Generative AI solutions to solve network operation challenges [Part 1] ~Challenges in network operation and key points for using generative AI~
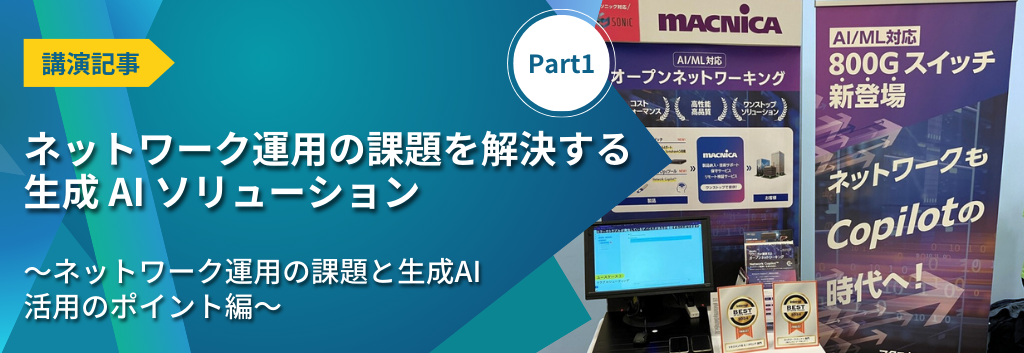
Network operations involve a variety of tasks, each of which requires advanced skills. There is also a shortage of experienced engineers and the need to pass on knowledge to new recruits.
This article is divided into Part 1 and Part 2. In Part 1, we will introduce the key points of Search Augmentation Generation (RAG), which is a method to reduce the limitations and hallucination caused by the training data of large-scale language models (LLMs) when using LLMs in your own network operations.
In Part 2, we will introduce "Network Copilot™" provided by Aviz Networks as a generative AI solution that solves network operation challenges, based on practical examples.
Please read this as well.
Network Operation Challenges
Network operation requires a variety of skills and comes with many challenges, so the following preparations are necessary before starting operations:
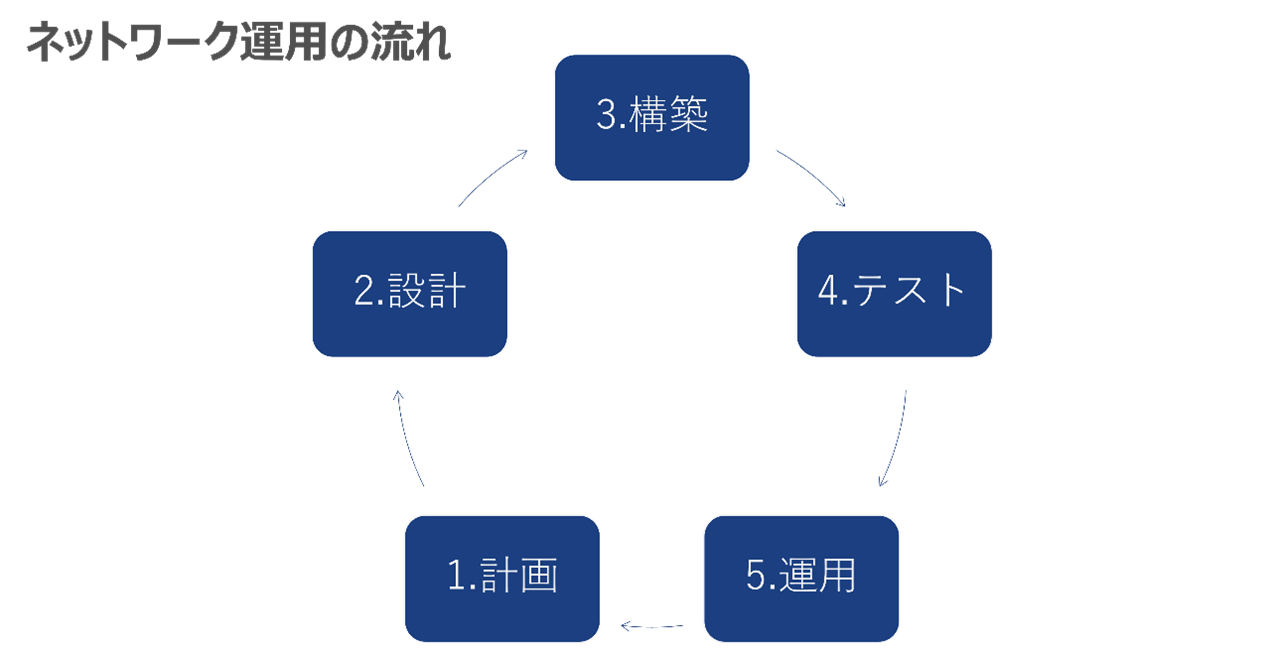
- Planning: What kind of network will you build?
