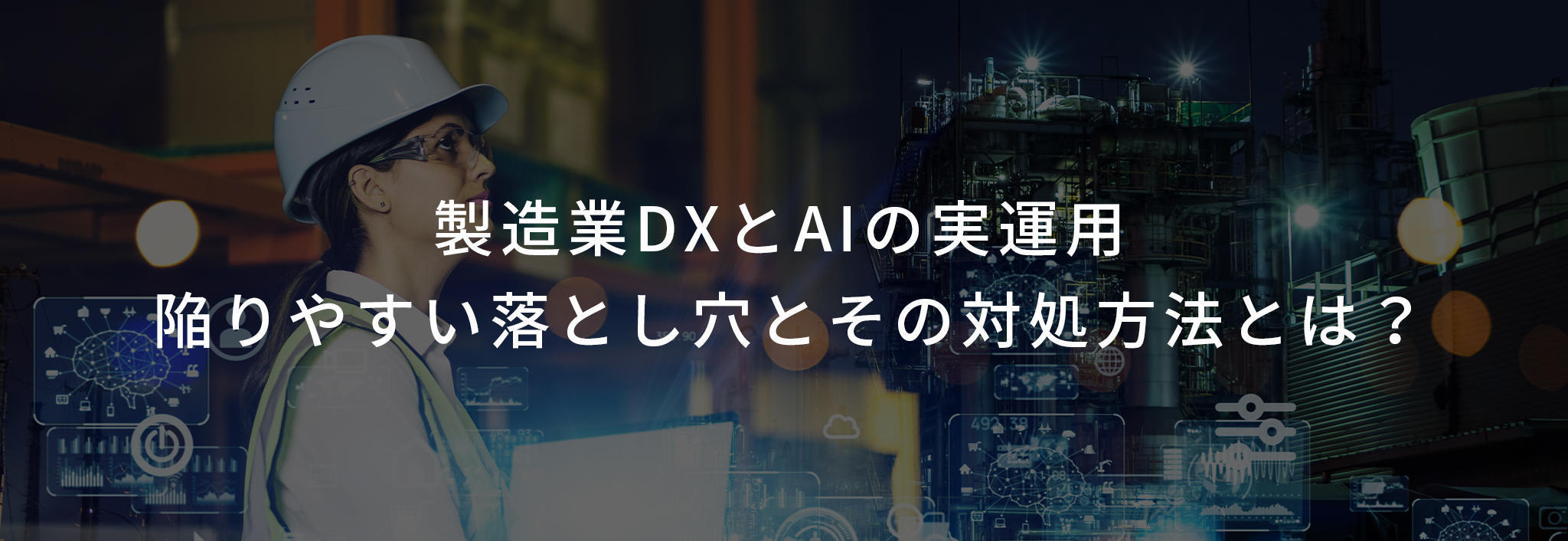
What is the core data collection point in digital transformation (DX)?
And what are the effective ways to use the collected data?
Macnica provides assistance and support to numerous DX projects.
Based on the experience cultivated there, here we will explain ``key points to increase the success rate of DX projects'' and points to note in ``data utilization'' that is the basis of various projects, and how to deal with it. I would like to introduce you to
Speaker information
Macnica
Innovation Strategy Business Headquarters AI Business Promotion Department
Digital Offering Department
Yoshitaka Haga
What are the barriers that hinder DX promotion projects in the manufacturing industry?
働き方改革の推進や新型コロナウイルスへの対応などを背景に、企業のDXが大きく加速しています。
その流れは製造業においても同様であり、データやAIを活用した業務改革が多くの企業で検討されています。
“I feel that the way of thinking about AI in the manufacturing industry has changed dramatically over the past few years.As a result, I think the scope of the roles required of DX personnel is expanding,” says Macnica. Haga speaks.
If you are assigned to utilize AI and promote DX, naturally results will be required.
Among many projects, we are forced to make decisions based on cost, effectiveness, balance, etc. In addition, when the project was launched in earnest, tasks that were not emphasized before, such as inter-departmental cooperation and organizing the work flow, began to pile up. And there should be very few things that the DX promotion department can solve alone.
