persistence through a technique called Create Accounts (T1136). UEBA helps identify anomalous account creation based on user criteria. For example, a system administrator's account routinely creates accounts between 9:00 a.m. and 6:00 p.m. US Eastern Standard Time. If an attacker compromises this administrator account and begins creating accounts outside of these hours, UEBA tools will identify this activity. UEBA tools can also identify anomalies based on other factors such as privileges granted, the system used to grant privileges, and the system's network zone.
- Brute Force Attack Detection: Cyberattacks can target cloud-based entities and third-party authentication systems. UEBA can be used to detect brute force attacks and block access to such entities. For an organization that constantly monitors failed logins, it takes less than a day to review all 200 accounts that generate failed logins and identify potentially malicious accounts. UEBA gives higher priority to accounts that generate an unusual number of login failures based on account profiles, providing contextual information to aid decision making.
- Reduce false positives: The UEBA system is constantly learning to improve accuracy and avoid false alarms. This approach reduces false positives by not alerting analysts unless there are multiple anomalies. Machine learning and UEBA prevent you from receiving a flood of false positive alerts.
Summary
UEBA enhances security by using machine learning and algorithms to monitor users and other entities to detect anomalies in behavioral patterns that may indicate threats. By taking a more proactive approach to security and gaining greater visibility into user and entity behavior, you can strengthen your security posture and more effectively mitigate threats and prevent breaches.
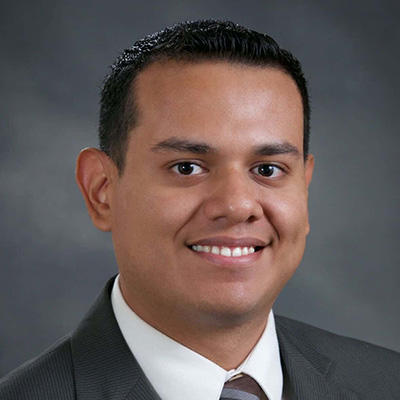
Abel Morales
Exabeam, Inc. Regional Sales Engineer
video on demand
The threat is in full view! Realizing effective log analysis with machine learning
~What is Exabeam, the next-Next-Gen SIEM Platform Exabeam?~
As targeted attacks and internal fraud continue to increase in recent years, an increasing number of companies are building mechanisms (such as SIEM) to correlate and analyze logs from multiple security products in order to implement appropriate security operations. . This is because it is difficult to visualize the impact of each incident using only the logs of security products that have already been installed, and threats may be overlooked. However, building such a system requires security-related knowledge, analytical know-how, and ideas. In this seminar, we will introduce “Exabeam” which realizes log analysis by UEBA (User Entity Behavior Analytics) technology and efficiency of conventional SIEM operation.
Inquiry/Document request
In charge of Macnica Exabeam Co., Ltd.
- TEL:045-476-2010
- E-mail:exabeam-sales@macnica.co.jp
Weekdays: 9:00-17:00