- Design: How to build a network device that meets your requirements
- Build: Build a real network
- Testing: Can the service be delivered as expected?
- Operation: Operation and maintenance after network construction
Furthermore, after a few years of operation, it is common for larger bandwidth and new functions to be required, leading to the planning and design of a new network.
There is a wide variety of major devices and components that make up a network, and each one must be designed, built, and operated appropriately, so network engineers are required to have high levels of skill.
Looking at the "Trends in Internet Traffic" section of the Information and Communications White Paper, we can see that traffic has been increasing by about 20% per year in recent years.
This shows that even if a network has been operating stably for some time, an increase in traffic can cause the bandwidth of network equipment to become insufficient, which can lead to the need to build a new network.
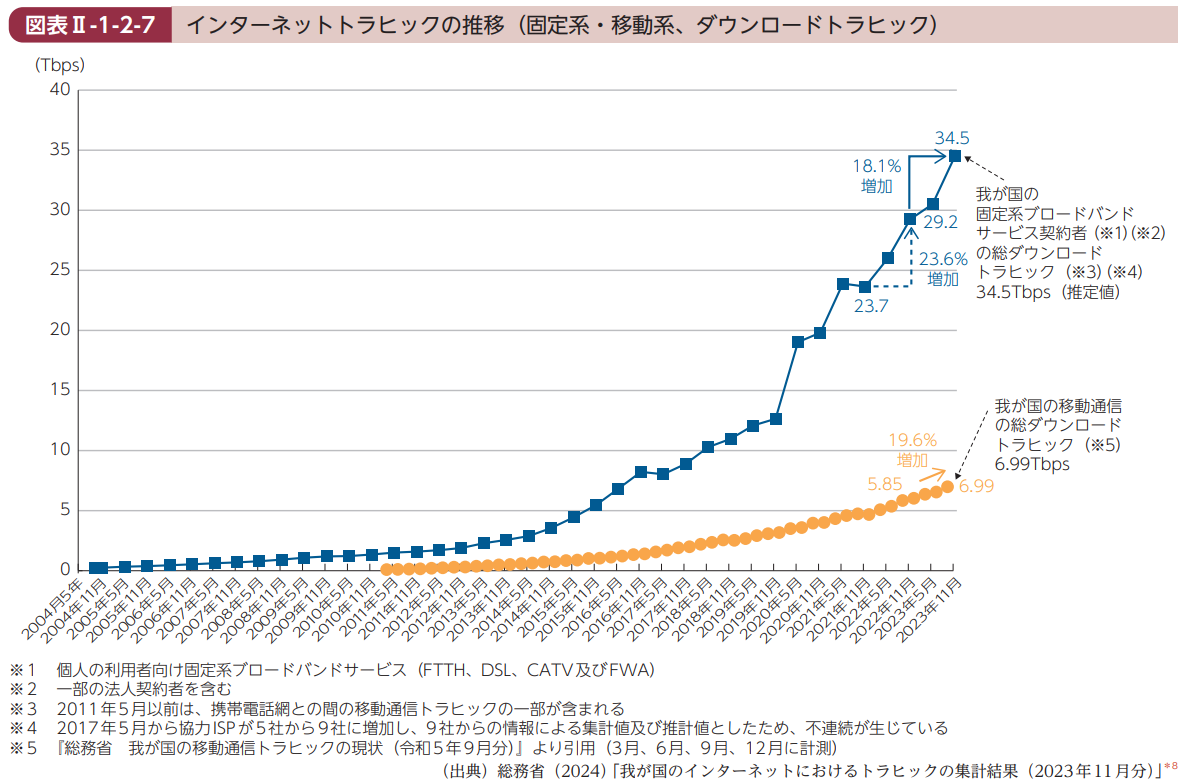
Source: "FY2024 Information and Communications White Paper" (Ministry of Internal Affairs and Communications)
https://www.soumu.go.jp/johotsusintokei/whitepaper/ja/r06/pdf/index.html
Next, if we consider network operations from the perspectives of hardware and software, as well as the technical and organizational/operational challenges, we can divide them into the following categories:
Hardware perspective |
|
Software Perspective |
|
Technical aspects |
|
Organizational and operational aspects |
|
As such, network issues have become apparent from various perspectives, creating a demand for automation and efficiency.