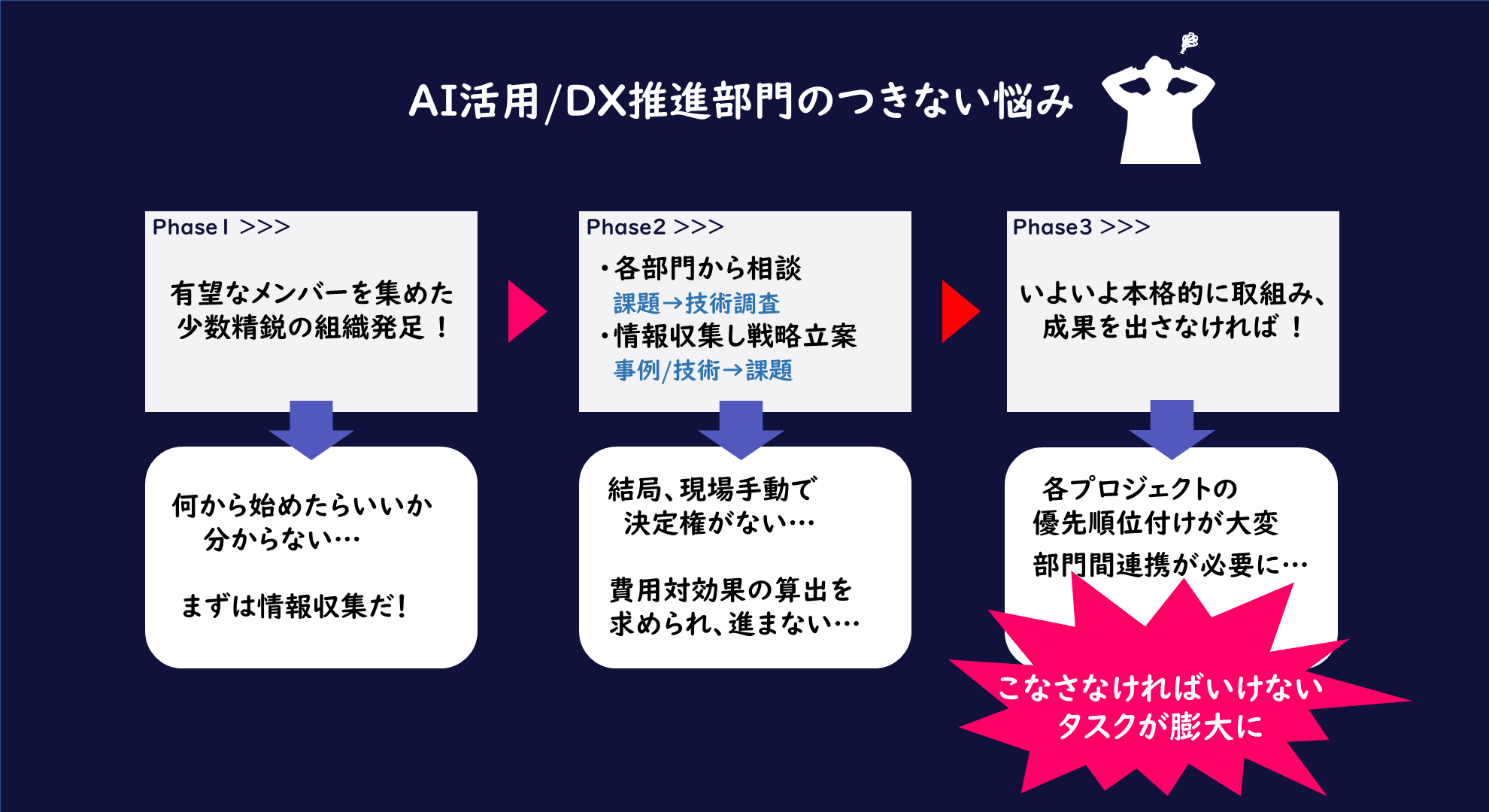
The role required of the DX promotion department continues to increase
In recent years, many DX projects have been started with an eye on actual operation. What are the obstacles and how can we overcome them?
“One of the most stumbling points in a DX project is the lack of a clear vision or roadmap. No. Among them, there are many projects where the division of roles between internal and external parties is not clear, and collaboration is not smooth.There are also human resources with the knowledge and skills necessary to promote DX. In some cases, the project does not get off the ground because of the shortage.”
“In order to overcome the barriers that hinder DX, it is necessary to formulate an overall plan that seeks cooperation and integration between individual projects and departments.
