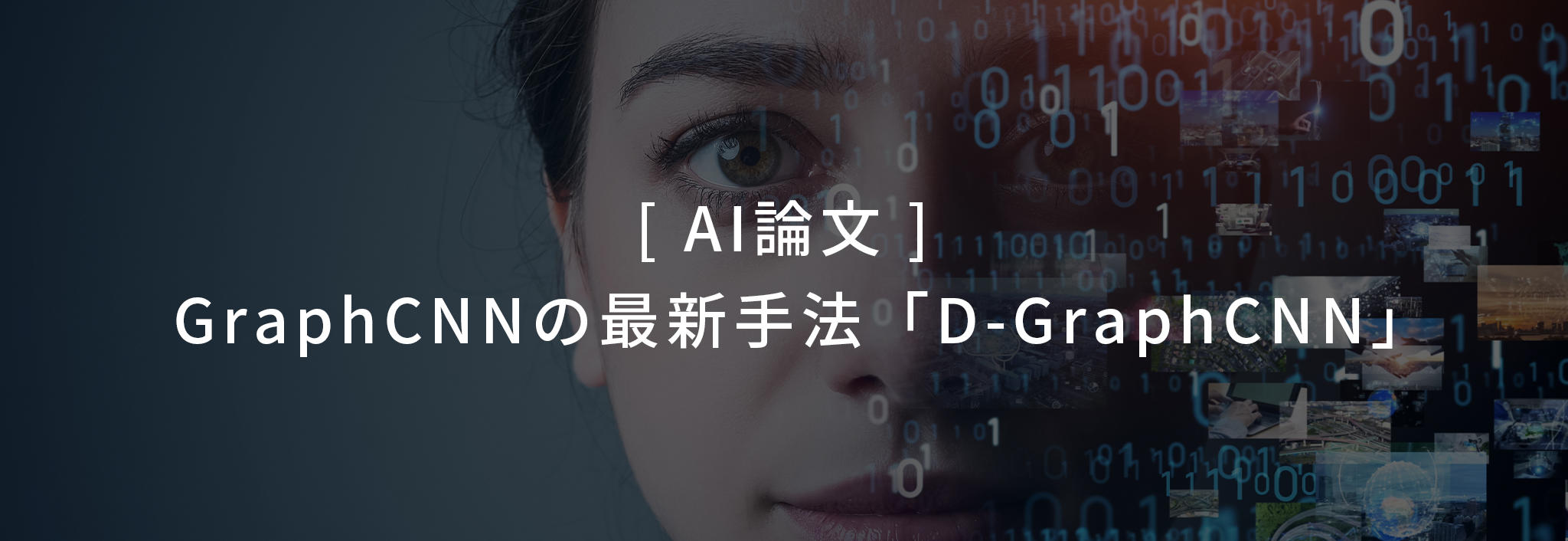
This article is recommended for those who
- I want to know the latest information about GraphCNN!
- I want to know the cutting-edge AI analysis method!
- I want to grasp the trend of the latest AI papers quickly!
Findings from this article introduction
- Learn how to apply CNN to non-image data
- 相関係数を用いたC-GraphCNNよりも有用なDistance GraphCNN(D-GraphCNN)という手法を知ることができる
Time needed to finish reading this article
10 minutes
Introducing AI papers presented at JSAI2018
Hello, I'm Makky from Macnica AI Research Center. I am also active as an AI female member!
As someone who grew up in the Tohoku region, the hot summer season has arrived. This year, my goal is to not give in to the heat and be active in everything I do.
AI女子部2回目のテックブログとなる今回は、JSAI2018で採択された論文の中から、「D-GCNN(Distance Graph Convolution Neural Network)」についてご紹介いたします。
What is JSAI (Japan Society for Artificial Intelligence)?
The AI paper introduced in this article is titled "Development of general-purpose CNN deep learning method using feature graph". This paper was presented at JSAI 2018 (Japanese Society for Artificial Intelligence 2018).
JSAI is an AI conference held in Japan, and I briefly introduced it in a previous tech blog.
Papers presented at AI conferences are a source of information on AI trends and are extremely valuable for learning about new methods. Be sure to check out our previous tech blog!
Tech Blog: Learning the cutting edge of AI from papers
In addition, two of our researchers will participate in JSAI2019 this year. We are planning to publish the state of JSAI on the tech blog, so please look forward to it too!
"GraphCNN" using CNN for non-image data
Now, let me introduce the content of the thesis! First, I would like to briefly touch on the overall picture of GraphCNN so far.
今回ご紹介する論文のテーマは「CNNの応用手法」
Do you know about CNN (Convolutional Neural Network), which is the basis of GraphCNN?
CNN is a neural network with deep layers and is often used in the image field.
To be useful with today's masses of data, we need to understand large and complex data sets. CNN is attracting attention as an effective means of doing so, which is the fundamental reason for picking up this AI paper.
CNN is difficult to adapt to non-image data
As I mentioned earlier, CNN is a method that has been widely used mainly in the image field.
This is because the order of elements in image data has meaning, and CNN analyzes the order of elements that has that meaning as a feature.