Key points for using generative AI (LLM)
Looking at the "Effects and impacts of utilizing generative AI" section of the Information and Communications White Paper, over 70 % of respondents agreed that it will lead to improved work efficiency and the elimination of personnel shortages, while over 60 % agreed that not utilizing generative AI will result in a loss of competitiveness as a company.
From this, we can infer that there is an urgent need to utilize generative AI, and that many people believe that if they do not start soon, they will fall behind other companies.
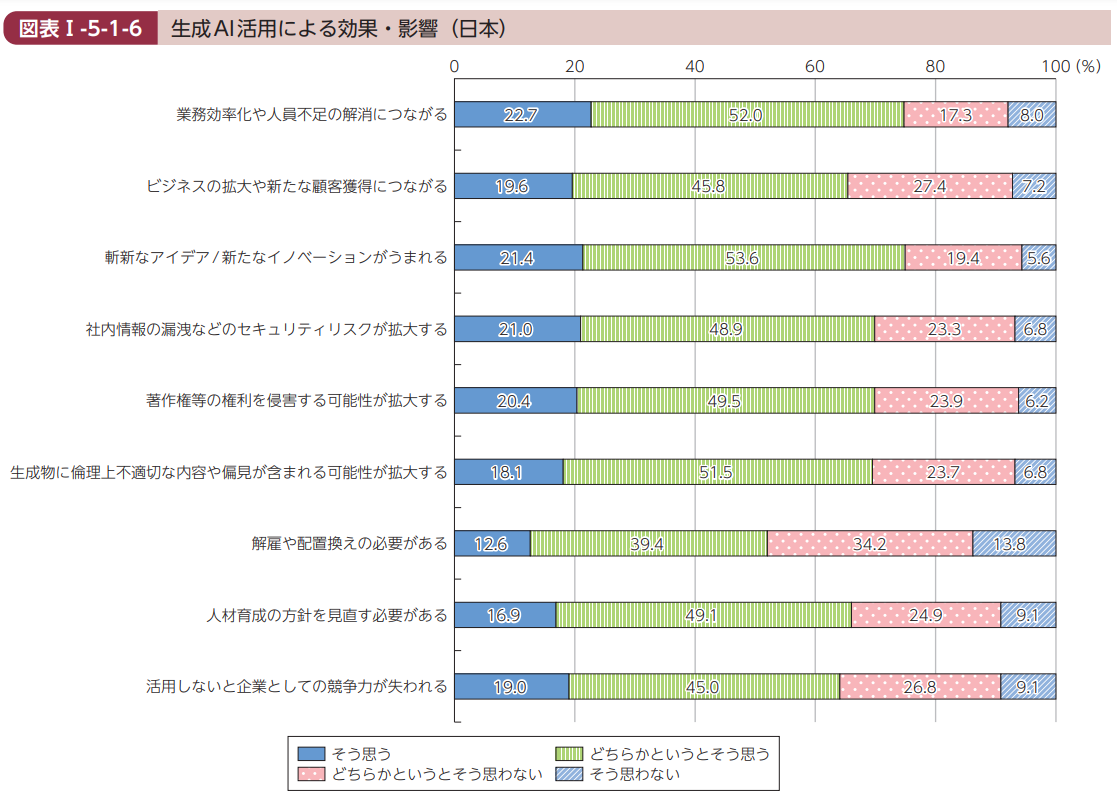
Source: "FY2024 Information and Communications White Paper" (Ministry of Internal Affairs and Communications)
https://www.soumu.go.jp/johotsusintokei/whitepaper/ja/r06/pdf/index.html
As a result, there has been a growing momentum recently to utilize generative AI, such as ChatGPT, in company operations.