As a solution, we can think of three patterns: training human resources in-house, utilizing external partners, or doing both. It is quite rare to get through with only internal human resources, and in many cases we utilize the knowledge of partners who have specialized knowledge. However, since we first need human resources who can speak at the same level as our partners, most companies work on human resource development in parallel.” (Haga)
Five success points common to DX advanced companies
While there are many companies that are stuck in promoting DX, there are also companies that have progressed to the actual operation of AI and have succeeded. Haga says that there are five points that successful companies have in common.
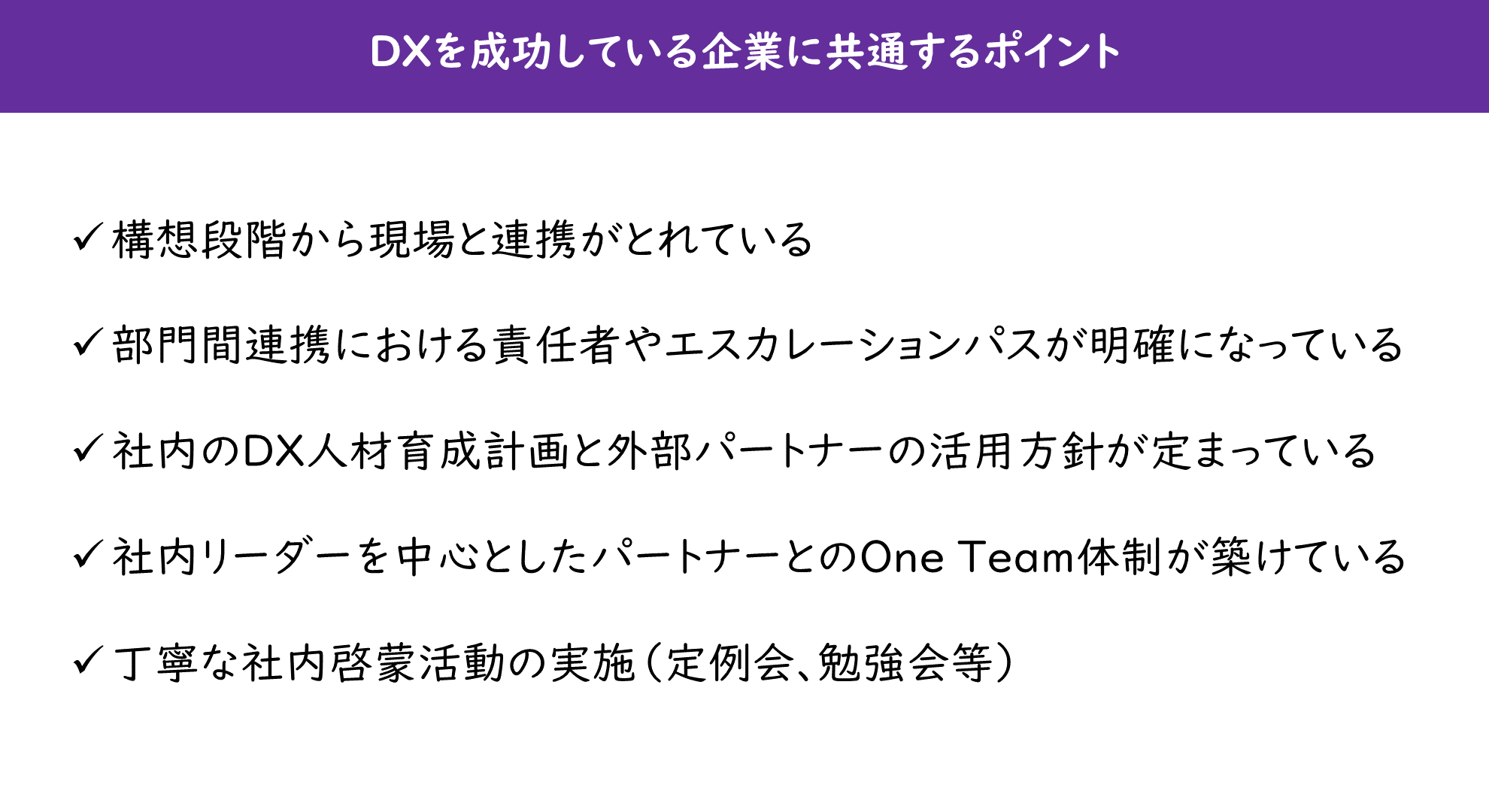
Incorporating common points of successful companies is a shortcut to promoting DX
Regarding the point "The person in charge and the escalation path for inter-departmental collaboration are clear", "This is especially noticeable in situations where people at the same level are together. For example, the manager of the DX promotion department , or the general manager of the manufacturing department are involved in the same project, unless it is clear who has the decision-making authority and responsibility, there will be disputes and ambiguity,” explains Haga.