So what about non-image data like csv? For non-image data, the meaning of the data does not change even if the column order is changed. Therefore, it was not possible to adapt to data with changing graph structure.
Correlation GraphCNN(C-GraphCNN)
しかし最近、従来の考えを覆す手法が報告されました。「非画像データの順番にも意味を持たせればCNNが使える!」という発想の逆転から生まれた "GraphCNN(Graph Convolution Neural Network)" です。
As the name suggests, it is a neural network that performs a convolution operation on the graph structure. The "graph structure" mentioned here is not something like a pie chart, but a network structure like the one shown in the figure below.
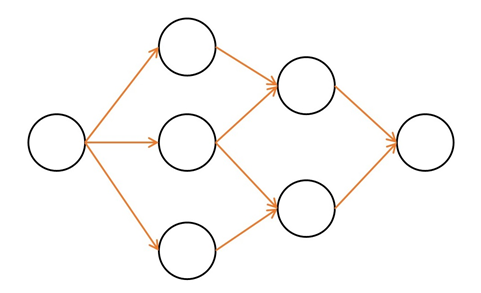
For example, the method "C-GraphCNN" is also a kind of GraphCNN.
C-GraphCNN is a technique that uses "correlation coefficient" to give meaning to the order of non-image data and adapt CNN.
Since the correlation coefficient is an index that looks at the relationship between adjacent variables, it cannot be rearranged by nature. In other words, C-GraphCNN is a method using "sequentially meaningful" correlation coefficients.
C-GraphCNN seems groundbreaking when explained in this way, but there are also issues.
The problem is that the correlation coefficient does not take into account the actual distance between the data, so it is a suboptimal way to express the relationship between the data.
D-GraphCNN with distance between data
In the paper introduced this time, based on the problems of C-GraphCNN, we propose a method "Distance GraphCNN (D-GraphCNN)" that adapts CNN with "distance matrix".
There are two types of D-GraphCNN, depending on the algorithm used to create the distance matrix.
■ Kernel Graph CNN
Uses an algorithm called Gaussian kernel to create the distance matrix
■ DBSCAN Graph CNN
Use a clustering algorithm called DBSCAN to create the distance matrix
D-GraphCNN has high analysis accuracy
In the paper, experiments are conducted using two datasets to confirm the usefulness of D-GraphCNN. In this blog, I will introduce one of the experiments as an example.
Below are the results of an experiment on a dataset called 'The Boston Housing Dataset', which deals with the regression problem of predicting Boston housing prices from 12 pieces of information about property demographics.
出典: "特徴グラフを用いた汎用型CNN深層学習手法の開発"
キャプション: 回帰問題における決定係数の推移
グラフの縦軸の決定係数は、1に近いほど分析の精度が高いことを示します。
実験結果のグラフから、確かにGraphCNN(C-GraphCNN / Kernel-GraphCNN / DBSCAN-GraphCNN)の有用性が伺えます。
特にD-GraphCNN(Kernel-GraphCNN / DBSCAN-GraphCNN)は高い精度であることがわかります。
Can GraphCNN be applied to image data/reinforcement learning?
We know that D-GraphCNN is useful for non-image data, but can it be used for image data as well?
In the paper, I also presented experimental results on whether D-GraphCNN can be applied to image data and whether it can be applied to reinforcement learning.
Applying GraphCNN to image data
In the paper, we use the MNIST dataset to challenge the image classification problem. MNIST is an image dataset containing 60,000 images of handwritten digits and 10,000 test images.
The figure below shows the results of actually using the nine-layer CNN, C-GraphCNN, and D-GraphCNN methods for learning image data. Even for image data, the accuracy rate of GraphCNN exceeds 90%, confirming its high performance.
出典: "特徴グラフを用いた汎用型CNN深層学習手法の開発"
キャプション: 分類問題のおける正解率の推移
Can also be used for reinforcement learning applications
Similarly, for reinforcement learning, we replace the neural network used for reinforcement learning with GraphCNN and check the performance.
The content of the experiment is to see the game score transition called CartPole in a simulator environment using CartPole of Open AI gym.
The figure below shows the transition of the score, but the left side is the result of the neural network and the right side is the result of C-GraphCNN.
出典: "特徴グラフを用いた汎用型CNN深層学習手法の開発"
キャプション: カートポールにおけるスコアの推移
グラフからC-GraphCNNは最高点に到達するのが早く、かつ最高点を維持する回数が多いことが分かります。このことから、正解率のスコア差が激しいという課題はありますが、GraphCNNは有効と考えられます。
Summary
今回は、非画像データにCNNを応用する新しい手法「D-GraphCNN」が提唱された論文をご紹介しました。
テックブログではこれからもさまざまな分野における、最新のAI論文について取り上げていきます!
近々日本以外の最新論文もご紹介する予定なので、今後もAI女子部の活動をお楽しみに!
もし「AIについて相談してみたい」と思うことがあれば、マクニカのAI専門家組織、AI Research & Innovation Hubまでお気軽にお問合せください!
■ 本ページで掲載した論文の出典元 |