Microsoft The company AI Assistant Service "Copilot" is offered for office tools, security, GitHub It is being used for various purposes, such as coding. AI is assumed to continue to evolve, and AI There also seems to be a view that companies should review their operations with the aim of utilizing this technology.
Network operation generation AI It is natural to utilize 2024 year 7 Held in Nara Prefecture in JANOG54 The program also uses the "Generation in Network Operations" AI The lecture was titled "Considerations on utilizing technology."
Well, those of you who have read the article up to this point have generated AI You may be wondering, "I understand that I need to use it, but how should I actually use it?"
So, from here we will generate AI We will introduce the key points of using it, along with an explanation of the language model.
Generate AI The company is developing a system that learns from large amounts of text data to generate natural-looking sentences and programming code. LLM There are two types of models: the large-scale language model and the diffusion model that generates images and videos. LLM We recommend that you understand the learning and reasoning process and make use of it.
LLM In the pre-training process, a large amount of training data and corpus data were mainly obtained from the Internet. GPU Let the resources learn, LLM We will create a basic model of the above.
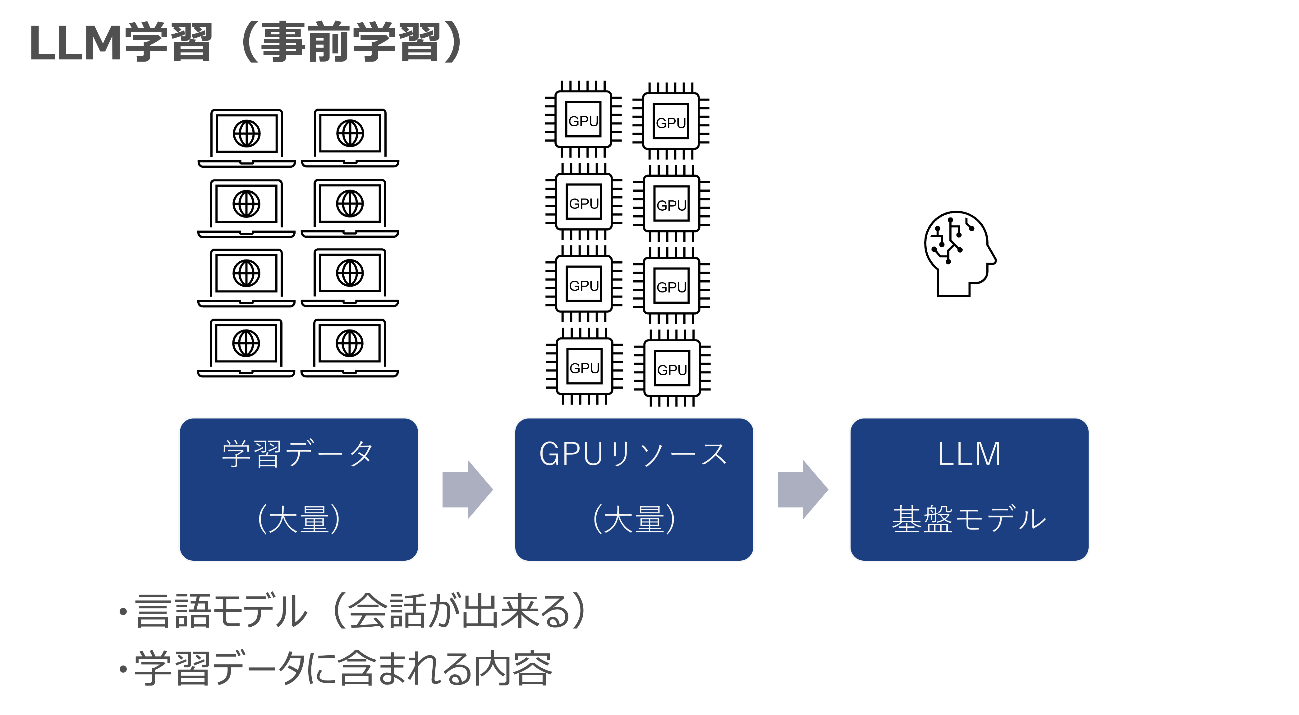
After pre-training, we use GPU resources to train data on specific tasks and domains, and tune the underlying model to create an LLM that is strong at specific tasks.