How to handle difficult data unique to the manufacturing industry
Even though everyone recognizes that data is the most important factor in the actual operation of AI, the DX project may come to a standstill. Most of the reasons are said to be due to the lack of reliable data.
“In order for the manufacturing industry to effectively utilize data and develop and grow in the future, data collection has become an unavoidable issue. Because the quality and structure of the data are completely different, it is necessary to devise data acquisition methods and processing for each, which is very “steady” and difficult.” (Haga)
The OT side is not a world where all you need is data, and the logic that comes from big data analysis is almost useless. Unstructured and complex data and fine-grained data are not easy to use just because they are collected.
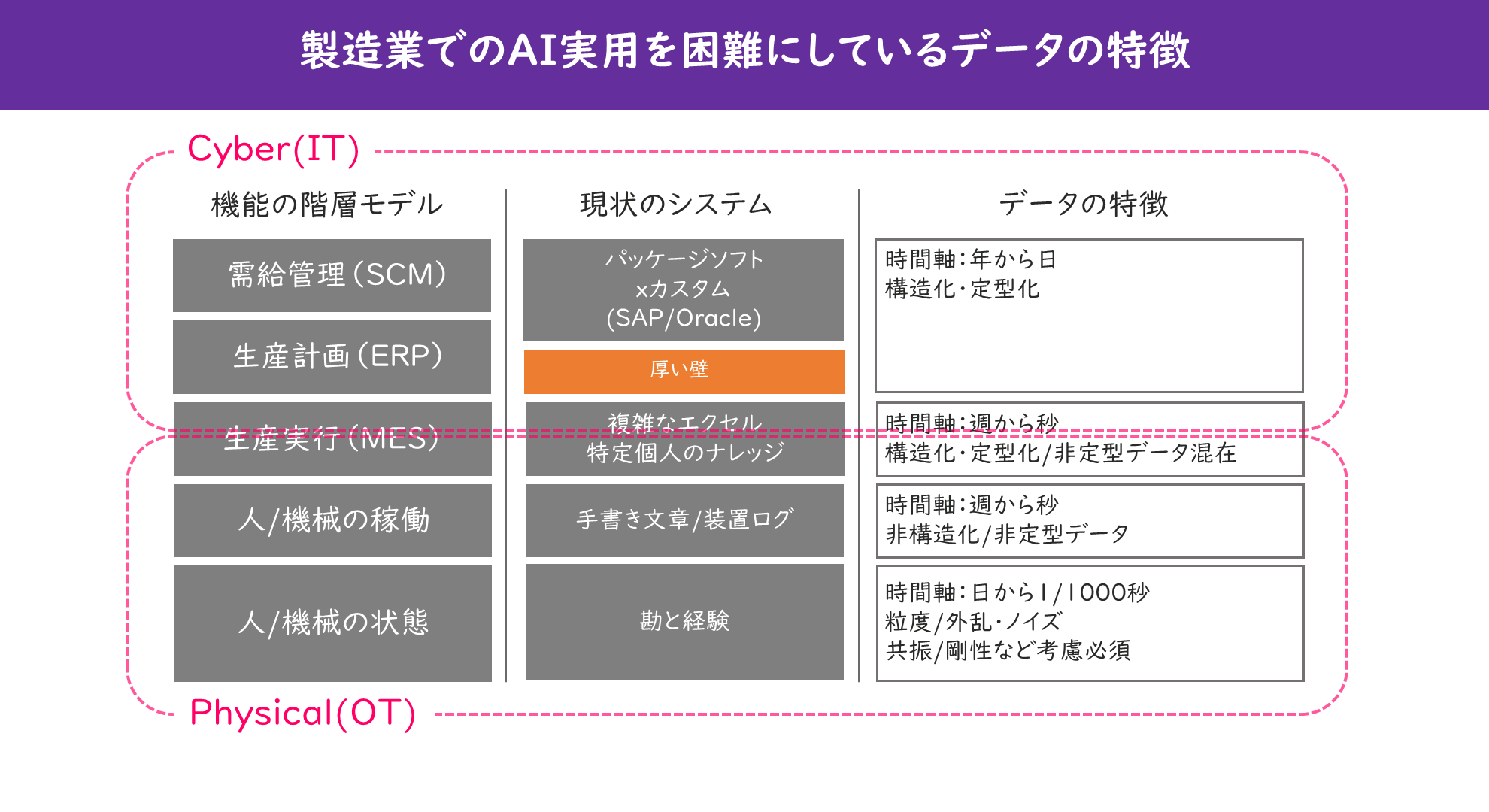
The manufacturing industry is characterized by a high degree of difficulty in handling data compared to other fields.
