Responding to Insider Fraud Using Data Science
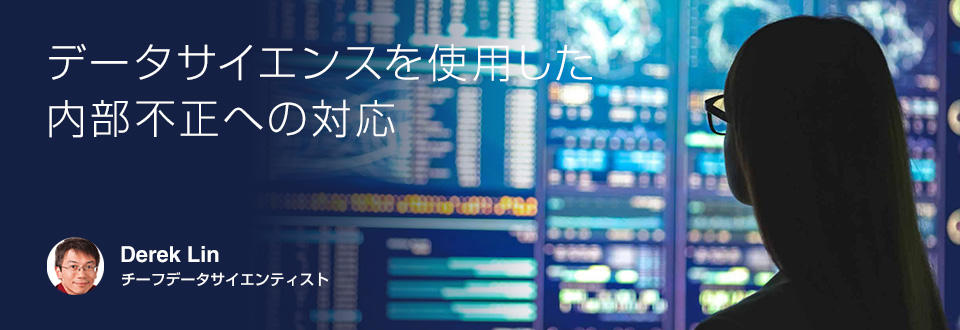
One of the key benefits of a Security Information and Event Management (SIEM) platform with UEBA (User and Entity Behavior Analytics) is that you don't have to be a data scientist to solve security use cases. The platform hides the underlying complexity of “doing data science”, allowing the SOC (Security Operations Center) to focus on defending the organization from attacks. But you might want to know what's actually going on under the hood. So, in this article, we provide an overview of how the Exabeam Security Management Platform (SMP) uses data science to address one of the most important and hard-to-find use cases: insider threat detection.
An insider is someone trusted by an organization. If such a person sabotages business or steals intellectual property or confidential data, it can have serious consequences through adverse financial, regulatory and reputational consequences. A big problem for SOCs is that insiders are allowed to use IT resources. Traditional security tools that use traditional correlation rules are poor at identifying and detecting maliciousness in seemingly authorized behavior.
The inherent limitations of static correlation rules have led IT security and management solutions to move to machine learning. This approach combines large volumes of operational and security log data with data enrichment to identify malicious activity. The security industry calls this data-centric approach UEBA (User and Entity Behavior Analytics). Let's take a look at some aspects of how data science is being used to address insider threat use cases.
Anomaly detection using statistical analysis
For insider threat use cases, Exabeam uses unsupervised learning methods to profile users' normal behavior and send alerts when there are deviations from normal. This method is used because the amount of data related to insider fraud is small, and conventional supervised machine learning requires much more data to obtain accurate results. Unsupervised learning, based on statistics and probabilistic analysis, has become the primary technical means in UEBA implementations.
Statistical analysis helps UEBA solutions perform normal state profiling of events. An example of three types of profiled data is shown in Figure 1 as histograms. High-probability events determined from profiled histograms or cluster analysis are considered benign. Deviant low-probability events are considered anomalous and correlated with security events. This is analogous to a SOC analyst manually sifting through large amounts of log data trying to make sense of it. But machines automatically do the same work on more data, faster and with greater accuracy. Based on statistical and probabilistic analysis, UEBA identifies normal behavior and determines deviations that indicate anomalous and potentially malicious behavior by insiders.
Figure 1: Histogram with different types of data
Realization of network intelligence by deriving context information
Contextual information consists of labeled attributes and properties of network users and entities. This information is critical for measuring risk of anomalous events and triaging and reviewing alerts.
Examples of context derivation use cases include service account inference, account problem solving, and personal email identification. Service accounts are used to manage assets and permissions. Because of their power, they are of high value to malicious insiders, but service accounts are not often tracked in large IT environments. Service accounts and general staff user accounts exhibit different behaviors. Data science can find unknown service accounts by analyzing text data in Active Directory (AD) or classifying accounts based on behavioral cues. As such, data science can help bring visibility into risky techniques used by malicious insiders.
The Account Resolution feature provides a security view for users with multiple accounts. Even if an individual account's activity stream appears normal to traditional security tools, aggregating the activity of multiple accounts belonging to the same user may reveal interesting anomalies. This often happens when an insider logs into a regular account and then moves to another account to perform unrelated and unusual behavior. Account resolution, powered by data science, can look at activity data and determine if two accounts really belong to one user.
Data exfiltration via personal email accounts is a common attack technique used by malicious insiders. For example, a user linked to a personal webmail account and sent an unusually large attachment. Link external email accounts to internal users based on historical behavioral data and gain visibility into data exfiltration by malicious insiders going forward.
Meta-learning for false positive control
False positives waste time and cause alert fatigue for time-stressed security analysts. Some metrics are more accurate than others, but statistically weaker metrics tend to trigger false positive alerts. Data science-powered meta-learning allows the UEBA system to automatically learn from its own behavior to improve detection performance. One method is to apply a data-driven adjustment to the initial expert-assigned scores, as shown in Figure 2. The Score Adjustment feature examines the occurrence and frequency of alerts across populations and within a user's history, respectively.
Another false positive control method intelligently suppresses some frequent alerts. By meta-learning from this history, the UEBA system automatically builds a recommender system that determines whether a user's first visit to an asset was predicted. If it was predicted, the corresponding alert is suppressed without adding a score, thus minimizing noise from false positives.
Figure 2: Scoring based on expert knowledge and data-driven adjustments
Summary
The unique challenges of insider fraud detection cannot be addressed by the traditional means of correlation rules. UEBA's statistical and probabilistic approach offers the most promising solutions for detecting such threats. However, no one-size-fits-all algorithm exists. A multi-faceted, data-centric approach is required to find insider threats with the aforementioned dimensions. Modern methods of analyzing existing data available in enterprise SIEMs using data science open up new possibilities for effective insider threat detection.
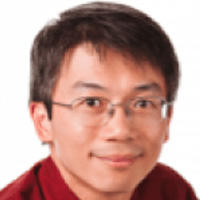
Derek Lin
Chief Data Scientist, Exabeam, Inc.
video on demand
Countermeasures against internal threats in the age of promoting remote work
~Risk visualization realized by Exabeam, a leading company in internal fraud countermeasure solutions~
働き方改革やCOVID-19の先行きが見えない中、リモートワークを推進する企業が増えております。リモートワーカーのリスク管理として内部不正対策が注目されております。このセミナーでは内部不正対策のリーディングカンパーであるExabeamを活用した事例やデモをご紹介させていただきます。
Inquiry/Document request
In charge of Macnica Exabeam Co., Ltd.
- TEL:045-476-2010
- E-mail:exabeam-sales@macnica.co.jp
Mon-Fri 8:45-17:30