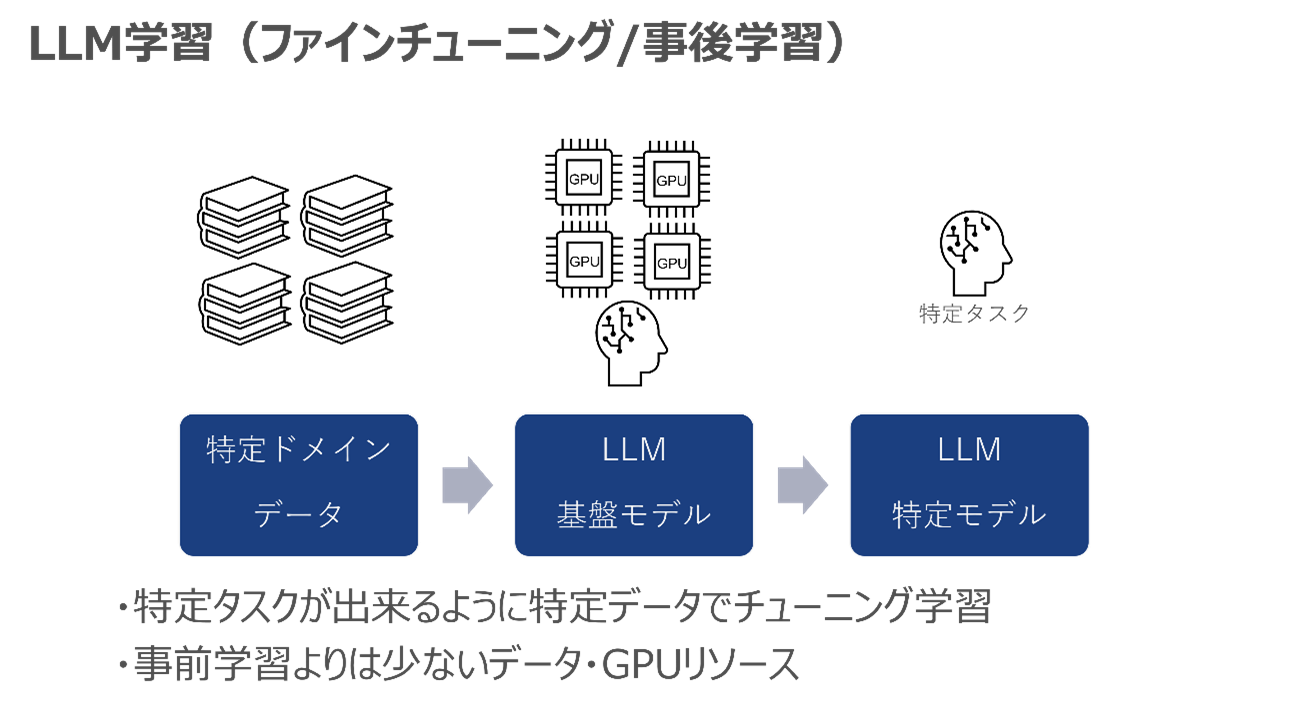
Pre-trained / fine-tuned LLMs can then be used for inference (queries). When a user asks a question, the LLM will respond based on what it has learned. Inference also requires a GPU, but there are models that can run on a CPU for small LLMs.
However, the disadvantage of the LLM is that you cannot learn what you have not studied.