Haga goes on to say that there are three pitfalls in data collection. If these aren't enough, you'll get stuck in the data validation phase.
The first is "insight and examination of sensing."
“It is common to hear that sensors were installed to collect data, but the data was not at a level that could be analyzed. There is no problem if it is based on agile course correction, but the longer you notice it, the greater the impact it will have on the project. (Haga)
The second is "assumed difference for each equipment".
“This means that it is more difficult than expected to collect equipment operation data. You may think that it is only natural to obtain the status of existing equipment, such as operation information, especially for management who are far from the site. People tend to think that data collection is not difficult, because there is a fundamental requirement that they do not want to spend money or time at the "seeing" stage, but in reality there are many cases where problems arise. (Haga)
And the third is “examination of the data collection mechanism”.
“It is important to firmly define the storage format of the data that will be output as a data mart. , It is important to create a system with an image of the number of digits in the lot number, manufacturing model number, etc. Since the resolution of the data in the process is often different, this definition requires experience and sense. (Haga)
Points to keep in mind in AI system management
AI systems require ongoing management unlike other IT systems. Haga talks about AI production system management as follows.
“Generally speaking, IT systems are designed and developed according to the requirements definition and guaranteed according to the requirements definition. However, it is difficult to guarantee the accuracy of AI.Therefore, it is necessary to monitor AI accuracy and data drift to see if AI is performing as expected.” (Haga)
Factory data is particularly complex, and data trends can change due to various factors. Due to the unexpected changes, the answers that AI derives will also change, so by the time you realize that something is completely wrong, it will be too late. For that reason, it is necessary to track changes firmly.
In addition, in order to respond to changes, it is necessary to have a mechanism to manage the version of the AI model and apply the optimal model. Without this mechanism, no matter how good the judgment was when it was created, the system would only become less accurate over time.
In addition, Haga identified the three major themes of manufacturing DX: “sophistication and labor saving of production planning,” “quality improvement,” and “standardization of individualized processes.”
Regarding "sophistication and labor saving of production planning", there is a need for sophistication to respond to sudden changes in demand and high-mix low-volume production, and needs for DX and AI utilization for the purpose of reducing and standardizing man-hours involved in planning operations. is rising.
“When creating an optimal production plan, “what” data is looked at and “how” decisions are made differ from person to person. It is necessary to visualize business processes and create decision rules.Understanding the situation at the site is more important than AI model development and accuracy improvement." (Haga)
Current situation analysis is an important process, but the resolution tends to be rough, and it may not be used as a basis for introducing new systems and mechanisms.
The reason for this is that the burden on those involved in the survey is heavy, or that the credibility of the survey results is uncertain. Efficiency analysis using a simulator is also effective for analyzing the current situation and redesigning business processes, so it is better to proceed with consideration before introducing AI.
It is best to consider both "quality improvement" and "standardization of individualized processes" at the same time, rather than separately. Since quality control is the source of competitiveness, many companies will be devoting a large amount of human resources to it. However, there are many cases where quality control depends on the know-how of experts, so more and more companies are feeling the risk of continuous maintenance and development.
“In terms of quality improvement, there are themes such as the cause of defects, quality prediction, and optimal control. Appropriate control is performed by deriving parameters, etc. Ultimately, some companies are considering automatic operation that feeds back the optimal settings to the machine, but the current system is based on the premise of coexistence with humans. is the mainstream.” (Haga)
Finally, "I imagine that the missions and projects you are working on are not all easy. I would like you to reduce the problems that you are likely to fall into and refer to the success pattern to increase the probability of success even a little. I think," he concluded.