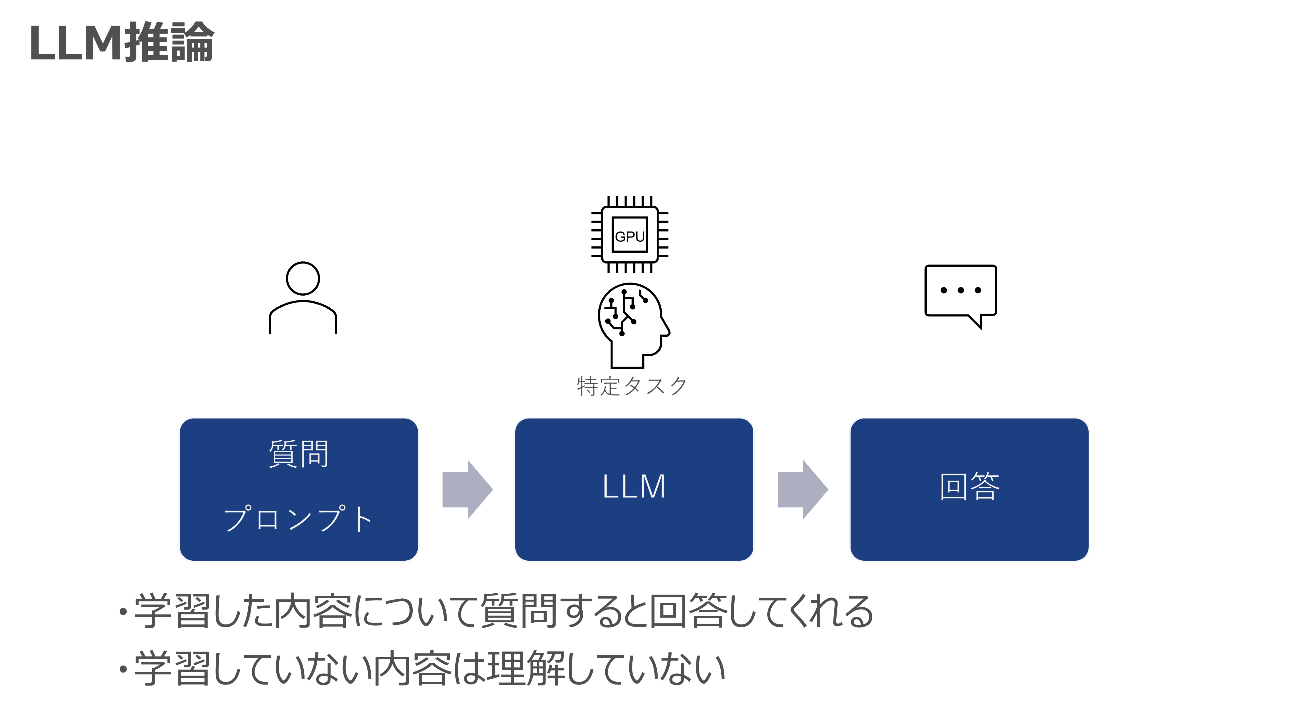
That's why a method called RAG (Retrieval Augmented Generation) has been gaining attention in recent years. With RAG, a company's knowledge and rules are stored in a database in advance, and before entering a user's question into the LLM, the database is referenced and the LLM is queried using the original question and related sentences, allowing the LLM to provide an answer based on the company's knowledge and rules.
This helps to reduce the phenomenon of "hallucination", where LLMs lie convincingly about material they have not studied.
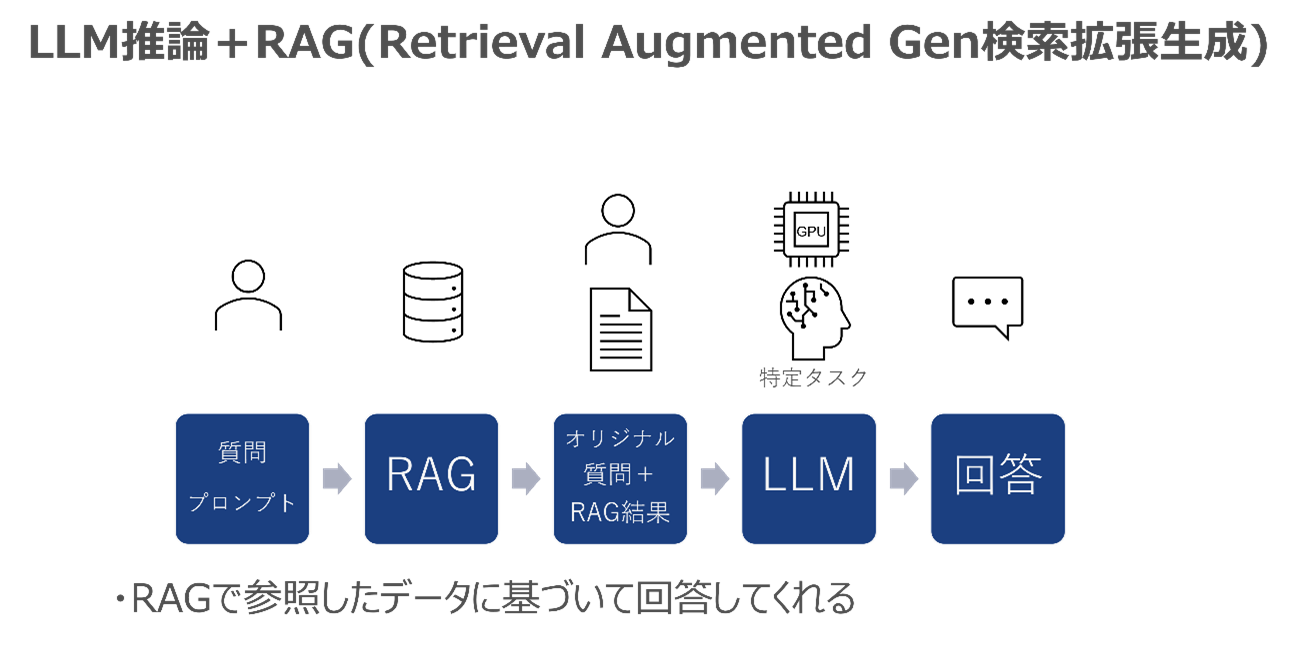
There is also a method called Tool Augmented Generation (TAG), which uses a similar concept to RAG. This is a method in which, instead of using RAG to refer to in-house knowledge and rules, an external tool is used and the results are input to the LLM, increasing the likelihood that the LLM will give the correct answer even for content that it has not learned.
For example, you can use a tool that accesses the Internet to get the latest information and then have LLM refer to it to provide you with an answer.
In addition, if a calculation is difficult to perform on the LLM, you can use a calculation tool and have the LLM provide only the results of the calculation.
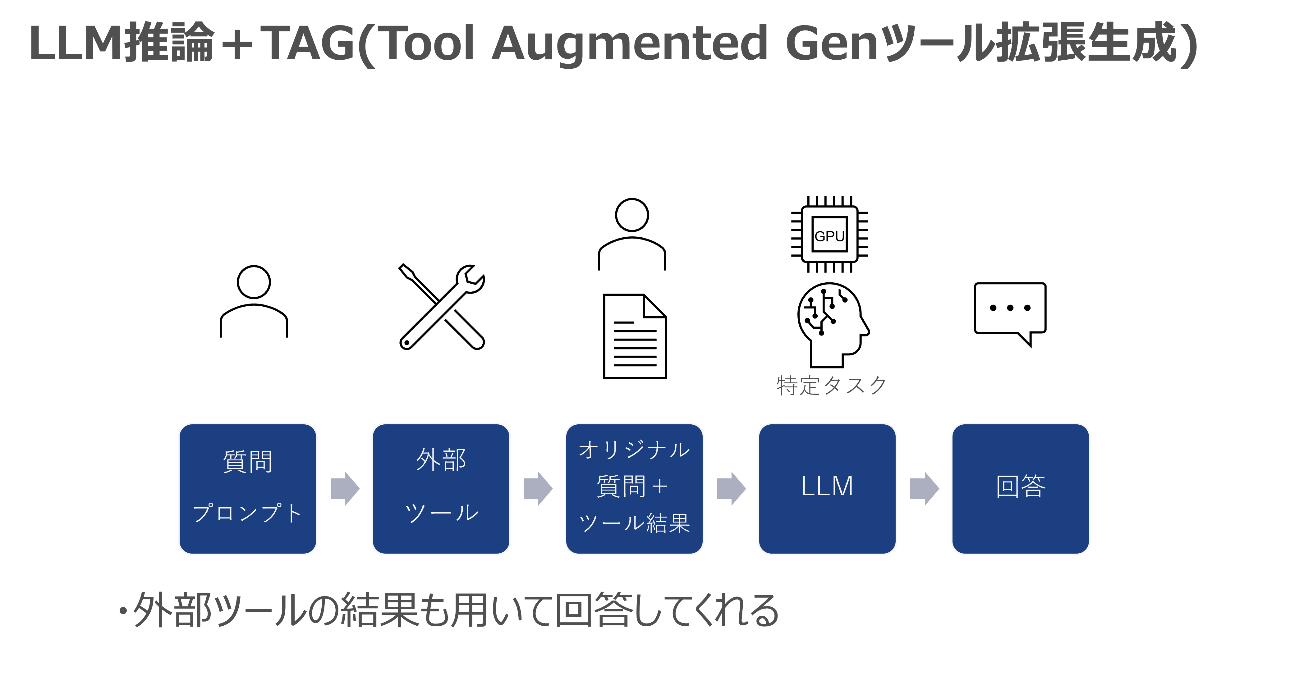
The pre-training, fine-tuning, and RAG are summarized in the table below.
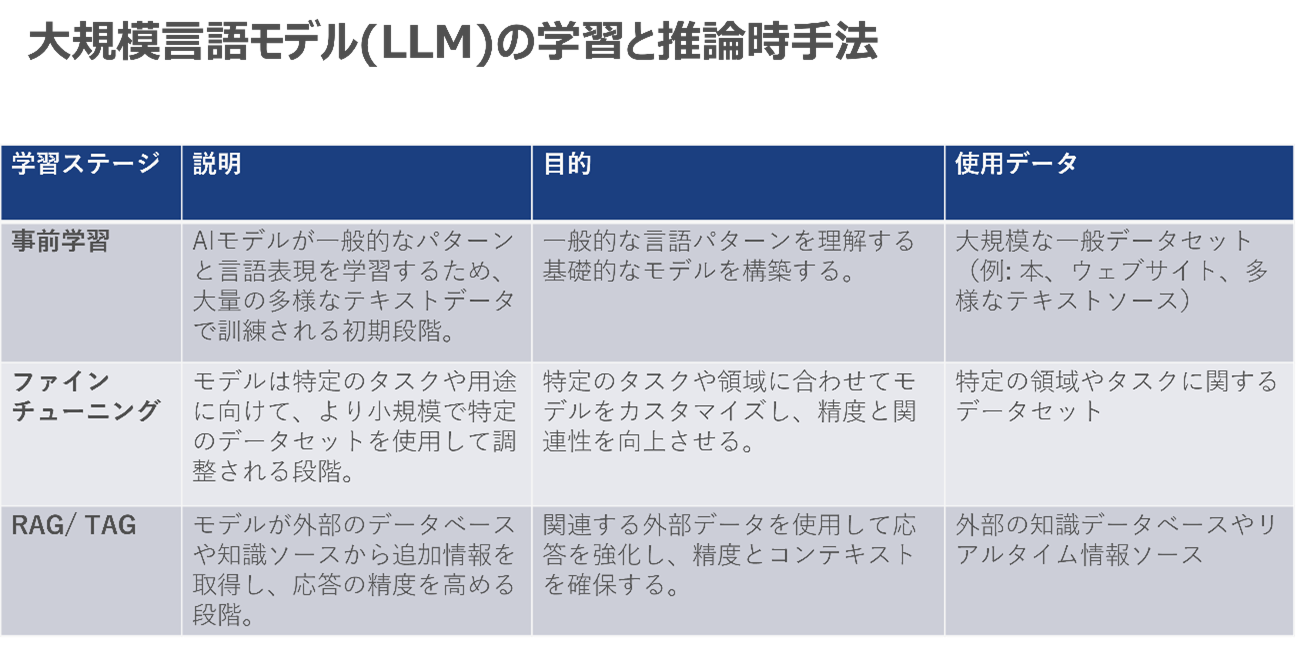
Pre-learning involves learning a large amount of public information from the Internet, etc., and post-learning involves learning for a specific task. Then, by using methods such as RAG (Search Augmentation Generation) and TAG (Tool Augmentation Generation) to answer questions based on the information referenced, hallucination can be reduced.
[Part 1] This concludes the section on learning methods using LLM and RAG.
In the next installment [Part 2], we will introduce Network Copilot™ as a generative AI solution that solves network operation challenges, using practical examples.
Related information
Click here for list of materials
In addition to introducing products handled by Macnica,
We publish materials related to open networking, such as BGP cross network automatic construction files and network operation test evaluation reports.
Click here for details
Product Page Top
Aviz Networks
We are pioneers of SONiC, an open source network operating system, providing observability, configuration automation tools and support from a team of SONiC experts.
Edgecore Networks
We continue to be a pioneer in open networking by developing and selling products related to OpenNetworking/white Box switches.
IP Infusion
As a market leader among open networking providers, we provide reliable network solutions to over 600 customers, including carriers, service providers, and data centers.